All published articles of this journal are available on ScienceDirect.
Investigating Functional Connectivity in Adolescent Depression and Suicide Attempt during Neurofeedback Sessions: A Multivariate Random Covariance Model Approach
Abstract
Background
A recent neurofeedback functional magnetic resonance imaging (NF, fMRI) study on depressed vs. healthy adolescents elicited differential functional connectivity (FC) amongst brain regions of interest (ROIs). Previous results employed univariate methods and included only two seed areas of FC (amygdala and hippocampus). In this study, we propose a new multivariate analysis for whole-network FC estimation.
Methods
Primary analyses concerned a pre-identified network of 17 salient ROIs reflecting key regions in self-processing and emotion regulation. A random covariance model (RCM) was applied to jointly estimate participant- and group-specific connectivity, where FC was measured by partial correlation conditioned on or adjusted for rest-of-network connectivity patterns. Secondary analyses concerned participant-specific network association with mental functioning changes and the AAL3 whole-brain atlas.
Results
New findings suggested that depressed adolescents with a suicide attempt expressed significantly higher positive FC between the left temporal gyrus and the left amygdala during NF, compared to negative FC in non-attempting depressed youth, while healthy controls displayed negative FC between the insula, inferior frontal gyrus to inferior parietal lobe connection, compared to mild negative connectivity in depressed adolescents. Previous cross-hemispheric findings in depressed vs. healthy adolescents were corroborated.
Conclusion
A multivariate RCM uncovered key ROI-pairwise connections differentiating FC patterns between depressed youth vs. healthy controls and among depressed youth, with and without a suicide attempt. Findings were strengthened by enhanced inference vs. univariate methods, and corroboration of previous NF secondary analyses demonstrated future utility for participant-specific study in association with clinical outcomes and/or whole-brain analyses with larger sample sizes.
1. INTRODUCTION
Major Depressive Disorder (MDD) is highly prevalent in the US. Estimates indicate that 1 in every 5 US adults will have MDD throughout their lifetime, and it is highly associated with comorbidity and adverse health outcomes [1]. Adolescence is a period of heightened risk for depression, which, in turn, is associated with higher symptom severity, suicide attempts, and its completion [2, 3]. Depression that persists or recurs is also a primary risk factor for adolescent suicide attempts [3]. Current treatments, i.e., medication and psychotherapy, are modestly effective at improving recovery from depressive episodes but do not necessarily prevent recurrence [4, 5], and the effectiveness of extant treatments for suicide symptoms is limited [6-12]. Given that suicide is the second leading cause of death amongst US adolescents [13] and a leading cause of death amongst 10-19-year-old youth worldwide [14], novel treatments targeting depression are vital to mitigate suicide risk. Quevedo et al. [15] proposed a novel neurofeedback (NF) procedure aimed at inducing neuroplasticity to improve self-processing in depressed adolescents, which elicited significant short-term symptom reduction in depressed youth [16]. The aim of the NF procedure was to lay down the preliminary work for more extensive clinical NF trials aimed at depression and suicide risks, as well as understanding the neural mechanisms of effective NF in depressed adolescents. As expected, some of the depressed youth (N = 15) had attempted suicide and were examined anew in this research.
1.1. Emotional Self-Other Morph Neurofeedback (ESOM-NF) Task
The Emotional Self-Other Morph Neurofeedback (ESOM-NF) task reported by Quevedo et al. [15] targeted the neural substrates of visual self-processing and emotional regulation in depressed adolescents. Negative self-processing is a core feature of depression that is often intractable and resistant to change even after clinical depression symptoms elapse [17]. Abnormal and negative self-processing is also a risk factor for persistent depression and suicide attempts [18]. Quevedo et al. [15] suggested the NF protocol of increasing bilateral amygdala and hippocampus activity for facial self-recognition. Facial self-recognition is enabled by the fusiform cortex and limbic structures and also recruits midline cortical structures and affective memory via the amygdala and hippocampus complex [19, 20]. The self-face was thought to be an ideal stimulus to pair with NF training targeting the amygdala and hippocampus complex. During the ESOM-NF procedure, participants interacted with real-time fMRI visualizations of their brains’ amygdala and hippocampus activity (displayed as a colored bar of varying height) and were asked to modulate visualized brain function during the task by recalling happy memories. The goal was to enhance positive self-processing and engage its neural networks, as well as to endow depressed youth with self-regulatory skills. Initial results revealed that, during the task, depressed adolescents showed higher fusiform, inferior parietal lobule, and cuneus activity in whole brain group comparisons, while healthy controls showed higher amygdala and hippocampus levels on average in region of interest (ROI) analysis compared to depressed youth [15]. Further analysis likewise demonstrated differential peak amygdala connectivity with key frontocortical ROIs by hemisphere in depressed youth versus controls [16]. However, the number of ROIs explored in those past publications was small (only amygdala and hippocampus), and the neural networks of emotion regulation and self-processing entail multiple brain regions that were not explored as direct contributors, including the insula, various sub-regions of the anterior or posterior cingulate cortex and the vast cortical regions of the superior, middle and inferior temporal gyri, which are engaged during social cognition. In addition to limitations due to unexplored neural mechanisms, prior connectivity analyses were partially limited by the methodological assumptions of psychophysiological interaction (PPI) analyses. Critically, Quevedo et al. [15, 16] did not test whether there were unique networks associated with adolescent suicide risks or participant-specific changes in pre-post neurofeedback during self vs. other face recognition tasks after versus before NF training.
1.2. The Neural Substrates of Self-processing and Emotion Regulation
Emotion regulation entails psychological functions supported by a network comprised of cortical and limbic regions, such as the amygdala, insula, and anterior cingulate cortex [21] and within the medial prefrontal cortex (MPFC) at large. Emotion regulation entails modulating the intensity, duration, and valence of emotional experiences. The neural substrates of emotion regulation overlap with the saliency and self-processing network. They include the amygdala-hippocampal complex (AMYHIPP), which is engaged by emotionally salient stimuli [22]. Interactions between the self-processing, dorsal attention, frontoparietal, and salience networks also enable positive and negative emotion processing and regulation [23]. The anterior cingulate cortex (ACC), a key emotion regulation node, supports conflict monitoring [24], error detection, and emotion-guided decision-making [25, 26]. The ACC, in coordination with the insula [27], contributes to interoception, sensorimotor and socio-emotional processing, as well as attention and salience processing [28]. Additionally, the MPFC enables emotion regulation [29], decision-making [30], and self-processing [31]. MPFC functional abnormalities are present in almost every psychiatric disorder, with impaired processing of facial expressions and self-relevant information [32]. Converging evidence indicates that the MPFC node may function as a central hub in the brain for information synthesis and coordination [33]. Given the complexity and multiplicity of interactions between neural nodes undergirding both emotion regulation and self-processing, we sought first to expand past research on connectivity differences during neurofeedback between control and depressed youth [16] by studying a larger network of neural nodes. Second, the present analysis extended prior work to explore potential neurobiological markers within depressed youth that might differentiate those who had attempted suicide, or had suicide ideation, versus those who had not. Lastly, a participant-specific model was leveraged to investigate potential neurobiological markers associated with changes in mental functioning pre-post neurofeedback for future precision intervention.
