All published articles of this journal are available on ScienceDirect.
Information Modeling Technique to Decipher Research Trends of Federated Learning in Healthcare
Abstract
Aim
The aim of this study is to determine the most prevalent types of federated learning, discuss their uses in healthcare, highlight the most significant issues, and suggest methods for further research.
Context
When it comes to handling distributed data, federated learning is revolutionary, especially in sensitive sectors like healthcare. In order to improve the outcomes of the growing number of healthcare studies, there must be a method to safely and effectively analyze and use this enormous data.
Objective
The purpose of this research is to use a large corpus of 6,800 healthcare studies published between 2000 and 2024 and apply topic modeling using Latent Semantic Analysis (LSA).
Methods
The corpus was analyzed using LSA with the goal of identifying latent themes that capture the spirit of federated learning in the healthcare industry. In order to provide an organized overview of the subject matter, a five-topic solution was devised. To guarantee relevance and clarity, the topics' coherence was assessed.
Results
The term frequency and the inverse document frequency of high-loading terms provided five major topic solutions. The coherence score of the five-topic solution was achieved, i.e., 0.789, indicating a high level of relevance and integration among the identified topics. Different types of federated learning (FL), applications of FL, and the key challenges and the possible solution associated with FL have been analyzed.
Conclusion
This study highlights the significance of using FL to improve privacy-preserving data analysis in the healthcare field, which may lead to the development of creative solutions for complex problems.
1. INTRODUCTION
The availability of several sophisticated tools has led to a significant increase in processing power and data analysis capabilities in various industries and sectors [1], which has further facilitated the ongoing enhancement of artificial intelligence. Various practical applications, including robots, categorized agriculture, and e-healthcare, undergo the ML and DL processes to meet their specific requirements. AI-powered computers surpass humans in terms of cognition and cognitive abilities to the point where robots possess more knowledge than human beings [2]. The collaboration of artificial intelligence (AI), machine learning (ML), and deep learning (DL) has far exceeded our expectations in terms of cognitive capacities. This has resulted in remarkable and thrilling advancements in technology, bringing us great joy. The integration of machine learning and deep learning systems into electronic health records has emerged as a means to enhance the accuracy of medical decision-making. The size of the data results in its yielding [3]. The stringent constraints imposed by HIPAA serve to protect patients' information from healthcare institutions, yet also pose challenges in obtaining data from healthcare businesses. DL-based models must possess resistance to attacks, algorithmic accountability, and data security in order to comply with the regulatory criteria set by the HIPAA regulatory act. Therefore, it will include an upgrading procedure that has the potential to optimize the models. A privacy-preserving and distributed system may be constructed by using pre-trained DL-based models obtained via the process of Federated Learning (FL). Recently FL has emerged as the primary location for researching privacy-preserving frameworks [4]. The emerging field of data privacy maintenance development is actively seeking researchers to build decentralized methods. The objective is to distribute a standardized configuration among many customers, including cloud and virtualization service providers while maintaining the integrity of each individual record. FL is a training technique for DL models that leverages local data at the edge for distributed computing across numerous devices in close proximity to the end user. Instead of gathering data from a central perspective, machine learning models are implemented on edge devices [5]. The process involves updating a single centralizing server with the newest learned model at each step. In order to overcome obstacles like data silos, restricted access, and limited dataset availability, FL allows several nodes to construct an ideal learning model. Among the many fields that may benefit from this distributed learning are pharmaceutics, healthcare, medical artificial intelligence, telecom, autonomous cars, mammography classification, and traffic monitoring and flow prediction [6]. The growth of FL is shown in Fig. (1).
Systematic reviews of the literature adhere to a strict four-phase approach, which involves identification, screening, eligibility evaluation, and inclusion of relevant articles for quantitative synthesis. This procedure is mostly directed by the recommended reporting elements for systematic reviews and meta-analyses (PRISMA) guidelines [7]. This work follows PRISMA principles in identifying qualitative literature related to FL. Various focus groups have been examined however researchers generally agree on search keywords. Previous evaluations have often overlooked the intersection of works from adjacent sectors, such as education and reading, creative styles, thematic components, genres, and more. Latent Semantic Analysis (LSA), a quantitative tool, is very advantageous in FL and related domains for identifying and comprehending the literary style of texts. The LSA approach automatically establishes connections and inferences based on the contextual occurrence of words [8]. Researchers may use
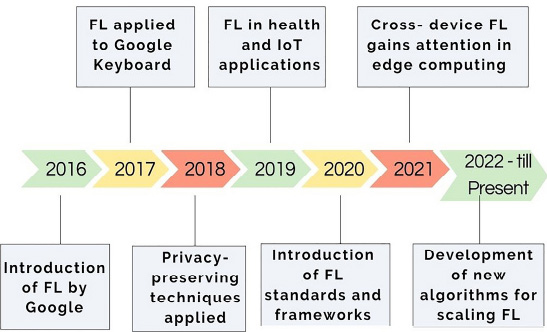
Development of federated learning.
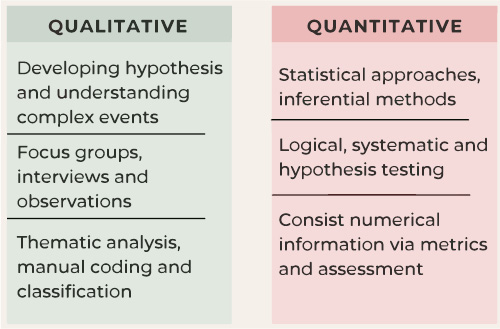
Difference between qualitative and quantitative approach.
quantitative approaches such as LSA to conduct unbiased qualitative analysis of textual content, enabling them to investigate individual or organizational queries. In Fig. (2), the authors illustrate a system that may automatically analyze texts to remove unconscious bias and enable the comparison of qualitative and quantitative research methodologies. The PRISMA criteria were used to select potential articles in the future literature search. The conversion of qualitative data into quantitative data was accomplished using LSA. LSA's capacity to analyze word occurrences within a context-based qualitative corpus stems from its mathematical representation of words, synonyms, and metaphors, elucidating many semantic features in the analyzed qualitative literature [9]. LSA utilizes interpretable dimensions to summarize a set of texts by comparing and categorizing them, generating new categories from the document corpus.
This study aims to address the following research questions:
RQ1: What are the different types of federated learning?
RQ2: What are the various healthcare application areas of federated learning?
RQ3: What are the key challenges and possible future directions of federated learning in healthcare Metaverse?
1.1. Motivation
• Integrating LSA into federated learning introduces a new research avenue that might enhance capabilities and tackle issues related to learning from decentralized, privacy-focused datasets.
• Applying LSA inside the FL framework may greatly improve learning results, privacy protection, and communication efficiency.
• LSA may enhance communication efficiency between client and server by reducing data dimensionality and extracting pertinent characteristics from unstructured data, thereby easing bandwidth limitations.
1.2. Highlights of the Study
• LSA was first utilized in the field of federated learning.
• 6800 research studies published between 2000-2024 were analyzed using the LSA technique.
• Using LSA can enhance the comprehension of data in FL, allowing for more significant feature extraction and decrease in dimensionality, ultimately resulting in improved model performance.
• The coherence score that has been achieved for five topic solutions is 0.789.
1.3. Structure of the Study
The research study is structured into five separate parts, each playing a crucial role in presenting the study's aims, techniques, results, and conclusions. Section 1 introduces Federated Learning by providing a detailed overview of its fundamental ideas and importance as a cutting-edge learning approach. Section 2 explores the authors' methodological framework, including the new methods and methodologies used in their study. Section 3 delves into a thorough discussion of the research question, providing incisive analysis and interpretation of the results within the framework of previous literature. The limitations of the study have been discussed in Section 4. Section 5 concludes the study by summarizing the important conclusions and contributions and proposing directions for further research on this evolving topic.
2. MATERIALS AND METHODS
2.1. Data Sources
The authors conducted an in-depth investigation on FL by following the PRISMA guidelines to facilitate the search, selection, and preparation of a corpus for an automated review utilizing LSA. This systematic technique helped in systematically and transparently discovering and analyzing relevant material, assuring the strength and dependability of the review process. The authors carried out an in-depth investigation by systematically researching and surveying many academic databases and digital libraries to gather a large collection of research papers and technical reports on the subject of Federated Learning. The websites and sources used include Google Scholar, Mendeley, ACM Digital Library, as well as Taylor & Francis, IEEE Xplore, and Scopus. The platforms were chosen regardless of their demographic coverage in order to be equally applicable over the whole FL area. After collecting the research articles from the mentioned sources, the articles were again manually reviewed by the authors as per the inclusion/exclusion criteria, as shown in Table 1.