1.3. The Current Study
Methodologically, differential amygdala connectivity results between depressed and healthy adolescents in past research were obtained with a two-level modeling approach and univariate significance testing [16]. A 1st level psychophysiological interactions (PPI) analysis was employed, well characterized by O’Reilly et al. [34], comprised of a voxel-wise generalized linear model (GLM) to explain activity changes in 8mm seed ROIs via the interaction between the amygdala and task conditions. After obtaining the 1st level PPI estimates, amygdala time course(s) for each task condition and participant, a 2nd level GLM model was employed with diagnostic group and hemisphere as covariates. Finally, salient regions identified in level 2 were included in post-hoc, univariate ROI pairwise t-tests to infer FC differences between healthy and depressed adolescents.
While the multi-level procedure was useful for identifying salient ROIs functionally connected to the amygdala, as a univariate approach, it cannot assess FC across an entire network of ROIs and only affords group-wise comparisons, unadjusted for participant-specific connectivity or variability within groups. To improve and extend previous analyses, the random covariance model (RCM) developed by Zhang et al. [35] was proposed to re-examine network connectivity during the ESOM-NF task. The RCM takes a bi-level, Gaussian graphical modeling approach to network analysis of multiple ROIs from multi-participant fMRI studies. Graphical models serve as a useful tool for representing the conditional relationships amongst a set of random variables; in our case, it was the mean-extracted time series of the blood oxygen level-dependent (BOLD) signals from a collection of ROIs in the brain.
The general motivation behind the RCM is that we assume there is an overall group-level network of ROIs where some regions are functionally connected while others are not. We then hypothesize that since individual brain function can vary greatly from person to person, each participant in the study has their own individual-level network, which likewise varies randomly around the overall group-level network. Using graphs and precision (inverse covariance) matrices, we then jointly modeled each participants’ network as a graph with edges if two ROIs were connected and edge weights from the precision matrix, as well as the overall group network. The RCM then employed a penalized likelihood-based approach, enabling multivariate estimation of FC over networks with many ROIs and simultaneous estimation of both group- and participant-specific functional networks. It is critical to note that the RCM estimates functional connectivity jointly through partial correlation (from the precision matrix) as opposed to marginal univariate correlation. Partial correlation may be interpreted as estimating the FC between two ROIs conditioned on or adjusted for the FC between other ROIs in the rest of the network. Without adjusting for the rest of the network, marginal univariate correlation can be greatly affected by intermediary regions altering the association or simply just noise in the network. Thus, the RCM provides enhanced FC estimation and inference through partial correlation, though it should be noted that effect sizes may differ significantly from previous estimates using marginal correlation. In the biological context, the additional complexity of this new method allows for a broader coverage of emotion regulation and self-processing networks, novel inference on differential connectivity within and between diagnostic groups, more flexibility to assess and leverage participant-specific FC within diagnostic groups, as well as the novel exploration and visualization of specifically targeted networks or whole-brain atlases.
We applied the RCM to the ESOM-NF task-based fMRI data with the following primary goals: (1) uncover key FC differences in depressed adolescents between those with or without suicide attempt(s) or ideation; (2) validate past cross-hemispheric amygdalae FC differences between depressed and healthy adolescents presented by Quevedo et al. [16]; (3) investigate potential precision neuro- biological markers for targeted intervention via the correlation between participant-specific FC and pre-post neurofeedback changes in rumination or mood and feelings questionnaire (MFQ) scoring; and (4) explore whole-brain differences across the ESOM-NF task between depressed and healthy youth via the Automated Anatomical Labeling Atlas 3 (AAL3) detailed by Rolls et al. [36].
2. METHODS
2.1. Data and ESOM-NF Task
These fMRI data were obtained from the neurofeedback (NF) and neuroplasticity study of visual self-processing in adolescent depression [15]. Right-handed adolescents (N = 53) were recruited from the University of Minnesota community and inpatient clinics for study, after exclusion for substance abuse, major medical or psychiatric disorders (though experimentation was allowed), major medical or psychiatric disorders, and standard MRI exclusions. All adolescents were evaluated using the standard Kiddie Schedule for Affective Disorders and Schizophrenia for School-age Children - Present and Lifetime (K-SADS-PL) and Children's Depression Rating Scale (CDRS) inventories, with IQ measured by the Wechsler Abbreviated Scale of Intelligence (WASI). Depressed participants were stable on medication. During the second session, the Mood and Feelings Questionnaire, MFQ [37], and the Responses to Depression, RD [38], were used to measure depression and rumination symptoms before and after scanning. Participants (ND = 34) were diagnosed by a licensed clinical psychologist (KQ) as presenting depression or not having depression or any psychopathology and were deemed to be healthy controls (NC = 19). Within the depressed group, NS = 15 adolescents had a suicide attempt and NI = 29 reported suicide ideation. Demographic characteristics were found to be comparable between depressed and healthy controls at baseline reported by Quevedo et al. [16] (Table 1), and within the depressed group, all were on stable medication, with 26 taking antidepressants, 10 taking anxiolytics, and 2 taking antipsychotics.
Region of Interest (ROI) | MNI | Coordinates | - | |
---|---|---|---|---|
- | X | Y | Z | Hemi. |
(R) Hippocampus | 34 | -34 | -10 | Right |
Anterior cingulate cortex, superior and middle frontal gyrus (BA 6, 8, 9, 24, 32) | -36 | -26 | -14 | Left |
(L) Superior, middle, inferior temporal gyrus (BA 19, 22, 37, 39) | -44 | -78 | 12 | Left |
Insula, inferior frontal gyrus, putamen (BA 13, 45, 47) | 30 | 20 | -10 | Right |
(R) Superior, middle, inferior temporal gyrus (BA 19, 22, 37, 39) | 5 | -62 | -2 | Right |
Insula, inferior frontal gyrus (BA 13, 47) | -28 | 22 | -10 | Left |
Cerebellum | -8 | -72 | -40 | Left |
Precentral gyrus, middle and inferior frontal gyrus (BA 6, 9) | 40 | -2 | 50 | Right |
Anterior cingulate cortex, superior and middle frontal gyrus (BA 6, 8, 9, 24, 32) | 0 | 28 | 34 | Both |
Postcentral gyrus, inferior parietal lobe (BA 2, 3, 40) | 64 | -26 | 28 | Right |
Inferior parietal lobe (BA 1, 2, 3, 40) | 60 | -30 | 40 | Right |
Cuneus, fusiform (BA 18, 19) | 12 | -68 | 0 | Right |
Precentral, middle and inferior frontal gyrus (BA 9) | 42 | 8 | 30 | Right |
Middle and inferior frontal gyrus (dPFC) (BA 46) | 46 | 36 | 16 | Right |
Superior and middle frontal gyrus (BA 100) | 28 | 44 | 14 | Right |
(L) Peak amygdala | -24 | -1.4 | -14.4 | Left |
(R) Peak amygdala | 25.7 | -0.76 | -16.3 | Right |
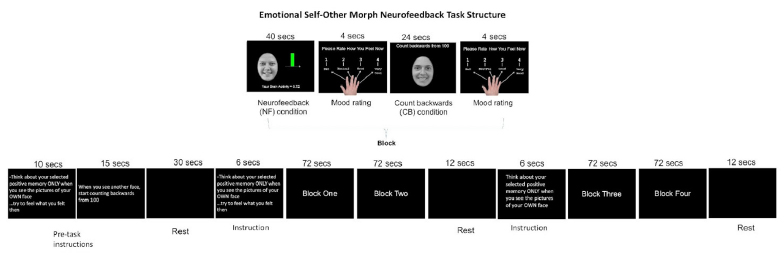
Visual depiction of the Emotional Self Other Morph Task structure, with blocks, time resolution, and task condition illustrated chronologically from left to right.
Available online under under the terms and conditions of the Creative Commons Attribution (CC BY) license (https://creativecommons. org/licenses/by/4.0/) [15].
The goal of the ESOM-NF task was to engage the circuitry of positive self-processing via 'live' amygdala and hippocampus activity feedback provided by MURFI software [39]. Prior to MRI scanning, pictures of participants’ faces with happy, neutral, or sad expressions were obtained, and participants identified positive autobiographical memories with experimenters. The 5.45-minute task was then conducted with 4 blocks of NF training, followed by a control condition (Fig. 1). Within each NF block, participants saw their own happy faces and attempted to increase amygdala and hippocampus activity by recalling happy memories for 40 seconds. The control condition entailed seeing another teens' happy face and counting backward from 100 for 24 seconds, after which participants rated their mood. This contrast control condition allowed for control for neural networks engaged by face processing and working memory that overlap with the tasks engaged during the neurofeedback blocks. Before the start of each condition block, participants read instructions and rested (Fig. 1). A rigorous description of the full study, ESOM-NF protocol, and justification can be found in previous studies [15, 16].
2.2. Acquisition and Preprocessing
The collection of neuroimaging data was done using a 3.0 Tesla Siemens Prisma MRI scanner with a receive-only 32-channel head coil. Acquisition of 3D structural MPRAGE axial images for each participant was performed with parameters (TR/TE: 2,100 ms/3.65 ms; TI: 1,100; Flip Angle 7°; Field of View: 256 × 256 mm; Slice-Thickness: 1 mm; Matrix: 256 × 256; 224 continuous slices). Mean blood oxygen level-dependent (BOLD) GRAPPA 2. images were then acquired with a slice-accelerated gradient echo-planar imaging sequence during 6.02 min for the ESOM-NF task (2.4 mm3 voxels, covering 60 oblique axial slices; TR/TE = 1510/32.4 ms; FOV = 216 × 216 mm; matrix 90 × 90; Flip Angle 65°; multi-band acceleration factor 3). Previous studies indicated that multiband has been successfully used for neurofeedback [15].
For all (N = 53) participants, the final task data consisted of V = 235 volumes or image slices in time, at a time resolution of one image per 1.51s and spatial resolution of 2 mm. Initial preprocessing of echo-planer imaging was performed with SPM12 and included (1) motion correction via rigid body realignment, (2) slice timing correction, (3) rigid body co-registration with high-resolution anatomical data, (4) spatial smoothing (6 mm full-width at half maximum), and (5) head motion outliers, which were identified and corrected with Artifact Detection Tools [15, 16]. FSL was then used for (6) spatial normalization to Montreal Neurological Institute (MNI152) anatomical space via affine transformation, (7) general linear modeling of the BOLD-contrast signal variance, and (8) extracting the ‘mean-extracted’ residual time series from each ROI. In general linear modeling of the BOLD-contrast signal variance (7), condition duration regressors (NF, control) were convolved with the canonical hemodynamic response function, and associated parameters were estimated using restricted maximum likelihood. The final mean-extracted residual time series were ultimately standardized, centered at mean zero, and scaled by ROI to variance 1 for appropriate comparability.
2.3. Regions of Interest
The scope of the study is dichotomized into a primarily targeted analysis of 17 pre-identified ROIs and a secondary exploratory whole-brain analysis of 166 regions from the automated anatomical labeling atlas 3 (AAL3) reported by Rolls et al. [36], with a subsequent ROI-pairwise analysis of differential connectivity between groups.
2.3.1. Predefined Targeted Network
The pre-selected network comprised a collection of significantly active regions during neurofeedback or functionally connected with the amygdala, as identified in prior analyses [15, 16]. These include the peak hippocampi activation locations, most salient or significantly activated regions during the neurofeedback vs. count-backward control condition, most functionally connected regions with the amygdalae, and peak amygdalae activation coordinates for a total of 17 targeted ROIs critical to the activity and connectivity patterns elicited by the ESOM-NF task. FSL was then used to draw 8mm spheres around each ROI’s peak coordinates, guided by the seed definition(s) for connectivity analysis [16] and the PickAtlas toolbox [40] to localize and create the spheres. The resulting targeted functional network of 17 pre-identified, 8mm spherical ROIs and their coordinates is given in Table 1, inducing a total of 17C2 pairwise functional connections between ROIs.