2.2. Search String
The authors used a strategic search string strategy to perform their complete assessment of the literature on Federated Learning, ensuring an efficient and successful search for relevant research publications across several sources. This carefully created and optimized search string included important keywords, Boolean operators, and particular phrases relevant to Federated Learning. The reason for employing such a search string was twofold: to maximize the relevancy of returned articles and to guarantee that the search could be reproduced by researchers in the future. For example, the authors have used main keywords along with the Boolean operators such as AND, OR, NOT and formed the final string that is used for the collection of data sources:
(“Federated learning” OR FL) AND (health OR medical)
Inclusion Criteria | Exclusion Criteria |
---|---|
Articles must be published in English. | Studies not published in English. |
Studies published from 2000 onwards. | Research studies published before 2000. |
Only articles from peer-reviewed journals and conferences. | Articles not subjected to a peer-review process. |
Directly related to Federated Learning, including methodologies, applications, challenges, or advancements. | Studies not specifically addressing Federated Learning or its applications. |
Empirical studies, theoretical frameworks, case studies, and reviews. | Studies with incomplete data or findings |
2.3. Selection of Studies
The authors collected a large amount of data by obtaining 12,000 articles from the specified sources. The initial collection was carefully examined manually, following strict inclusion and exclusion criteria, resulting in the refinement of the selection to 9,600 relevant articles. After the first curation, the corpus underwent more stringent filtering to remove any remaining non-relevant articles. This process refined the collection to 7,800 items very relevant to the study question. The authors carried out a comprehensive duplication process in the next refining step, finding and eliminating every duplicate article in the dataset. After a meticulous process, redundant articles were removed, providing an improved collection of 6,800 research articles, as shown in Fig. (3). After undergoing a thorough evaluation process to ensure relevance, specificity, and originality, these articles made up the final dataset designated for LSA.
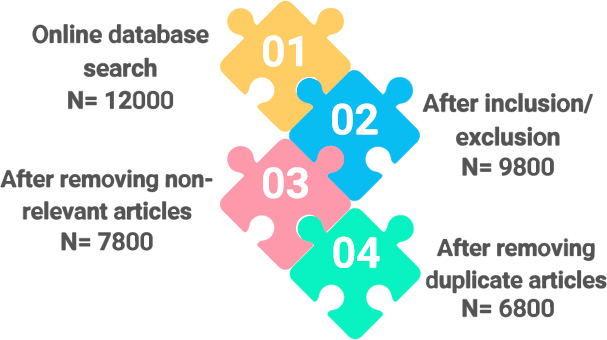
Research studies selection process.
Pre-processing Steps | Document ID | ||||
---|---|---|---|---|---|
Doc1 | Doc2 | Doc3 | Doc4 | Doc5 | |
Sample abstract | A disease is an abnormal condition that negatively impacts the functioning of the human body. Pathology determines the causes behind the disease and identifies its development mechanism and functional consequences. | Data privacy is a very important issue. Especially in fields like medicine, it is paramount to abide by the existing privacy regulations to preserve patients’ anonymity. | The use of machine learning (ML) with electronic health records (EHR) is growing in popularity as a means to extract knowledge that can improve the decision-making process in healthcare. | Recent advances in deep learning have shown many successful stories in smart healthcare applications with data-driven insight into improving clinical institutions’ quality of care. Excellent deep learning models are heavily data-driven. | Recent advances in communication technologies and the Internet-of-Medical-Things (IOMT) have transformed smart healthcare enabled by artificial intelligence (AI). |
After tokenization | ‘A’, ‘disease’, ‘is’, ‘an’, ‘abnormal’, ‘condition’, ‘that’, ‘negatively’, ‘impacts’, ‘the’, ‘functioning’, ‘of’, ‘the’, ‘human’, ‘body’,’.’, ‘Pathology’, ‘determines’, ‘the’, ‘causes’, ‘behind’, ‘the’, ‘disease’, ‘and’, ‘identifies’, ‘its’, ‘development’, ‘ mechanism’, ‘and’, ‘functional’, ‘consequences’,’.’ | ‘data’, ‘privacy’, ‘is’, ‘a’, ‘very’, ‘important’, ‘issue’,’.’, ‘Especially’, ‘in’, ‘fields’, ‘like’, ‘medicine’,’,’, ‘it’, ‘is’, ‘paramount’, ‘to’, ‘abide’, ‘by’, ‘the’, ‘existing’, ‘privacy’, ‘regulations’, ‘to’, ‘preserve’, ‘patients’, ‘anonymity’,’.’ | ‘The’, ‘use’, ‘of’, ‘machine’, ‘learning’, ‘(‘, ‘ML’, ‘)’, ‘with’, ‘electronic’, ‘health’, ‘records’, ‘(‘, ‘HER’, ‘)’, ‘is’, ‘growing’, ‘in’, ‘popularity’, ‘as’, ‘a’, ‘means’, ‘to’, ‘extract’, ‘knowledge’, ‘that’, ‘can’, ‘improve’, ‘the’, ‘decision’, ’-‘, ‘making’, ‘process’, ‘in’, ‘healthcare’, ‘.’ | ‘Recent’, ‘advances’, ‘in’, ‘deep’, ‘learning’, ‘have’, ‘shown’, ‘many’, ‘successful’, ‘stories’, ‘in’, ‘smart’, ‘healthcare’, ‘applications’, ‘with’, ‘data’, ‘-‘, ‘driven’, ‘insight’, ‘into’, ‘improving’, ‘clinical’, ‘institutions’, ‘quality’, ‘of’, ‘care’, ‘.’, ‘Excellent’, ‘deep’, ‘learning’, ‘models’, ‘are’, ‘heavily’, ‘data’, ‘-‘, ‘driven’, ‘.’ | ‘Recent’, ‘advances’, ‘in’, ‘communication’, ‘technologies’, ‘and’, ‘the’, ‘Internet’, ‘-‘, ‘of’, ‘-‘, ‘Medical’, ‘-‘ ‘Things’, ‘(‘, ‘IOMT’, ‘)’, ‘have’, ‘transformed’, ‘smart’, ‘healthcare’, ‘enabled’, ‘by’, ‘artificial’, ‘intelligence’, ‘(‘, ‘AI’, ‘)’, ‘.’ |
After removing stop words | ‘disease’, ‘abnormal’, ‘condition’, ‘negatively’, ‘impacts’, ‘functioning’, ‘human’, ‘body’, ‘Pathology’, ‘determines’, ‘causes’, ‘behind’, ‘disease’, ‘identifies’, ‘development’, ‘ mechanism’, ‘functional’, ‘consequences’ | ‘data’, ‘privacy’, ‘very’, ‘important’, ‘issue’, ‘Especially’, ‘in’, ‘fields’, ‘like’, ‘medicine’, ‘it’, ‘paramount’, ‘to’, ‘abide’, ‘by’, ‘existing’, ‘privacy’, ‘regulations’, ‘to’, ‘preserve’, ‘patients’, ‘anonymity’ | ‘use’, ‘machine’, ‘learning’, ‘ML’, ‘with’, ‘electronic’, ‘health’, ‘records’, ‘HER’, ‘growing’, ‘in’, ‘popularity’, ‘as’, ‘means’, ‘to’, ‘extract’, ‘knowledge’, ‘can’, ‘improve’, ‘decision’, ‘making’, ‘process’, ‘in’, ‘healthcare’, | ‘Recent’, ‘advances’, ‘in’, ‘deep’, ‘learning’, ‘have’, ‘shown’, ‘many’, ‘successful’, ‘stories’, ‘in’, ‘smart’, ‘healthcare’, ‘applications’, ‘with’, ‘data’, ‘driven’, ‘insight’, ‘into’, ‘improving’, ‘clinical’, ‘institutions’, ‘quality’, ‘care’, ‘Excellent’, ‘deep’, ‘learning’, ‘models’, ‘are’, ‘heavily’, ‘data’, ‘driven’ | ‘Recent’, ‘advances’, ‘in’, ‘communication’, ‘technologies’, ‘and’, ‘the’, ‘Internet’, ‘of’, ‘Medical’, ‘Things’, ‘IOMT’, ‘have’, ‘transformed’, ‘smart’, ‘healthcare’, ‘enabled’, ‘by’, ‘artificial’, ‘intelligence’, ‘AI’ |
After stemming and lemmatization | ‘diseas’, ‘abnorm’, ‘condit’, ‘neg’, ‘impact’, ‘function’, ‘human’, ‘bodi’, ‘Patholog’, ‘determin’, ‘caus’, ‘behind’, ‘diseas’, ‘identifi’, ‘develop’, ‘ mechan’, ‘functional’, ‘consequ’ | ‘data’, ‘privaci’, ‘very’, ‘important’, ‘issue’, ‘especi’, ‘in’, ‘field’, ‘like’, ‘medicin’, ‘it’, ‘paramount’, ‘to’, ‘abid’, ‘by’, ‘existing’, ‘privaci’, ‘regul’, ‘to’, ‘preserv’, ‘patient’, ‘anonym’ | ‘use’, ‘machin’, ‘learn’, ‘ML’, ‘with’, ‘electron’, ‘health’, ‘record’, ‘HER’, ‘grow’, ‘in’, ‘popular’, ‘as’, ‘mean’, ‘to’, ‘extract’, ‘knowledg’, ‘can’, ‘improv’, ‘decis’, ‘mak’, ‘process’, ‘in’, ‘healthcar’, | ‘Recent’, ‘advanc’, ‘deep’, ‘learn’, ‘have’, ‘shown’, ‘mani’, ‘success’, ‘stori’, ‘smart’, ‘healthcar’, ‘applic’, ‘data’, ‘driven’, ‘insight’, ‘improv’, ‘clinic’, ‘institut’, ‘qualiti’, ‘care’, ‘excel’, ‘model’, ‘are’, ‘heavili’ | ‘recent’, ‘advanc’, ‘commun’, ‘technolog’, ‘Internet’, ‘of’, ‘medic’, ‘thing’, ‘iomt’, ‘have’, ‘transform’, ‘smart’, ‘healthcar’, ‘enabl’, ‘by’, ‘artifici’, ‘intellig’, ‘ai’ |
3-character filtering | ‘diseas’, ‘abnorm’, ‘condit’, ‘impact’, ‘function’, ‘human’, ‘bodi’, ‘Patholog’, ‘determin’, ‘caus’, ‘behind’, ‘diseas’, ‘identifi’, ‘develop’, ‘ mechan’, ‘functional’, ‘consequ’ | ‘data’, ‘privaci’, ‘very’, ‘important’, ‘issue’, ‘especi’, ‘field’, ‘like’, ‘medicin’, ‘paramount’, ‘abid’, ‘existing’, ‘privaci’, ‘regul’, ‘preserv’, ‘patient’, ‘anonym’ | ‘machin’, ‘learn’, ‘ML’, ‘with’, ‘electron’, ‘health’, ‘record’, ‘HER’, ‘grow’, ‘popular’, ‘mean’, ‘extract’, ‘knowledg’, ‘improv’, ‘decis’, ‘mak’, ‘process’, ‘healthcar’, | ‘Recent’, ‘advanc’, ‘deep’, ‘learn’, ‘have’, ‘shown’, ‘mani’, ‘success’, ‘stori’, ‘smart’, ‘healthcar’, ‘applic’, ‘data’, ‘driven’, ‘insight’, ‘improv’, ‘clinic’, ‘institut’, ‘qualiti’, ‘care’, ‘excel’, ‘model’, ‘heavili’ | ‘recent’, ‘advanc’, ‘commun’, ‘technolog’, ‘Internet’, ‘medic’, ‘thing’, ‘iomt’, ‘have’, ‘transform’, ‘smart’, ‘healthcar’, ‘enabl’, ‘artifici’, ‘intellig’, |
3. RESULTS
The literary collection was fed into the LSA model in order to uncover the semantic structure at its core. LSA provides a way to automatically organize, understand, search, and summarize a corpus of texts; it is a method for natural language processing. According to a study [10], it finds concepts by analyzing the dataset's document-word correlation. Using Singular Value Decomposition (SVD), this unsupervised text-mining method creates a reduced-dimensional space for finding connections, themes, and document comparisons. In addition, it is a well-established technique for discovering common research trends in a large body of literature. Advice on how to use the method is based on what was found in a study [11]. Using the quick truncated incremental stochastic SVD approach with a single pass, the study intended to identify the underlying structure of the corpus using the factor analysis extension to LSA. A word loading matrix and a document-loading matrix are both produced by LSA [12]. Examine the term-loading matrix to see which subjects have the most densely packed terms. You can see which papers are popular and which themes are connected to them in the document-loading matrix. When loading numbers are greater, it means that the topic is more familiar to you. Table 2 shows a five-topic solution that exemplifies five latent classes, associated keywords, and corresponding labels. After applying the LSA to the literature collection, an empirical investigation was conducted, and the term-loading matrix was created. It also comprises the language's highly-loading terms.
3.1. Pre-processing and Filtering
Filtering and pre-processing phrases was the first step in building the corpus. For text-mining methods to work, the literature collection must be pre-processed. During the pre-processing stage, the LSA uses the letters, words, and sentences it detects as tokens for further processing. Improving the text-mining process' efficiency and efficacy while decreasing the dictionary's size is the goal of this step. The process followed the guidelines laid down [13] and included the elimination of certain characters, digits, acronyms, slang, abbreviations, etc. Following the steps below, the corpus preparation procedure was carried out using the Natural Language Toolkit (NLTK) available at (http://www.nltk.org). The results are displayed in Table 2.
• Tokenization was used to separate the titles and abstracts of each article into separate phrases.
• A lowercase version of the tokens was applied to every document. It is now free of punctuation marks such as periods, exclamation points, commas, apostrophes, question marks, quote marks, and hyphens.
• Only numbers containing alphanumeric words were considered.
• Terms with less than three characters were excluded from the keyword set using N-character filtering. Stop-words in English were eliminated. Excluding words with a frequency of one in a text further narrowed the dataset.
The dataset originally had 97,950 tokens. The token count was decreased to 15,150 after the pre-processing stage. Six thousand eight hundred sparse vectors were generated using 15,510 tokens in this research. The dataset, including 6800 papers, was transformed into a vector space. Each row in the vector space represented one of the 15,510 phrases, and each column represented an article. After that, each piece of paper was changed into a “bag-of-words” structure. The mapping method created a dictionary by assigning each word an integer Identity (ID) and tracking how often it appeared in each document. A weighted matrix was generated by advancing the dictionary to the next step of the process.
3.2. Term Frequency (TF)-Inverse Document Frequency (IDF)
A method called TF-IDF grading was used to show how important an item was compared to other things (like terms or documents) in the collection. A word's weight was changed based on how often it appeared in the text, but sometimes, the term's frequency in the collection overrode this change. This helped change how much weight was given to terms that came up more often. It also leads to a better topic study. A number of different TF-IDF balancing methods could be used. In Eq. (2), Wi, j, tf, df, and nd stand for the TF-IDF weight, term frequency, document frequency, and the total number of documents in the dataset, respectively. This equation shows how the study was done. The term frequency (Eq. 1) tells us how often a word appears in a document, while the inverse document frequency (log2 (nd/dfi)) tells us how important a word is in the whole set of documents.
![]() |
(1) |
A weighted matrix based on terms and documents was generated by multiplying the term's frequency by the inverse document frequency. The weighting approach outlined in Eq. (2) was used to construct a term-document weighted matrix of size 15510×6800 for the ith term in the jth document of a corpus consisting of nd documents. All of the chosen topic solutions were given the same weight. A weighted matrix of i*j words is generated for the jth document in the nd document corpus to collect answers on different topics. Table 3 shows the document term matrix, which indicates the frequency of a word inside a certain sample document. The matrix represents words and documents as rows and columns, respectively.
![]() |
(2) |
The TF calculation considers all words equally essential, but the IDF evaluates the relevance of a phrase inside a particular text and throughout the whole corpus. Common phrases like “based,” “that,” and domain-specific jargon may occur often in numerous books, but they don't add anything. Since a consequence, IDF must be used to magnify unusual terms while decreasing frequent ones since the former may lead to biased generalizations. Tables 4 and 5 show the TF and IDF scores obtained from Eqs. (1 and 2) for the example texts.
LSA and other approaches in information retrieval and text mining use the IDF score to assess the significance of a term within a collection of documents or a corpus. The TF score quantifies how often a phrase occurs in a particular document, whereas IDF evaluates the uniqueness or prevalence of a term over the whole corpus. TF-IDF, a mixture of TF and IDF scores, is useful for emphasizing significant and unique terms in a document, enhancing the efficiency of information retrieval and text analysis techniques.