2.3.2. AAL3 Atlas Exploration
The AAL3 atlas presents a macroscopic network of 166 regions across the entire brain, subdividing the anterior cingulate cortex into 26 new areas “not previously defined, but of interest in many neuroimaging investigations” [36]. Unlike the precise, dense network of 17 pre-identified salient ROIs, the AAL3 atlas presents a whole-brain network with many ROIs and potentially unknown connections for discovery. A comprehensive list of AAL3 regions is given in Supplementary Table S1-S2. Final AAL3 analyses were split between individual hemispheres containing 83 regions to reduce multiple comparisons in this reduced sample, inducing a total of 83C2 pairwise functional connections between ROIs.
2.4. Random Covariance Model for Multi-participant fMRI
The random covariance model (RCM) for multivariate multi-participant FC estimation, developed by Zhang et al. [35], was applied to the ESOM-NF task-based fMRI data to evaluate: (1) differential FC within depressed adolescents comparing those with a suicide attempt or ideation, respectively, versus depressed youth without suicide ideation with a targeted network of 17 pre-identified salient ROIs, (2) differential FC between depressed and healthy adolescents for the same set of ROIs, (3) further participant-specific FC correlation with changes in rumination or depression scores pre vs. post neurofeedback, and (4) a novel whole-brain exploration of the recent AAL3 atlas with 166 ROIs.
2.4.1. RCM as a bi-level Graphical Model
The random covariance model is a bi-level graphical model, drawing inference by simultaneously estimating group and participant-specific sparse precision (inverse covariance) matrices, which estimate FC by partial correlation. To induce sparsity and simultaneous participant-group estimation, the RCM takes the form of a penalized Gaussian likelihood employing three penalties {λ}1:3 terms and (λ2) pools information across participants and shrinks participant-specific networks toward a shared group network while (λ1) and (λ3) induce sparsity in the participant-specific and group networks, respectively. Penalties {λ}1:3 are tuned or optimized via modified Bayesian Information Criterion (mBIC) minimization defined by Zhang et al. [35].
Rigorously, let V = 235 denotes the number of fMRI volumes from the ESOM-NF task, P the number of ROIs in the network (P = 17, 83 or 166), and K is the number of participants, then our data may be represented by Y(1), ... , Y(K) matrices of dimension V x P describing the mean-extracted residual BOLD time series in P ROI variables across V volumes or temporal images. Next, a time-invariant Gaussian assumption is made such that each ROI vector is distributed as where Ʃk denotes the kth participant’s covariance matrix. Furthermore, let
denotes the participant-specific precision matrix, Ω0 is the group-level precision matrix, and
is the collection of all parameters or FC networks of interest. Next,
denotes the sample covariance matrix for the kthparticipant, and the penalized Gaussian log-likelihood takes the form as:
![]() |
![]() |
Here, the L1 penalties on {Ω}1:K, Ω 0 by {λ}1,3 are usual LASSO penalties, shrinking unrelated or independent connections to zero and inducing sparsity in each group and participant-specific network. In contrast, λ2 penalizes the Kullback-Leibler divergence between the participant-level precision matrices {Ω}1:K and the group-level Ω 0, inducing similarity between group and participant-specific functional networks. Full derivation, a computational algorithm for estimating , mBIC criterion for tuning {λ}1:3, and asymptotic properties are given by Zhang et al. [35].
To compare FC networks between two groups {A, B} and
were estimated separately, and partial correlation matrices
and
were obtained by
for
and
; yielding our final estimator for differential FC denoted as
. For interpretation, recall that Ω(.) represents precision matrices, which induced partial correlation matrices P(.). Thus for
implies stronger negative FC in group A while
indicates stronger negative FC in B; else in all other cases,
implies stronger positive FC in group A while
indicates stronger positive FC in B. In general, one must ascertain the direction and magnitude of correlation in order to correctly interpret a meaningful or ‘significant’ difference between groups. It must also be noted that partial correlation inherently yields different effect sizes compared to previous marginal correlation results, as marginal correlation does not adjust for extraneous or intermediary interactions with other ROIs in the network. This study, however, is more concerned with significant differences than overall effect sizes in the network.
2.4.2. Inferential Permutation Procedure
When situations arise where a theoretical ‘null’ distribution for hypothesis testing is invalid or unavailable, a synthetic ‘null’ may be simulated through permutation to obtain empirical confidence intervals and p-values for hypothesis testing. To do so, assume that if one were to randomly permute or shuffle the group labels like a deck of cards and refit a model to obtain new estimates, then these estimates would reflect a theoretical ‘null’ population, where there is no difference between groups (by design, as labels were randomly shuffled). If we repeat this process many times, we can reconstruct a synthetic ‘null’ population that can be compared against the original estimate with correct group labels. We may then compute empirical 95% confidence intervals directly from the upper and lower 0.025 quantiles of this synthetic ‘null’ distribution and p-values by literally counting how many values in the synthetic ‘null’ population distribution are as or more extreme, divided by how many permutations or shuffles comprise this empirical distribution.
In this study, we adopt a permutation-based nonpara-
metric procedure for assessing the statistical significance of the estimated between-group FC differences obtained by the RCM. M = 5000 permutations were conducted. For each, group labels were permuted by resampling without replacement, the RCM was refit, computed and ‘unraveled’ or flattened into a vector
of length
, describing each ROI pairwise functional connection. Raw p-values were then obtained by comparing the observed FC difference
against the 5000-permutation null distribution. To adjust for multiple comparisons, a correction by Benjamini et al. [36-41] was used for the full study sample comparison of depressed youth versus healthy controls, and a heuristic threshold of
was employed for the reduced sample of depressed youth with a suicide attempt for inflated type I error rates.
3. RESULTS
3.1. Differential Connectivity between Depressed Youth with a Suicide Attempt and those without during Neurofeedback
Novel differences in connectivity were uncovered within depressed adolescents (ND = 34), between those with a suicide attempt (NS = 15) and those without during neurofeedback. In a new finding, youth with a suicide attempt displayed strong positive connectivity within the left hemisphere (0.053), specifically between the left superior, middle, and inferior temporal gyrus (BA 19, 22, 37, 39) and the left amygdala ( = -0.098, praw = 0.009) compared to negative connectivity in depressed youth without a suicide attempt (-0.0459) (NS = 19) displayed in (Figs. 2, 3, S1, Table S1). Suicide attempters also showed higher insula to the postcentral gyrus and inferior parietal lobe than depressed youth without a suicide attempt (Fig. 2). This higher left amygdala connectivity was similar to independent findings reported by Alarcón et al. [42] using a non-neurofeedback self vs. other face recognition task. Differential connectivity among depressed adolescents, between those with suicide ideation (NI = 29) and without, likewise revealed interesting patterns but interpretation was limited by imbalanced sample sizes and can be found in the Supplementary material.