Terms | Doc1 | Doc2 | Doc3 | Doc4 | Doc5 |
---|---|---|---|---|---|
Diseas | 1 | 0 | 0 | 0 | 0 |
Abnorm | 1 | 0 | 0 | 0 | 0 |
Identifi | 1 | 1 | 0 | 0 | 0 |
Impact | 1 | 0 | 0 | 0 | 0 |
Mechan | 1 | 0 | 1 | 1 | 0 |
Human | 1 | 0 | 0 | 0 | 0 |
Differ | 1 | 1 | 1 | 0 | 0 |
Data | 1 | 1 | 1 | 1 | 1 |
Privaci | 1 | 1 | 0 | 1 | 1 |
Veri | 0 | 1 | 0 | 0 | 0 |
Especi | 0 | 1 | 0 | 0 | 0 |
Regul | 0 | 1 | 0 | 1 | 0 |
Field | 0 | 1 | 0 | 0 | 0 |
Like | 0 | 1 | 0 | 0 | 0 |
Machin | 0 | 1 | 1 | 0 | 0 |
Grow | 0 | 0 | 1 | 0 | 0 |
Electron | 0 | 0 | 1 | 0 | 0 |
Improv | 0 | 0 | 1 | 1 | 0 |
Decis | 0 | 0 | 1 | 0 | 0 |
popular | 0 | 0 | 1 | 0 | 0 |
Process | 0 | 0 | 1 | 0 | 1 |
Recent | 0 | 0 | 0 | 1 | 1 |
Commun | 0 | 0 | 0 | 0 | 1 |
Driven | 1 | 0 | 0 | 0 | 0 |
Stori | 0 | 0 | 0 | 1 | 0 |
Smart | 0 | 0 | 0 | 1 | 0 |
Improv | 0 | 0 | 1 | 1 | 0 |
Clinic | 0 | 0 | 0 | 1 | 0 |
Technolog | 0 | 0 | 0 | 0 | 1 |
Medic | 0 | 1 | 0 | 0 | 0 |
Iomt | 0 | 0 | 0 | 0 | 1 |
Intellig | 0 | 0 | 0 | 0 | 1 |
Transform | 0 | 0 | 0 | 0 | 1 |
Enabl | 0 | 0 | 0 | 0 | 1 |
Artific | 0 | 0 | 0 | 0 | 1 |
Document id | TF Scores |
---|---|
Doc1 | {‘diseas’: 0.055901, ‘abnorm’: 0.006211, ‘condit’: 0.006211, ‘impact’: 0.006211, ‘function’: 0.012422, ‘human’: 0.006211, ‘bodi’: 0.006211, ‘patholog’: 0.006211, ‘determin’: 0.006211, ‘caus’: 0.006211, ‘behind’: 0.006211, ‘identifi’: 0.018634, ‘develop’: 0.012422, ‘mechan’: 0.006211, ‘functional’: 0.018634, ‘consequ’: 0.006211} |
Doc2 | {‘data’: 0.024845, ‘privaci’: 0.006211, ‘veri’: 0.009259, ‘important’: 0.009259, ‘issue’:, ‘especi’: 0.009259, ‘field’: 0.009259, ‘like’: 0.009259, ‘medicin’: 0.009259, ‘paramount’: 0.009259, ‘abid’: 0.006211, ‘existing’: 0.009259, ‘privaci’: 0.009259, ‘regul’: 0.009259, ‘preserv’: 0.006211, ‘patient’: 0.009259, ‘anonym’: 0.006211} |
Doc3 | {‘machin’: 0.011765, ‘learn’: 0.043478, ‘ML’: 0.047059, ‘with’: 0.043478, ‘electron’: 0.011765, ‘health’: 0.011765, ‘record’: 0.011765, ‘HER’: 0.047059, ‘grow’: 0.011765, ‘popular’: 0.011765, ‘mean’: 0.043478, ‘extract’: 0.011765, ‘knowledg’: 0.011765, ‘improv’: 0.047059, ‘decis’: 0.011765, ‘mak’: 0.043478, ‘process’: 0.043478, ‘healthcar’: 0.011765} |
Doc4 | {‘recent’: 0.008929, ‘advanc’: 0.008929, ‘deep’: 0.008929, ‘learn’: 0.008929, ‘have’: 0.008929, ‘shown’: 0.008929, ‘mani’: 0.008929, ‘success’: 0.008929, ‘stori’: 0.008929, ‘smart’: 0.008929, ‘healthcar’: 0.008929, ‘applic’: 0.008929, ‘data’: 0.017857, ‘driven’: 0.017857, ‘insight’: 0.017857, ‘improv’: 0.017857, ‘clinic’: 0.008929, ‘institut’: 0.008929, ‘qualiti’: 0.008929, ‘care’: 0.008929, ‘excel’: 0.008929, ‘model’: 0.008929, ‘heavili’: 0.008929} |
Doc5 | {‘recent’: 0.008929, ‘advanc’: 0.008929, ‘commun’: 0.013333, ‘technolog’: 0.013333, ‘Internet’: 0.013333, ‘medic’: 0.013333, ‘thing’: 0.013333, ‘iomt’: 0.013333, ‘have’: 0.013333, ‘transform’: 0.013333, ‘smart’: 0.013333, ‘healthcar’: 0.013333, ‘enabl’: 0.013333, ‘artifici’: 0.013333, ‘intellig’: 0.013333} |
Term | IDF Score | Term | IDF Score | Term | IDF Score | Term | IDF Score | Term | IDF Score |
---|---|---|---|---|---|---|---|---|---|
Diseas | 0.698970 | Data | 0.064815 | Machin | 0.397940 | Recent | 0.397940 | Technolog | 0.698970 |
Abnorm | 0.698970 | Privaci | 0.096910 | Grow | 0.397940 | Commun | 0.698970 | Medic | 0.698970 |
Identifi | 0.397940 | Veri | 0.698970 | Electron | 0.698970 | Driven | 0.698970 | Iomt | 0.698970 |
Impact | 0.698970 | Especi | 0.698970 | Improv | 0.397940 | Stori | 0.698970 | Intellig | 0.698970 |
Mechan | 0.221849 | Regul | 0.397940 | Decis | 0.698970 | Smart | 0.397940 | Transform | 0.698970 |
Human | 0.698970 | Field | 0.698970 | Popular | 0.698970 | Improv | 0.397940 | Enabl | 0.698970 |
Differ | 0.221849 | Like | 0.698970 | Process | 0.397940 | Clinic | 0.698970 | Artific | 0.698970 |
3.3. Singular Vector Decomposition
Singular Vector Decomposition (SVD) is a key method in linear algebra that is extensively used in signal processing, statistics, data science, and machine learning. It offers a method to break down a matrix into three smaller matrices, exposing several beneficial characteristics of the original matrix. SVD breaks down any m×n matrix X into the product of three matrices. X=UΣVt; As its columns represent the left singular vectors of X, U is an orthogonal matrix with dimensions m×m. As shown in Fig. (4), the diagonal members of the diagonal matrix ∑ are non-negative real numbers, and the matrix has dimensions m×n. (Modi & Singh, 2022) Singular values of matrix X are these entries. It is common practice to arrange the values in descending order. Finding out X's rank and condition number is possible with the help of the singular values. An n×n orthogonal matrix, where the columns stand for the right singular vectors of X, is transposed to form Vt. For each subject, the mathematical formulas XXt and XtX were used to determine the term-loading and document-loading, as shown in Eqs. (3 and 4). In decreasing order, t denoted the subject weights, which are represented by single numbers. It was possible to generate as many themes as there were texts in the corpus. Extracting the top k singular values from the matrix ∑∑t allowed us to discover a few themes (k).
![]() |
(3) |
![]() |
(4) |
3.4. Identification of Best Possible Topic Solution
Dimensionality reduction yields optimal topic loadings. Using the term matrix it generates, it meticulously analyzes the data to find the k best phrases or values. The complexity of the topic's content and the number of steps needed to arrive at a suitable topic value have made this process challenging. Optimal topic loadings for a 6800 document corpus are about five, as seen in Table 6, according to the proposal made by. One may use it to spot patterns in federated learning studies.
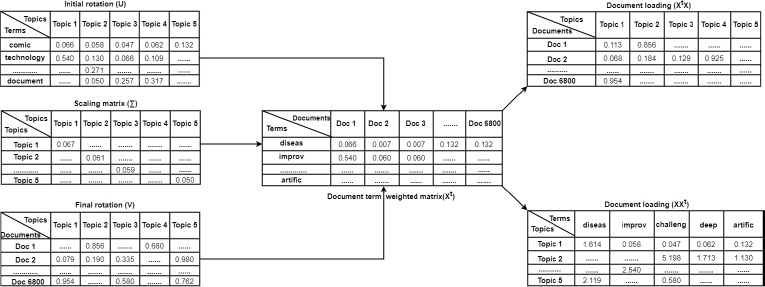
Term and document loading.
Topic ID | Topic Label | High Loading Terms |
---|---|---|
T5.1 | Federated Learning Technologies | Federated, machine, learning, Smart, recent, advance, commun, technolog, |
T5.2 | Federated Learning in Disease Detection | Artificial, intelligence, Diseas, detect, improve, function, develop, FL, learning |
T5.3 | Case Studies and Solutions in Federated Research | AI, Improve, case, solute, studi, electron, health |
T5.4 | Data Privacy in AI and Machine Learning | ML, Site, preserv, very, import, issu, AI, DL |
T5.5 | Robustness in Data-Driven Federated Models | FL, Deep, learning, challeng, datadriven, robust, shown |
3.5. Choosing Threshold Values
Both the word loading matrix and the document loading matrix show the relative importance of the revealed topics. In other words, each row and column of the loading matrix for terms and documents has a loading value that corresponds to a word or document. Loading matrices could have positive or negative values. In order to make sense of the data provided by the loading matrices, the Varimax rotation was applied. As a consequence, a certain problem noticed more loading than others. The significance of a topic is determined by the amount of papers that are loaded for that topic. The difference between significant and insignificant loading was determined using a heuristic empirical tail distribution technique. The cutoff for the five-topic solution was determined to be 0.789 after certain calculations were run using the tail distribution. Consequently, the papers were deemed relevant for the issue if their loading values were above the given level. The coherence score of 0.789 was assessed using standard topic coherence metrics to validate the quality and relevance of the topics generated through Latent Semantic Analysis (LSA). Specifically, topic coherence evaluates how semantically similar the words within a topic are, ensuring that the topics make sense as cohesive units. For this study, coherence validation was performed by calculating word pairwise similarity within each topic, using Term Frequency-Inverse Document Frequency (TF-IDF) weights to highlight the significance of terms in the corpus. A coherence score closer to 1 indicates higher topic relevance, and 0.789 suggests a good level of semantic coherence, meaning the identified topics are well-integrated and relevant for interpreting federated learning trends in healthcare.
3.6. Topic Labeling
To begin labeling topics based on heavily loaded words in term loading, the authors have sorted the loading values from the document loading matrix and term loading in decreasing order. Then, the authors have used an iterative strategy. The values with the highest loading are categorized according to how often they appear and how much weight they carry when doing topic labeling. Due of the substantial variation in topical coherence, the topic labeling was done manually and is therefore susceptible to human bias.
4. DISCUSSION
The research questions in this study were selected based on a systematic approach that addressed theoretical gaps in the literature and practical challenges within federated learning (FL) in healthcare. First, the study aimed to fill existing literature gaps, particularly in privacy-preserving data management and FL integration into healthcare, leading to Research Question 1 (RQ1) on identifying applicable types of FL in healthcare. Second, the study sought to investigate how FL meets specific healthcare needs, including applications in diagnostics, patient monitoring, and medical education, which informed Research Question 2 (RQ2) on FL’s healthcare applications. Finally, recognizing the technical, privacy, and computational challenges that hinder FL’s broader adoption in healthcare, Research Question 3 (RQ3) was crafted to examine these challenges and potential future directions. These research questions thus emerged from a thorough review of relevant literature, focusing on FL’s role in addressing data privacy concerns and operational demands in healthcare, providing meaningful contributions to both academic research and practical implementations.
4.1. RQ1: What are the Different Types of Federated Learning?
Federated Learning (FL) is a sophisticated machine learning technique used to tackle and overcome concerns pertaining to the confidentiality of data. The data is kept secret and isn't transmitted to the server. Only the latest modifications are sent out to the server [14]. The system operates in a decentralized manner, ensuring data confidentiality by training models on the customers' premises using their own local data. Subsequently, it transmits model changes to a centralized server for the purpose of consolidation and integrates an incentive system. In the field of FL, several hospitals function as clients, while a centralized server is located at a certain location. The server uses a proprietary model that is trained using medical data. The learned model is disseminated to various clients, each possessing its own distinct local data. Consumers use their regional data to train the foundational model [15]. In addition, the locally trained models are transmitted to the server one after another for aggregate without transmitting the clients' local data. The server is tasked with aggregating the locally acquired models using several aggregation techniques, such as FedAVG, FedPxN, and others. The updated global model is regularly sent to customers to boost performance, after the calculation of the new averaged value. During the whole process, there is no exchange of local data between clients or with the server, hence increasing the potential of federated learning to improve data privacy [16]. The FL system is capable of processing non-identically distributed (non-IID) data from clients that have different data distributions. It has attributes that make it capable of processing real-time, non-independent and identically distributed (non-IID), widely scattered, and imbalanced data, all while guaranteeing data privacy and reducing connection requirements. FL develops a strong and effective quality model via the use of a decentralized network approach, as described by Mehta et al. (2023). Data in the federated learning setting is distributed over several nodes, including, health applications, hospitals, and health wearable devices. This data is often saved as a feature matrix [17]. The feature matrix presents data instances of clients on the horizontal axis and their accompanying characteristics along the vertical line throughout the FL network. FL may be categorized into three groups based on their data division methods [18]. Fig. (5) illustrates many types of FL and their corresponding methods of distributing data.
4.1.1. Horizontal Federated Learning (HFL)
Each client of HFL has its own dataset specific to the location under consideration. These datasets include distinct properties but with varying instances, as seen in Fig. (6). This method is used when a small sample of data does not adequately represent the whole range of changes in sample size [19]. HFL is a collaborative machine learning strategy that involves numerous hospitals in modeling populations by directly combining data, without the need to disclose individual information. Initially, each technology provider undergoes the process of training a model using its own dataset in order to guarantee the confidentiality of the data [20]. The regional models that have been trained are then sent to a central system known as the Aggregate Server. A server consolidates the distinct models from several hospitals and endeavors to include the distinctive attributes and patterns identified from each dataset. The resultant global model is more resilient and may be used to predict lung cancer statistics over a wider geographical region. Once the process of merging the data is complete, the merged dataset is then uploaded to the updated global model [21]. Through the use of a server, all hospitals that are using the latest worldwide model will get the updated material. All hospitals in the vicinity will promptly adopt the latest iteration of the model as soon as it is acquired by any of their counterparts. Moreover, we ultimately reached the federated learning cycle. By including numerous data sources in the model and regularly updating the data, the entire model is continuously improved with each new iteration [22]. This system lacks the capability for centralized and direct exchanges of data, making it the optimal choice for instances when data secrecy is crucial. The graphic effectively illustrates the efficiency and effectiveness of the distributed learning technique, in which the server orchestrates several local models to ensure they both contribute to and benefit from the global model [15].
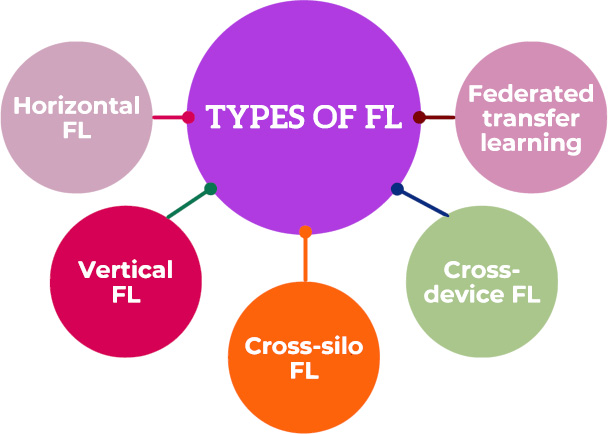
Types of FL.
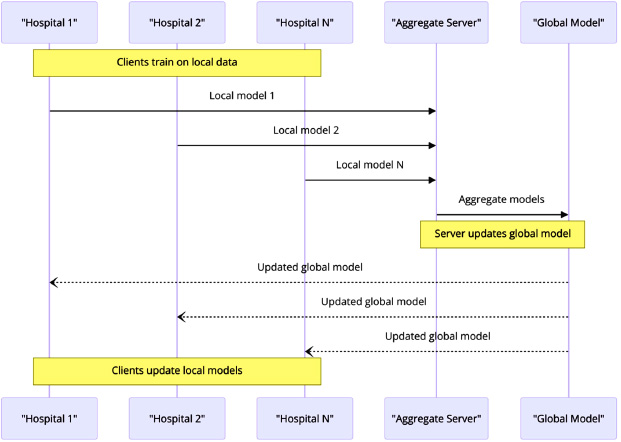
Horizontal federated learning.
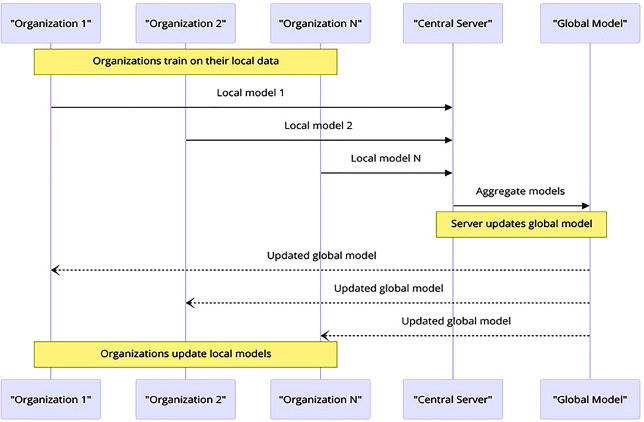
Vertical federated learning.
4.1.2. Vertical Federated Learning (VFL)
Each client in VFL has a distinct local dataset with identical instances but varying feature spaces, as seen in Fig. (7). The nodes in the illustration depict hospitals and wearable health devices that have overlapping patients with different characteristics [23]. In this model, Data Owner A and Data Owner B have different traits for the same piece of data. Their data is not instantly shared. The learning process is coordinated by a Coordinator Server, which they connect to. The Coordinator Server needs to hear from data owners about their features first. Data privacy is often ensured during transport by using secure protocols [24]. All of the characteristics that were received are combined by the Coordinator Server to give you a full picture of the data. In order to create a more accurate and robust machine learning model, this integrated model takes use of the combined traits of all data owners rather than just one [25]. After the model is constructed, the Coordinator Server notifies all data owners of the modifications. Incorporating insights obtained from the combined dataset into their local models, Data Owners A and B may make use of these improvements [26].
4.1.3. Cross-silo Federated Learning (CSFL)
A novel method called cross-Silo federated learning has been developed for collaborative machine learning. Unlike previous approaches, this method enables different industry silos and organizations to collectively train models without revealing their raw data. This approach effectively addresses privacy and security concerns [27]. Each organization, ranging from organization 1 to organization N, trains a local model using its own dataset, as seen in Fig. (8). The communication procedure begins securely, as shown by the successful transfer of locally stored models to the central server. The central server consolidates the national networks and aggregates all models into a global model by using the shared knowledge and insights derived from the datasets of participating organizations [28]. The uncle persistently provided support for every endeavor, even the most recent one after the overall accumulation. By thoroughly reviewing all data recording procedures, ensuring privacy and minimizing any potential loss, the accuracy and robustness of any model will significantly improve across all datasets. CSFL plays a crucial role in enhancing data security and protection by adopting a collaborative approach with other entities, such as cross-Silo learning [29].
4.1.4. Cross-device Federated Learning (CDFL)
The cross-device federated learning technique is a decentralized approach to machine learning. It involves training models across several devices, including smartphones, tablets, and IoT devices, without centralizing the data in a single location [30], as shown in Fig. (9). Cross-device fede-
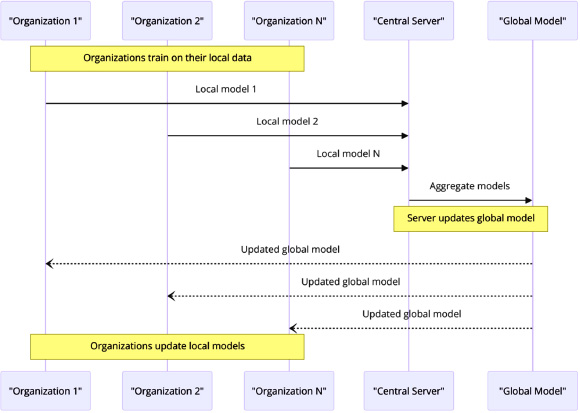
Cross-silo federated learning.
rated learning refers to the practice of training machine learning models on several devices. In contrast to cross-silo federated learning, which involves a large number of end-users, cross-device federated learning typically involves a smaller number of devices, potentially reaching millions. Each device actively participates in the learning process by using its own data to assess and improve the required model [31]. When the merges are sent, either to a central server or disseminated to machines, they are used to incorporate fresh information into the overall model. Subsequently, all the remaining appliances follow the path of refinement. The primary objective of the global model is to augment the efficiency of local models. This is accomplished by moving the local models to a central server for the purpose of sharing. Subsequently, the local model undergoes an update by including the latest global model received from the server [32]. This approach enhances the overall model by including different data from several devices, hence increasing the accuracy and resilience of the global model while ensuring the privacy of individual device data [33].