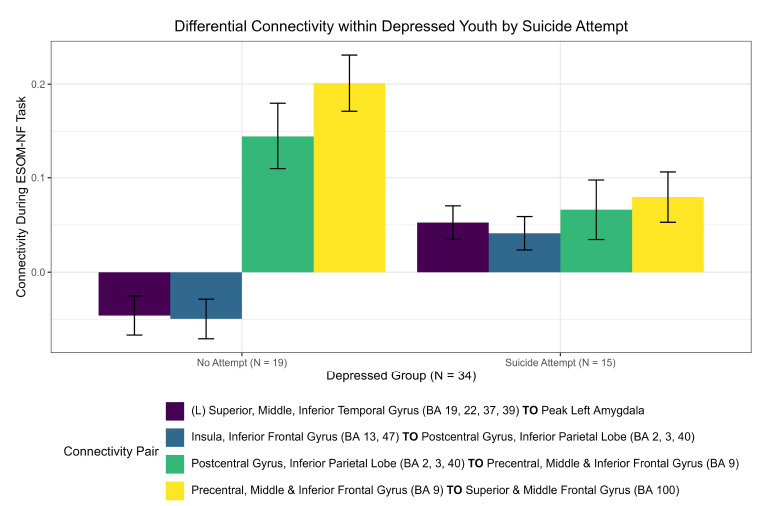
Differential connectivity within depressed youth by suicide attempt.
Significant functional connectivity differences between depressed youth with a suicide attempt versus those without by ROI pair estimated by partial correlation and adjusted for extraneous connectivity within the rest of the network. Depressed adolescents with a suicide attempt elicited significantly higher connectivity in the left hemisphere between the left superior, middle, and inferior temporal gyrus (BA 19, 22, 37, 39) and left amygdala. Conversely, those without an attempt showed higher connectivity in the upper right hemisphere between the superior, middle frontal gyrus (BA 100) and precentral, middle, and inferior frontal gyrus (BA 9).
3.2. Differential Connectivity between Depressed and Healthy Adolescents during Neurofeedback
New differential connectivity results were uncovered between depressed and healthy adolescents during the RCM network analysis of 17 pre-identified ROIs during the ESOM-NF task. Both groups showed negative functional connectivity between the insula, inferior frontal gyrus, putamen (BA 13, 47), and inferior parietal lobe (BA 1, 2, 3, 40) but significantly stronger connectivity ( = -0.088, praw = 0.0006) was observed in healthy controls (-0.105) versus depressed youth (-0.016) during the ESOM-NF task (Figs. 4, 5, S4, S5, Table S2). Furthermore, depressed and healthy adolescents also shared significant differential cross-hemispheric connectivity with the right inferior parietal lobe (BA 1, 2, 3, 40). However, for depressed youth, this ROI was significantly more connected with the left insula and inferior frontal gyrus (BA 13, 47) versus healthy controls, while compared to depressed youth, controls showed a significantly stronger connection with the left amygdala.
These results also corroborated the cross-hemispheric findings reported by Quevedo et al. [16], demonstrating differential functional connectivity between depressed youth and healthy controls by the amygdalae hemisphere. A higher positive right amygdala to cuneus fusiform (BA 18, 19) connection ( = -0.042, praw = 0.041) was observed in depressed adolescents (0.016) versus negative connectivity in healthy youth (-0.026). In contrast, a significantly higher left amygdala to inferior parietal lobe (BA 1, 2, 3, 40) hyper-connection (
= 0.1, praw = 0.001) was observed in healthy controls (0.081) versus negative connectivity in depressed youth (-0.019) during neurofeedback (Figs. 4, 5, S5, Table S2).
4. DISCUSSION
An application of the Random Covariance Model (RCM) [35] yielded novel insight into differential connectivity patterns within depressed adolescents, differentiating suicide attempts or ideation, as well as corroborating previous findings between depressed youth and healthy controls [15, 16, 42]. Improving previous univariate marginal correlation methods, RCM analyses used multivariate partial correlation as an estimator of whole-network functional connectivity. Partial correlation describes the conditional relationship between two brain regions adjusted or controlling for connectivity amongst the rest of the brain network. In other words, stronger conclusions about pair-wise ROI connectivity can be drawn because the influence of network interactions, mediators, or extraneous noise is removed from connectivity estimation. Beyond improved estimation and inference, RCM analyses also uncovered participant-specific connectivity patterns within a diagnostic group for novel applications in targeted neurofeedback and precision medicine. Results from this enhanced methodology were examined, beginning with primary findings differentiating connectivity in depressed youth with a suicide attempt from those without and depressed youth overall compared to healthy controls, followed by an in-depth discussion of neurobiological mechanisms underlying these results. Secondary exploratory analyses of participant-specific connectivity association with mental functioning changes post-neurofeedback were then briefly inspected, ending with consideration regarding the potential limitations of this study.
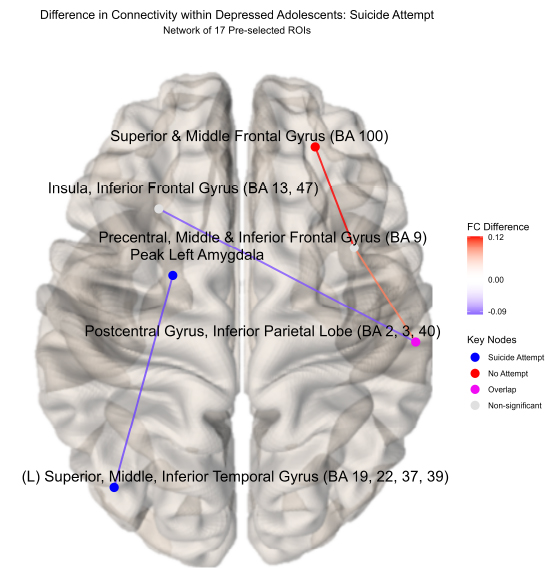
Difference in connectivity within depressed adolescents: suicide attempt( network of 17 pre selected ROIs).
Regions of interests (ROIs) colored blue denote a significantly stronger connection in the depressed adolescents with a suicide attempt (N = 15) compared to those without (N = 19); red denotes the inverse for depressed adolescents without a suicide attempt compared to those with a suicide attempt; pink denotes significant ROI shared between depressed adolescents with or without an attempt. Depressed adolescents with a suicide attempt elicited significantly higher connectivity in the left hemisphere between the left amygdala and the left superior, middle, and inferior temporal gyrus (BA 19, 22, 37, 39). Conversely, those without an attempt showed higher connectivity in the upper right hemisphere between the superior, middle frontal gyrus (BA 100) and precentral, middle, and inferior frontal gyrus (BA 9).