4.1.5. Federated Transfer Learning (FTL)
By combining federated learning (FL) with transfer learning (TL), a unique method called federated transfer learning may handle cases when many parties wish to train a machine learning model together, but their datasets have different feature spaces [34]. When direct data transmission is not an option or not permitted due to privacy, regulatory, or practical concerns, this approach comes in handy. As shown in Fig. (10), a centralized server facilitates the process of FTL [35], whereby information from one domain is transmitted to another in order to enhance the model in a target domain. Sending a model from the source domain to the server initiates the process, which then sends pertinent information to the destination domain [36]. The receiving domain then asks the server to revise its model based on this new information. The server then updates the target model and sends it out, making it more effective and able to learn from the source domain [37].
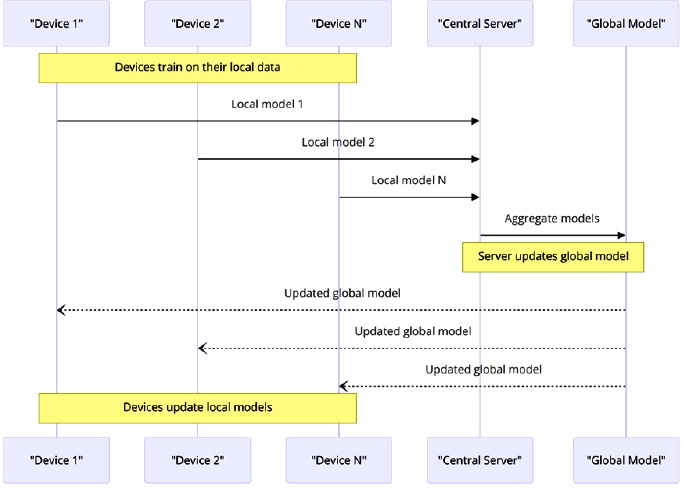
Cross-device federated learning.
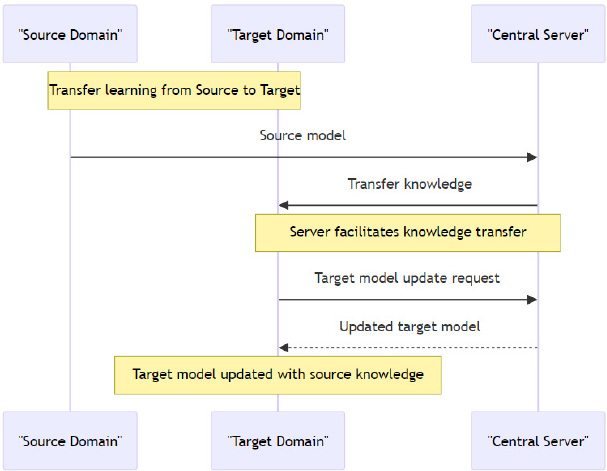
Federated transfer learning.
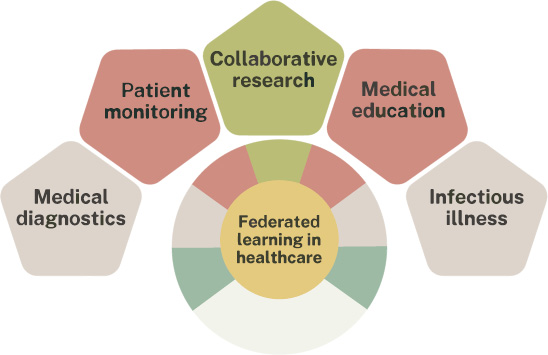
Application of federated learning in healthcare.
4.2. RQ2: What are the Different Healthcare Application Areas of Federated Learning?
The study covers a wide range of applications related to FL-enabled healthcare Metaverse. The investigated healthcare applications include medical diagnostics, medical education, collaborative research, patient monitoring, infectious illnesses or pandemics, and pharmaceutical manufacturing [38]. An exhaustive assessment of the possible applications of FL-enabled healthcare Metaverse may be conducted by taking into account factors such as privacy, security, scalability, and durability. Fig. (11) depicts the many uses of FL-enabled healthcare Metaverse and the accompanying assessment criteria.
4.2.1. Medical Diagnostics
Medical diagnosis involves recognizing patients' illnesses by analyzing their symptoms [37]. Disease diagnosis is traditionally conducted in person at health facilities or hospitals, requiring the patient to undergo a physical examination by a clinician. Conventional diagnostic methods during crises might lead to delayed treatment and potential loss of life. Thus, telemedicine was implemented as a method to remotely monitor patients [39]. The fast advancements in AI have impacted other fields, such as healthcare. ML models may improve illness diagnosis by replicating human cognitive skills and being trained on data from different health institutions [40]. One disadvantage of telemedicine is that patients are unable to effectively communicate their symptoms or the severity of their injuries since they do not have face-to-face interactions with healthcare experts. The Metaverse is an advantageous solution that utilizes sophisticated technologies like AR, VR, digital twins, and blockchain to construct a digital replica of the real world [41]. Medical professionals may provide personalized diagnoses by using the Metaverse, which enables doctors and patients to interact in shared, long-lasting, and immersive virtual worlds. Machine learning models may use data collected from wearable devices and other systems inside the Metaverse environment for model training [42]. Nevertheless, this requires the full availability of data in the system for the whole training procedure. Moreover, as the data collected may include sensitive patient information, any illegal release of this data might result in significant consequences. The Metaverse environment utilizes real-world data to create digital representations of patients via the utilization of digital twins [43]. Groups of patients, individual individuals, or particular organs may be replicated digitally. Modifying digital twins enables the acquisition of accurate insights throughout the decision-making process. Data may be gathered from several sources, including AR/VR devices and wearables. Should the data get into the hands of malevolent persons, they might possibly use it to fabricate inaccurate diagnoses and inappropriate treatments. Hence, it is essential to conduct the model training process in a manner that guarantees the confidentiality of the patient's sensitive data [44].
4.2.2. Collaborative Research
The impact of Metaverse on collaborative research represents a transformative revolution inside an already revolutionary domain. With the ability to have laboratories situated internationally instead of in the same place, it would then be feasible to make data-driven judgments about all chemical supply chains [45]. Integrating a digital parallel model, also known as digital twinning, might potentially enhance the advancement of collaborative project design throughout the first phases of process and control system engineering design [46]. Utilizing advanced simulators and modeling machines is essential for teams from various locations on different planets to collaborate on shared tasks and develop stable and adaptable models that can be integrated into complex systems. Based on this, it can be said that this technique allows for the use of real-time dynamic simulations to support the implementation of process engineering [47]. Another concern arises from the divergent interests of various parties related to the government, which might lead to aggressive activities. Metaverse’s enable comprehensive study across all conceivable domains; nonetheless, they also encounter specific challenges. The FL may be optimally used to establish a collectively safeguarded condition since all the entities within an organization are actively involved and interested in security. The formation of smaller-scale coalitions of cooperation is offered in a study [2]. In this scenario, each client functions at a team level and has the ability to collectively determine which team members contribute the most to the advancement of the model or who are the least efficient in their efforts to enhance it. Federated learning technology enables the collaboration of various institutions to safeguard data privacy. In the field of Model-learning, the manager closely monitors all publicly accessible data. The entities participating in creating the model are not allowed to share data with each other at the same time. The data trainers are educated to prepare the model in a manner where each data owner is allocated a share of the training data, which is then consolidated [48].
4.2.3. Patient Monitoring
The Metaverse has revolutionized the methods by which remote patient care and monitoring are carried out. By using blockchain, augmented reality (AR), virtual reality (VR), mixed reality (MR), and decentralized applications, solutions may be found for the difficulties involved in developing and enhancing the Metaverse [39]. The Metaverse would enable continuous monitoring of patients in real-time to assure active human participation and replication of in-person experiences. Immersive 3-D interfaces are used for remote patient monitoring, enabling synchronized and real-time digital communication between patients and healthcare professionals, resulting in a rich and lifelike user experience [49]. Telemedicine is a component of healthcare that facilitates the delivery of medical services remotely. The need for telemedicine has been greatly recognized with the rise of COVID-19 [50]. Before 2020, 43% of healthcare institutions offered remote therapy to patients. Currently, 95% of healthcare facilities provide remote treatment. Metaverse technology has eliminated the need for regular in-person medical consultations, decreasing the strain on physicians and nurses and enabling them to address minor health issues from a distance [51]. Telemedicine consultations using VR allow for interaction with consultants and professionals from remote locations worldwide using basic headsets. The MRI and scans may be conducted at a nearby facility and the data can be sent to a medical professional situated anywhere globally [52]. Such facilities are very advantageous in nations experiencing severe shortages of medical staff. Patients from rural and isolated areas do not have to travel long distances to get medical services. It is essential to highlight the significance that the Digital Twin plays in this context. In this context, test dummies may be used to get an understanding of how a patient would be recuperating from surgery or how a postoperative patient would respond to a certain drug [53]. Additionally, Metaverse makes it possible for patients and healthcare providers to make use of medical wearables. These wearables communicate with emergency personnel and caregivers in order to notify them in the event of exceptional occurrences such as seizures, flare-ups of chronic obstructive pulmonary disease, or any other healthcare anomalies that may occur for patients who are located in remote locations [54]. For the purpose of accelerating and immediately disseminating therapy using artificial intelligence, the wearables make it possible for clinicians to get data of high quality and to draw the required conclusions and forecasts. The potential of doing daily check-ups in the Metaverse is unrivaled and may be used to facilitate crucial decision-making. Utilizing modern technical approaches for remotely provided mental healthcare in the Metaverse offers several benefits, enabling patients to enhance their capacity to confront fear-inducing events and reduce suicidal tendencies. The Metaverse facilitates health experts in remotely identifying occurrences that are far from their patients via the use of Virtual Reality and Augmented Reality. It also informs family members about situations that may elicit anxiety, anger, or psychosis [55].
4.2.4. Medical Education
The advent of virtual reality (VR) and other technologies that are connected to it has led to the enthusiastic adoption of digital transformation in a variety of fields, particularly in collaborative research [56]. In addition, virtual reality has been able to quickly expand in tandem with the development of technologies for the metaverse. Because of the rising acceptance of these technologies, the related costs of implementation have decreased, and the technology has become more accessible to users who are more prevalent. Through the use of hands-on experimentation, researchers often discover that it is difficult to acquire and remember knowledge. Experimentation and research are fundamental components of higher education programs [57]. There is also a lack of legitimacy in the justification of the experimental findings, which is related to the same cause. Providing the required experimental and hands-on learning experience, the virtual reality-based simulated laboratories are here to help. Students are able to conduct a variety of crucial experiments involving chemicals inside a virtual reality (VR) safe zone, which eliminates the possibility of causing damage to either property or human life [58]. With the elimination of the difficulties associated with the availability of chemical or related resources, it is now possible to conduct tests several times in order to guarantee that the findings are not a coincidence. Moreover, the elimination of risks assures that student minds will be innovative and creative, allowing them to conceive and execute experiments that may result in ground-breaking findings and discoveries, which will contribute tremendously to the development of science. When it comes to the field of medical education, the Metaverse makes it possible for medical professionals to interact with one another with the help of simulation-based training while they are still in the process of obtaining their medical education [59]. In the context of surgical procedures, for instance, the use of the Metaverse must be supplemented by an awareness of the utilization of instruments that ensure the capability of dexterous grasping techniques. Therefore, there is an increasing need for the monitoring of technologies that are being used in order to improve the adaptability and flexibility of the technologies that are employed in the Metaverse. As a training tool, virtual reality (VR) has the potential to show students a full-body model of the human body and a bird's-eye view of a patient's illness. Professionals in the medical field may train their colleagues and other staff members using Metaverse technology [60]. Just like the Internet of Things (IoT), digital twins, 6G networks, robotics, edge computing, cloud computing, and quantum computing have the potential to revolutionize medical diagnosis and treatment, and they can also be used to create VR, AR, and MR systems. Surgery in the Metaverse might benefit from the use of robots since it would allow for more precision and adaptability throughout the operation. The Metaverse's cloud-based and real-time communication technologies allow medical students to practice their talents with the maximum degree of accuracy [53]. This contributes to the guarantee that patients get sufficient healthcare at the right place and time. The Metaverse environment makes use of a wide variety of materials, such as geographical settings, mixed reality, avatars, and 3D models. All of these resources go into making the setting. As the number of students increases, it is expected that data and communication standards will also rapidly expand, facilitating student communication throughout the Metaverse. Maintaining privacy, scalability, and interoperability may be achieved by the use of FL to simplify data flow between various platforms and networks [61]. The deployment of these services, however, requires several institutions to share large amounts of medical imaging data. In order to build data-driven models that may enhance healthcare services, academics and healthcare practitioners utilize this data.
4.2.5. Infectious Illnesses or Pandemics
The COVID-19 pandemic has created the ideal conditions for the technology of the Metaverse to take off and become widely used [62]. The epidemic has had a significant impact on the national economies as well as the social and political stability of the countries. Isolation for longer periods of time has even contributed to the development of social anxiety in a culture [63]. As a result of the pandemic, significant technical improvements have been achieved in the fields of education, healthcare, and entertainment. In addition to schools, companies and medical professionals have also seen the impact of virtual meetings, telemedicine, and online lessons delivered via over-the-top (OTT) services [61]. The monitoring of the pandemic in July is a crucial aspect that reveals the impact on individuals and provides valuable insights into the illness's progress. This information may be utilized to make informed decisions and improve control measures to mitigate the spread of the disease. By developing a platform capable of integrating data from many sectors such as telephony, transport, and commerce, it becomes easier to monitor activities in a transparent and environmentally responsible manner [1]. Nevertheless, even using the most cutting-edge technological advancements might provide challenges in terms of transmitting, delivering, and monitoring medical therapy in the real world. In order to create a digital environment that accurately replicates the real world and has a comprehensive understanding of its functioning, it is vital for the Metaverse to acquire knowledge and accurately simulate real-world responses. The selection of the Metaverse may be influenced by the prevalence of illnesses and the efficacy of existing methods for managing them. Nevertheless, the current data is diverse, making it necessary to effectively use cross-models [64]. Furthermore, ensuring the confidentiality and safeguarding of data is crucial in situations when the exchange of information worldwide is necessary for the development of an international illness prediction and trend assessment model. This model will allow for the implementation of any significant measures that are necessary to prevent the disease from spreading further. Even in the field of healthcare, the Metaverse has shown to be useful in the performance of surgical procedures, the monitoring of patients, and the detection of diseases [40]. FL is a solution that may be used in these kinds of circumstances since it offers more security, improved data administration, and cross-model utilization [42]. In order to identify anomalies in computed tomography (CT) that were associated with COVID-19, the authors [58] used FL. Take, for instance, the COVID-19 pandemic era. During that time period, people from a variety of nations were suffering from different coronavirus strains. Because of this, the therapeutic treatments that are applicable would likewise vary depending on the location of the virus and the kind of virus. Moreover, the kinds of preventative actions that each country need to implement are significantly different from one another. As a result, individualized treatments are essential, particularly during the epidemic era. A global model that any of the participating clients may use for training and testing their own data is created by the FL-enabled healthcare Metaverse, which provides assistance in instances like these. It would be easier to acquire individualized solutions for the end customer if this were done [65]. Under these circumstances, the implementation of FL will not only assist in the protection of data privacy, but it will also assist in addressing issues over scalability in a Metaverse healthcare context. When compared to the conventional way of training the data locally at the edge devices, the strategy was shown to be effective in protecting the confidentiality of patients. Because of this, FL is able to assist in the construction of a global model without requiring the original data to be shared with any centralized Metaverse server. This protects the data from being accessed by any type of nefarious users federated [17].
4.3. RQ3: What are the Key Challenges and Possible Future Directions of Federated Learning in Healthcare Metaverse?
In healthcare, federated learning, which utilizes remote data sources while protecting patient confidentiality, is an innovative approach to constructing machine learning models. By adopting this approach, healthcare organizations can collaborate on AI research and application development, while simultaneously addressing the unique challenges associated with healthcare data and adhering to rigorous privacy regulations.
4.3.1. Restricted Computing Abilities
Challenge: A device's processing power is affected by its data processing, bandwidth, and storage capacity. The healthcare Metaverse's FL gathers information from dispersed healthcare consumers to construct a worldwide mode [4]. FL enables healthcare Metaverse on-device training while simultaneously updating the global model with local model changes, as long as the client's private local data is preserved. With Florida's help, the healthcare VR industry can grow while still keeping patient information safe. Collaboration in disease diagnosis, real-time monitoring of vital signs, personalized treatment suggestions, healthcare resource allocation, and pharmaceutical research and development are all areas where FL improves the healthcare Metaverse. According to [51], FL allows medical professionals to use decentralized data while protecting patient anonymity. As a result, patient care is improved and the Metaverse healthcare ecosystem advances. In the healthcare Metaverse, devices that are connected to the internet, such as surgical robots and inexpensive computers, are used; nevertheless, these devices are designed with limited processing power, bandwidth, and storage capacity. It is difficult to adapt FL for the health Metaverse due to this constraint.
Possible solutions: One possible solution to the problem of limited computer capabilities is edge computing. Metaverse healthcare applications that need low latency and high bandwidth close to the data source may benefit from mobile edge computing. The authors [38] noted that in order to save power and computer resources, healthcare IoT devices might send data to nearby edge servers. Because they are physically located closer to end users, edge servers in the Metaverse are able to provide services with much lower latency than their cloud-based counterparts. Extensive computation and storage capabilities are provided by cloud-native technology that utilizes hierarchical architecture. An appropriate combination of edge and cloud-based applications is required for optimal device performance. Surgical robots and other low-cost medical computing equipment are made possible by the cooperation of edge and cloud-based applications.