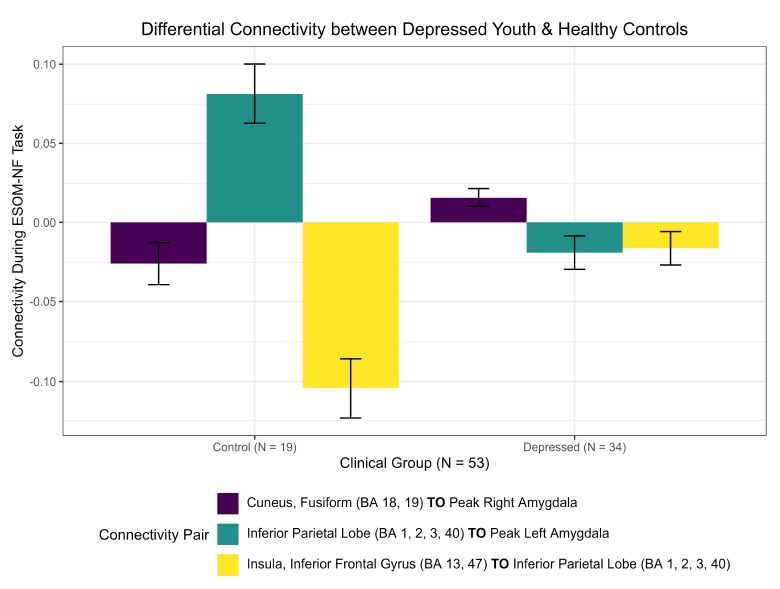
Differential connectivity between depressed youth and healthy controls.
Significant functional connectivity differences between depressed youth versus healthy controls by ROI pair estimated by partial correlation and adjusted for extraneous connectivity within the rest of the network. Healthy controls showed significantly higher connectivity between the left amygdala and right inferior parietal lobe (IPL) compared to depressed youth, whereas depressed youth demonstrated increased (less negative) connectivity between the left insula, inferior frontal gyrus (BA 13, 47), and right IPL. Differential connectivity between the cuneus fusiform (BA 18, 19) and the right amygdala was also observed, corroborating previous results, but was not found to be significant after the permutation procedure.
4.1. Differential Connectivity in Depressed Youth with Suicide Attempt vs. no Attempt
Primary findings of this RCM approach revealed new key brain regions and patterns of connectivity that may differentiate suicide-attempting adolescents from depressed youth without an attempt. For suicide attempters, positive connectivity within the left hemisphere between the superior, middle, and inferior temporal gyrus (BA 19, 22, 37, 39) and the peak left amygdala was observed and was significantly higher compared to negative connectivity shown in depressed non-attempting youth. Given that the fusiform gyrus (BA 37), occipital lobe cortex (BA 19), and temporal sulcus (between BA 22 and 39) are all associated with face perception [43-45], and the left amygdala is associated with conscious, explicit, language-dependent emotional processing [46, 47], this mirrors similar left-amygdala to ACC dynamics found in a previous study [42]. Continuation of this work presents potentially critical targets for precision (participant-specific, precise ROIs) in neurofeedback intervention and, optimistically, future suicide prevention. In contrast, depressed adolescents who had experienced suicidal ideation, often a precursor to an attempt, expressed strong cross-hemispheric negative connectivity between the left anterior cingulate cortex (ACC; BA 6, 8, 9, 24, 32) and right superior, middle, inferior temporal gyrus (BA 19, 22, 37, 39); whereas those without ideation demonstrated no connectivity at all between those regions. This presents an interesting finding as the ACC plays an important role in affecting regulation [48] or controlling and managing uncomfortable emotions, while Brodmann areas (19, 22, 37, 39) are involved in face perception, implying that this functional connection may play a significant role in suicide ideation as those depressed youth without ideation elicited no connectivity whatsoever.
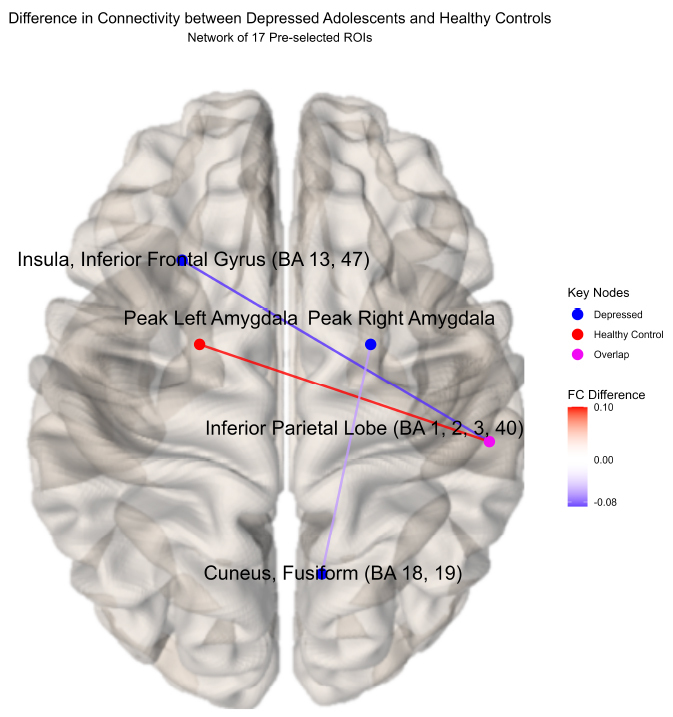
Difference in connectivity between depressed adolescents and healthy controls(network of 17 pre-selected ROIs).
Significant differential cross-hemispheric connectivity was observed between depressed youth (N = 19) and healthy controls (N = 34). Both diagnostic groups elicited differential connectivity regarding the right inferior parietal lobe (IPL; BA 1, 2, 3, 40). Healthy controls showed significantly higher connectivity between the left amygdala and right IPL compared to depressed youth, whereas depressed youth demonstrated increased (less negative) connectivity between the left insula, inferior frontal gyrus (BA 13, 47), and right IPL. Differential connectivity between the cuneus fusiform (BA 18, 19) and the right amygdala was also observed, corroborating previous results, but was not found to be significant after the permutation procedure.