4.3.2. Privacy and Security
Challenge: For the healthcare Metaverse to gain confidence, privacy and security must be paramount. FL may ensure the privacy of all participants in the healthcare Metaverse by training the models locally and only sending the model parameters to their server [65]. It is still possible for healthcare Metaverse users to inadvertently provide attackers or intruders access to sensitive data when they share model changes during training. The healthcare Metaverse's FL is thus vulnerable to distributed denial-of-service (DDoS) attacks and jamming, which are communication security issues. One kind of attack is a jamming attempt, in which the perpetrator sends out powerful radio-frequency jamming signals in an effort to disrupt or block the connections between the primary server and the mobile healthcare MR devices [24]. The reliability and efficiency of the FL systems in the healthcare Metaverse may be compromised if this kind of attack leads to mistakes in model uploading and downloading.
Possible solutions: Differential privacy and collaborative training provide potential solutions to address the concern of user data privacy in the healthcare Metaverse. Nevertheless, the use of these methodologies leads to a decrease in performance, specifically in terms of the models' accuracy. They are also quite resource intensive for the healthcare Metaverse devices that are taking part in them. Consequently, the FL system's development must take the tradeoff between privacy protection and system efficiency into serious consideration. By delivering an additional copy of the model update over several frequencies, antigambling methods like frequency hopping may be used to circumvent the security issues indicated in the issue.
4.3.3. Integrating the Real and Virtual Realities
Challenge: It is the goal of the Metaverse to create an exact digital copy of all real-world objects. To make the most of the Metaverse and make any modifications to an item instantly visible in the virtual world is the only way to go. A major obstacle in the healthcare Metaverse is maintaining constant synchronization between the real and virtual worlds [24]. The healthcare Metaverse provided by FL relies on real-time data from a range of devices and sensors to establish a complete global model for patient diagnostics and medication development in the healthcare sector. In order to transmit data from the physical world to the healthcare Metaverse empowered with FL, a dependable network with low latency is necessary. An example of the healthcare Metaverse is the use of telesurgery when a surgeon performs an operation on a patient using robots from a different location [66]. Remote surgery of this kind requires the following elements: the ability to transmit data in real-time, robotic technology that can accurately capture a surgeon's movements, precise coordination among the surgeon's virtual colleagues in the Metaverse to faithfully replicate the surgeon's actions, and the assistance of FL to provide surgical expertise. The widespread adoption of the healthcare Metaverse is severely hindered by any network-related problem, which in turn causes disastrous consequences.
Possible Solutions: The 6G network might be the key to getting the physical and digital worlds in harmony. Sixth Generation wireless networks use the terahertz spectrum, boast a maximum data transfer rate of 1Tbps, and provide very dependable, low-latency communication with a latency of less than 1 ms [67]. In the Metaverse, FL-enabled healthcare provides a far higher quality experience overall because of 6G wireless networks. When 6G's dependable and fast connectivity allows for the seamless merging of the digital and physical realms, it will greatly improve surgeons' ability to do procedures quickly and accurately [68].
4.3.4. Lack of Clarity
Challenge: The models created using FL lack justification and explanation. FL models' opaque nature obstructs consumers from comprehending the model's output. In a complex situation, the black-box model's unproven predictions or conclusions may cause the healthcare Metaverse to collapse. Devices used in medicine that may identify a patient's condition using a visual recognition model are one example. If the model is too complicated for the doctor to interpret, the results can be misleading [14]. Due to the black-box model's potential for faulty interpretation, healthcare workers in the virtual reality healthcare setting may make bad decisions. Expanding the range of healthcare Metaverse evaluations requires the development of an interpretable FL model. The creation of a diagnostic support system in Florida to aid doctors in the diagnosis of complex diseases is one such example. Multiple healthcare Metaverse clients' patient datasets are used to train the FL model. Diagnostic assistance may still be generated in the event of a client data breach that exposes the model's parameters. Following this suggestion, the physician will arrive at an inaccurate diagnosis. Justifiable and explainable proposals are necessary to hold such circumstances responsible [4].
Possible Solutions: In the healthcare Metaverse, creating FL models that are both intelligible and interpretable could be a problem; explainable AI (XAI) might be the solution. In order for those involved in the healthcare Metaverse to comprehend and have faith in the results generated by prediction models, XAI incorporates a number of approaches and strategies. As part of the healthcare Metaverse, doctors have access to interpretable FL models that may help them understand ideas, verify them with their experience, and get meaningful insights into diagnosis [65]. Enhanced transparency and interpretability of black-box models reduce the likelihood of wrong judgments, hence improving the overall efficacy and reliability of the healthcare Metaverse. XAI integrated with FL offers justifiable, explainable, and responsible ideas to physicians, enabling them to make improved individualized recommendations to patients.
4.3.5. Fair FL
Challenge: In the healthcare Metaverse, creating FL models that are both intelligible and interpretable could be a problem; explainable AI (XAI) might be the solutions. Naïve global model optimization may lead to specific devices in this system gaining an unwarranted advantage or disadvantage. Efforts to achieve fairness and equality are essential. Equitable allocation of resources and a fair system of incentives are essential for FL, as stated by [61]. Distributing an adequate amount of computer and communication resources equitably is a significant obstacle in the healthcare Metaverse. For instance, if the model exhibits bias towards certain devices or patient data, it might lead to the provision of erroneous predictions during a disease outbreak such as COVID-19.
Possible Solutions: The implementation of effective incentive mechanisms encourages additional devices to participate in the global model generation effort of the healthcare Metaverse. When gadgets collaborate and provide valuable data, they have the potential to receive more incentives [49]. This enhances the dependability of the model and the learning efficiency of federated learning models. Furthermore, this promotes the development of equitable federated learning models. In the aforementioned scenario, these incentive mechanisms serve to entice a greater number of devices to participate, hence facilitating the establishment of a fair model for managing disease outbreaks such as COVID-19.
CONCLUSION
In this research, FL is comprehensively investigated within the healthcare industry. The study highlights the potential of FL to improve data privacy and integrity while complying with severe laws such as HIPAA. The systematic use of LSA on a dataset of 6,800 research studies following PRISMA guidelines resulted in the identification of a structured five-topic solution with a coherence score of 0.789. The thorough analytical method uncovered the extensive range of FL's uses in healthcare, including telemedicine, diagnostics, and patient monitoring. It also emphasized the significant hurdles related to data privacy, processing requirements, and the incorporation of many data sources. The healthcare Metaverse presents a fresh opportunity for study in FL, emphasizing data security, computational efficiency, and practical implementation of FL technologies. This document highlights Florida's potential to revolutionize patient care by securely processing data in a decentralized manner. It also outlines a future-oriented plan for innovation and research at the crossroads of Florida and healthcare technologies, with the goal of overcoming current limitations and maximizing the benefits of Florida in enhancing healthcare results. The study’s limitations include reliance on a dataset from major academic databases, potentially omitting niche studies, and the use of LSA, which, while effective, may not capture subtle semantic nuances as newer models might. The coherence score (0.789) indicates room for better thematic alignment, and manual topic labeling risks human bias. The theoretical focus, without real-time data, limits practical applicability in live healthcare settings. High computational demands also challenge scalability, and the study does not fully address the diverse regulatory and resource constraints across healthcare systems, signaling areas for future research to improve generalizability.
AUTHORS’ CONTRIBUTION
R.: Methodology, Review; V.K.: Methodology, Review and editing, Supervision; S. H: Integration, Validation; A.D.: Analysis, Visualization; B.G.: Integration, Visualization
LIST OF ABBREVIATIONS
LSA | = Latent Semantic Analysis |
FL | = Federated learning |
DL | = Deep Learning |
ML | = Machine Learning |
AI | = Artificial Intelligence |
HIPAA | = Health Insurance Portability and Accountability Act |
PRISMA | = Preferred Reporting Items for Systematic Review and Meta-analyses |
TF | = Term Frequency |
IDF | = Inverse Document Frequency |
SVD | = Singular Value Decomposition |
NLTK | = Natural Language Toolkit |
ID | = Integer Identity |
Non-IID | = Non-identically Distributed |
HFL | = Horizontal Federated Learning |
VFL | = Vertical Federated Learning |
CSFL | = Cross-silo Federated Learning |
CDFL | = Cross-device Federated Learning |
TL | = Transfer Learning |
FTL | = Federated Transfer Learning |
AR | = Augmented Reality |
VR | = Virtual Reality |
MR | = Mixed Reality |
OTT | = Over-The-Top |
CT | = Computed Tomography |
DDoS | = Distributed Denial-Of-Service |
XAI | = Explainable AI |
IoT | = Internet of Things |
AVAILABILITY OF DATA AND MATERIALS
The data supporting the findings of the article is derived from an extensive corpus of 6,800 research articles collected from the following academic databases and repositories: Google Scholar, Mendeley, ACM Digital Library, Taylor & Francis, IEEE Xplore, and Scopus. These materials were systematically selected following the PRISMA guidelines to ensure high-quality and relevant studies. The findings were generated using Latent Semantic Analysis (LSA), which processed the abstracts and titles of the collected articles to identify trends in federated learning research in healthcare. Since the study is based on secondary sources (peer-reviewed articles), no new datasets were generated. However, detailed search strings and inclusion/exclusion criteria for selecting articles are available within the manuscript for reproducibility.
ACKNOWLEDGEMENTS
Declared none.