A key region differentiating suicide-attempting youth, the fusiform gyrus, is critically involved in face recognition and facial emotion perception [49]. The amygdala strongly shapes fusiform gyrus function during face perception, which can be further influenced by a participant’s experiences and external stimuli [50]. It has been shown that direct inputs from the amygdala led to an increase in fusiform gyrus activation while witnessing emotional faces. This activation was altered depending on the emotional valence of the faces [51]. Potentially, face perception as a visual process may be under the direct influence of brain regions associated with motivational influences, such as reward and affect. It is hypothesized that having more experience with seeing a variety of emotional faces may lead to a highly activated fusiform gyrus that continuously receives input from the amygdala. Additionally, emotional stress from negative emotions may alter neural circuits responsible for emotion regulation, such as the amygdala, which may also provide a possible explanation for increased amygdala-fusiform gyrus connectivity in suicide-attempting individuals [52].
Aversive stimuli, such as fear, also appear to increase activation in the amygdala and occipital cortex [53]. As amygdala responses are highly controlled by arousing and motivating stimuli, the amygdala may be impacted by a phenomenon known as “negativity bias,” which may influence other regions in the neural network that are involved in visual and emotional processing, such as the occipital lobe cortex [53, 54]. Similar increases in activation in the occipital lobe and amygdala were also seen in participants who viewed negatively valenced words [55]. There was also found to be a significant increase in functional connectivity between the left amygdala and the parieto-occipital cortex when participants were exposed to negative affective stimuli. This suggests that there may be a connection between areas responsible for emotional processing and networks involved in visual processing. Participants with anxiety disorders (such as OCD) exhibited altered connectivity when presented with negative valence stimuli [56]. OCD patients reported significantly increased negative sensitivity in response to negative pictures, and this may also be the case for patients with affective disorders. For patients with affective and anxiety disorders, connections between the amygdala and visual areas may be perceived as “more intense”, which would explain higher connectivity between these areas. Visual areas appear to play an important role in emotional processing mechanisms.
Furthermore, the temporal sulcus has been implicated in processing information related to nonverbal communication, such as facial expression, mouth movement, and eye gaze direction [57]. Neuroimaging studies hypothesized that connectivity between the temporal sulcus and amygdala is implicated in facial perception [58, 59]. Particularly, the amygdala and temporal sulcus modulate reflexive responses to negative visual stimuli [60]. Studies have found that electrical intervention in the right superior temporal sulcus altered the human’s ability to label facial emotions [61]. It has been shown that suicidal ideation and behavior are associated with structural changes in the brain, including a thinner left bank of the superior temporal sulcus [62]. The superior temporal sulcus has also been involved in fear-based hypervigilance in suicide-attempting individuals with borderline personality disorder [52]; thus, it could be hypothesized that suicide-attempting participants may show increased amygdala-temporal sulcus connectivity as a result of hypervigilance to negative emotions. Other than this, little is known in regard to amygdala-temporal sulcus connectivity in suicide-attempting individuals; however, research points to evidence of irregular left amygdala functional connectivity in suicide-attempting individuals [42].
4.2. Differential Connectivity in Depressed Youth vs. Healthy Controls
Primary findings regarding depressed youth vs. healthy controls mirrored previous cross-hemispheric results regarding significant differential right and left amygdala-cuneus connectivity in depressed vs. healthy adolescents, respectively [16]. However, when utilizing a more robust RCM that adjusts for connectivity in the rest of the brain, strong positive connectivity between the left amygdala and the right inferior parietal lobe (IPL; BA 1, 2, 3, 40) was observed in healthy controls compared to weak negative connectivity in depressed youth during neurofeedback. Considering the IPL’s role in social cognition and emotional recognition [63-65] and the fact that the left amygdala likely enables more specialized and explicit emotional processes [66], this implies that healthy controls may be exhibiting more voluntary control of their emotions during neurofeedback than depressed adolescents, implying this could be another connectivity target for future intervention. A novel result also found that healthy controls were also observed to express strong negative connectivity between the insula, inferior frontal gyrus, putamen (BA 13, 47), and inferior parietal lobe (BA 1, 2, 3, 40), whereas depressed adolescents elicited weak negative connectivity by comparison.
The insula is involved in sensorimotor processing, auditory function, and risk and reward behavior [67]. It is thought to be important for characteristics of suicidal behavior, such as risk evaluation and self-recognition [68]. Participants suffering from MDD displayed higher IPL-dorsal agranular insula connectivity compared to healthy controls [69]. As the IPL plays a critical role in the default mode network (DMN) and the cognitive control network (CCN), it is hypothesized that increased IPL activity may be related to impaired function in these networks, such as working memory and attention both of which are common characteristics in MDD patients [70]. Negative connectivity between the posterior inferior parietal lobe and the insula has been shown in healthy subjects, and it may occur due to an interplay of top-down and bottom-up attentional systems [71].
By contrast, the inferior frontal gyrus is involved in response inhibition and attentional control [72], as well as social cognition [73] and executive function [74]. The IPL is connected to the inferior frontal gyrus through the default mode network (DMN) [71]. It could be hypothesized that altered connections between these two areas result in reduced connectivity and deficits in the default mode network. Depressed adolescents with suicide ideation had lower coherence in the default mode network, which is associated with difficulties in self-referential processing and planning [75]. While less is known about the connectivity between the IPL and inferior frontal gyrus, research has found that higher levels of suicidality were associated with greater resting state functional connectivity between the inferior frontal gyrus and right precuneus [76]. Similar to risk and reward behavior associated with the insula, the putamen is involved in reward, cognitive functioning, and learning [77]. Reduced putamen volume has also been associated with impulsivity in neuropsychiatric disorders, including ADHD, bipolar disorder, antisocial personality disorder, and borderline personality disorder [78]. Little is known about the connectivity between the inferior parietal lobe and the putamen. Changes in the inferior parietal lobe and putamen connectivity appear to be connected with changes in dopamine [79]. In terms of suicide, ideation scores were associated with lower dACC connectivity to the putamen [80]. In summary, it could be hypothesized that abnormal changes in connectivity in the putamen and other brain regions may influence impaired decision-making in participants with suicide attempts, while suicide-related changes in inferior frontal gyrus connectivity may be associated with deviations in emotion regulation and affective experience [76].
4.3. Supplemental Analyses Illustrate the Utility of RCM in fMRI and Suggest Future Directions
Secondary analyses exploring participant-specific connectivity analysis within the depressed group, enabled by the RCM’s novel hierarchical design, likewise yielded new exploratory insight into ROIs, which may be associated with improved participant outcomes in pre-post rumination and depression scores (Supplementary material). Considering the correlation between participant-specific functional connectivity and changes in pre-post scores, stronger associations were observed with changes in rumination compared to depression (MFQ). This may be due to changes in self-processing affecting rumination more quickly post-neurofeedback intervention, whereas lasting effects on depression may be more evident or likely given additional follow-up. In addition to strong associations, at the participant level, large differences in variability and magnitude of connectivity across brain connections were also observed. This mode of analysis demonstrates the importance of accounting for participant-specific networks in group connectivity estimation, as well as the precise participant-level connectivity insights the RCM can offer versus other comparable methods like independent graphical LASSO (glasso) or group-fused graphical LASSO. With respect to scope and scale, this methodology may be readily applied to whole-brain networks described by the AAL3 atlas (Supplement) or unsupervised regional atlas derived from unsupervised clustering methods like Independent Component Analysis (ICA), not just the apriori targeted network investigated in our primary analysis. Results, relative to sample size, were inconclusive in these exploratory analyses, but the potential for future novel inquiry into functional connectivity and adolescent depression is clearly demonstrated.
4.4. Limitations of analysis and RCM approach
Limitations of this approach do exist and are primarily evident in the pairwise comparison of many ROI connections versus small sample sizes, especially regarding group-level analyses within the depressed group (ND = 34). This drawback is likewise present in the exploratory participant-specific correlation analysis within that same group, as well as the whole-brain AAL3 atlas, where far more ROIs relative to sample size are present. However, while depression is very heterogeneous and the generalizability of network findings can be difficult to reproduce, we were still able to corroborate and extend important suicide results from a similar study with a different participant sample and neurofeedback task [42], as well as employ a novel multivariate method to improve upon prior univariate analyses from the same study [16]. Though results from our participant-specific connectivity correlation with rumination and depression symptom change may be inconclusive from a ‘statistical significance’ perspective, a sample size of 34 relative to 136 pairwise ROI connections from a network of 17 regions was never sufficiently powered to detect a meaningful difference after multiple comparison adjustment; and this is even more true for the 3,408 comparisons across the whole-brain AAL3 atlas. The purpose of these exploratory results, while potentially biologically meaningful, is more to demonstrate the novel types of analyses and usefulness of the RCM methodology for future applications with increased sample sizes. The most exciting future application lies in the realm of precision medicine, where leveraging individuals’ functional connectivity, given a large overall diagnostic group, could be utilized for participant-specific intervention, symptom reduction, and, optimistically, suicide prevention (Supplementary information).
CONCLUSION
This study introduced the Random Covariance Model (RCM) in the context of a task-based fMRI neurofeedback study of adolescent depression, which provided enhanced insight into differential connectivity patterns for entire functional networks at both the diagnostic-group and individual-participant level. The method’s use of multivariate partial correlation offered a more accurate estimator of functional connectivity between two regions by adjusting or controlling for regional interactions with the rest of the network, overcoming the limitations of previous univariate marginal correlation methods. These novel improvements enabled the identification of specific connectivity patterns both within depressed adolescents, differentiating those with or without a suicide attempt and between depressed youth and healthy controls.
Most notably, increased positive connectivity within the left hemisphere involving the temporal gyrus and amygdala was observed in suicide attempters compared to depressed youth with no attempts. This enhanced connectivity could be linked to altered face perception and emotional processing mechanisms utilized during the unique ESOM-NF task. Comparison between depressed youth and healthy controls revealed strong differential connectivity between the amygdala and parietal lobe, aligning with neurobiology, indicating that healthy adolescents likely have improved emotional control. While that finding may seem obvious, the specific coordinates within those larger brain structures provide a great target for future specialized neurofeedback. Additionally, depressed adolescents with suicidal ideation exhibited distinctive cross-hemispheric connectivity patterns between the anterior cingulate cortex and temporal regions, underscoring the ACC's role in affect regulation.
These neurobiological insights highlight critical areas for potential intervention aimed at reducing suicide attempts and ideation through precise targeting of affected brain regions while simultaneously highlighting whole-network functional connectivity differences in depressed youth versus healthy controls. However, these results are only at the group level. The RCM likewise fits participant-specific networks, which vary around the group and can provide further insight into participant-specific or personalized neurofeedback. For example, correlation or regression analysis with external clinical mental functioning improvement, as we demonstrated here, may also be leveraged to find specific ROI connections that differ significantly from others, within or between diagnostic groups, and may be significantly associated with improved outcomes. This depth of joint analysis at both group- and individual levels is not available in other methods, highlighting the improved utility of the RCM for future fMRI studies.
While the RCM methodology demonstrated new capability and provided novel insights, the primary limitation was the small sample sizes and many ROI-pairwise comparisons relative to sample size. This issue was only exacerbated in the reduced sample comparisons within depressed youth (smaller sample size) and whole-brain AAL3 atlas analysis (more comparisons). Another limitation exists in the potential interaction of medication in either the group- or participant-specific analysis. However, rigorous inclusion/exclusion criteria ought to have minimized any additional effect of medication, as all depressed individuals were on stable medication throughout the study. Future research ought to focus on larger sample sizes to validate findings and increase the power to detect true differences in the face of many pairwise ROI comparisons. Both are necessary to further investigate the potential for an RCM to inform precision neurofeedback and, optimistically, aid in future suicide prevention through targeted intervention. Specifically, this will allow for novel information regarding potential medication interactions with participant-specific connectivity networks and, more generally, will allow for many new questions that were previously unanswerable to be effectively investigated.
LIST OF ABBREVIATIONS
NF, FMRI | = Neurofeedback Functional Magnetic Resonance Imaging |
FC | = Functional Connectivity |
ROIs | = Regions of Interest |
RCM | = Random Covariance Model |
MDD | = Major Depressive Disorder |
AUTHORS’ CONTRIBUTION
L.Z. and K.Q: contributed to the conception and design of the study; J.R. and K.Q.: took part in writing the paper;. Q.N: was involved in data preparation, analysis, and interpretation, and is a primary author. All authors reviewed the results and approved the final version of the manuscript.
AVAILABILITY OF DATA AND MATERIAL
fMRI data used in this study is protected patient data, obtained via Karina Quevedo's NIMH MH092601 grant below, and can be made available upon request to [K.Q].
FUNDING
This study was funded by the National Institute of Mental Health (NIMH), USA - MH092601 - 1R03MH115300.
SUPPLEMENTARY MATERIAL
Supplementary material is available on the publisher's website along with the published article.