Putamen Activation Represents an Intrinsic Positive Prediction Error Signal for Visual Search in Repeated Configurations
Abstract
We investigated fMRI responses to visual search targets appearing at locations that were predicted by the search context. Based on previous work in visual category learning we expected an intrinsic reward prediction error signal in the putamen whenever the target appeared at a location that was predicted with some degree of uncertainty. Comparing target appearance at locations predicted with 50% probability to either locations predicted with 100% probability or unpredicted locations, increased activation was observed in left posterior putamen and adjacent left posterior insula. Thus, our hypothesis of an intrinsic prediction error-like signal was confirmed. This extends the observation of intrinsic prediction error-like signals, driven by intrinsic rather than extrinsic reward, to memory-driven visual search.
INTRODUCTION
Because of its anatomical connectivity with premotor and somatosensory cortices, the putamen has mainly been regarded as part of a motor feedback loop [1, 2]. It appears to be involved in the representation of novel versus learnt motor sequences [3]. However, putamen activation also varies with feedback across a wide range of tasks. Increased putamen activation for positive feedback was observed in a range of different paradigms, e.g., motion prediction [4], time estimation [5], arbitrary associative learning [6, 7], delayed matching [8], sequential rule learning [9] probabilistic learning [10] and probabilistic reversal learning tasks [11].
A specific role of the putamen, along with the caudate, in error detection was described early on [12-14]. The putamen receives fibers from the rostral cingulate zone [15], which has been shown to support error processing [16] negative reward probability [17] and internally selected actions [18]. Putamen activation consistently mirrored reward prediction error responses in a recent metaanalysis of human neuroimaging studies, both for Pavlovian and instrumental conditioning [19]. A number of studies using the information-integration category-learning paradigm have investigated the nature of feedback that leads to putamen activation. In one study, putamen activation was found with either external feedback or observational learning regimes [20]. Overall, the activation strength of the putamen did not differ depending on feedback. However, left putamen showed a higher activation during the test phase than the learning phase in the observational learning task. There was also a numerical, but non-significant activation increase for the test over the learning phase in the feedback task. Likewise, posterior putamen activation was increased for successful versus unsuccessful categorization (i.e. after learning) in another information-integration category learning study with either monetary reward or informative (correct/incorrect) feedback [21]. In an observational learning version of the same task, activity in the posterior putamen could be modeled as an internally generated prediction error signal based on subjective confidence ratings [22]. Activation increased when the participant's confidence rating was higher than expected from his / her learning history and it decreased vice versa. Converging evidence comes from a perceptual learning study, in which a model-based analysis of the BOLD response yielded evidence for a confidence-based prediction error supported by the bilateral ventral striatum, with peaks in the left anterior putamen and just medial of the right anterior putamen [23]. Thus, in a whole range of studies, activation of the (particularly left) putamen increased when the participant was successful and the success evaluation did not necessarily depend on the presence of external feedback. In one study, the role of putamen could be further specified in that activation increased in the form of a prediction error, i.e. only when the success in solving the task was higher than expected [22]. Moreover, the latter activation pattern in the putamen occurred in the absence of external feedback but could instead be related to subjective confidence.
In order to investigate these findings further, we investigated if a prediction error-like response can be observed during visual search in repeated displays. If the interpretation of putamen activation representing an internally generated prediction error is correct, we would expect that the activation increases when a reward is given whose occurrence is uncertain, compared with a certain reward. In a classical conditioning task, a positive prediction error would occur when an unexpected reward is given, whereas a reward following a 100% reward-predictive conditioned stimulus would not elicit a prediction error [24]. Following the early studies on reward learning with primary reinforcers (see e.g [25] for a review) it has been found that reward can take many forms, from primary reinforcers via secondary reinforcers such as money to positive feedback (“correct”) and even to an internal increase in confidence (see [26] for a recent review).
In the present study, we investigated if the occurrence of a target in a visual search display would elicit an increase of activation if the target's location is predicted by a previously learnt spatial context. Importantly, we varied the probability that the target will appear at the previously learnt location given the repeated context to be p=0.5 or p=1. If the putamen activation reflects an internal prediction error, it should be increased if the target appears at the learnt (versus the changed) location in the p=0.5 probability displays. No prediction error signal was expected for the displays that predict the target location with certainty.
METHODS
Participants
We initially recruited 23 participants from the Otto-von-Guericke-University community. All of them were right-handed and reported normal or corrected to normal vision. Prior to participating, each participant was informed about the task and risks. The experiment followed the tenets of the Declaration of Helsinki (World Medical Association, 2013). The study was approved by the local ethics review board: Ethikkommission der Medizinischen Fakultät der Otto-von-Guericke-Universität Magdeburg. The participants signed a written consent form. Each participant received 24 Euro. One participant did not finish the fMRI session, feeling unwell. Another participant did not follow instructions. Three additional participants were excluded due to reporting to have employed search strategies (One subject searched line by line always looking from left to right like in reading, another one always searched clockwise and the third searched line by line always from bottom to top of a display) because search strategies may prevent contextual cueing [27]. Of the remaining 18 participants (age: mean=25.7, sd=3.93, range=21-33), eight were female.
Stimuli and Procedures Learning Session
Participants carried out a variant of the contextual cueing task [28]. The stimuli were black T's and L's presented on a gray background. Each display consisted of one target stimulus (T rotated 90° or 270°) and 11 distractors (L rotated 0°, 90°, 180° or 360°). The distractor L's had a line offset of 4 pixels to make them more similar to the T, thereby increasing search difficulty [29]. Configurations were created using 4 imaginary concentric circles with 4, 12, 20 and 28 positions respectively. The target location was always drawn from the largest and second-largest circle. The 24 target locations were never used for distractor presentation. Each distractor location was drawn randomly from one of the circles with the restriction that there had to be an equal number of stimuli in each quadrant. So there were 3 distractors in all quadrants but the target quadrant that contained only 2 distractors. The size of each stimulus was 1.25° by 1.25°.
The learning session consisted of 16 blocks with 24 trials each. Within each block 16 old and 8 new displays were presented. The old displays were identical for all participants; for the new displays the target positions remained identical across participants, but the exact stimulus configuration varied. A display was regarded as “old” when all stimuli were presented in the same configuration and the rotation of the distractors was identical. Due to a coding error, target locations used for displays that were destined to remain old throughout both sessions without any change in target location were always drawn from the outer circle. For the other conditions, half of the target locations were in the outer circle and half in the 3rd circle. The target rotation (90° or 270°) was randomly assigned over trials with 12 90° rotations and 12 270° rotations per block. Each of the 16 old configurations was repeated once per block. Target locations were evenly distributed across quadrants. New displays consisted of one of 8 fixed target positions, the distractor stimuli were presented at randomly drawn locations. The task of the participants was to search for the T and to indicate in which direction the T was rotated. For a T where the horizontal bar was left of the vertical bar, they had to press the left key with the index finger and vice versa the right key with the middle finger of their right hand. In the learning session, the left and right arrow keys on a standard keyboard were used for responding. Before starting the experiment, participants were asked to answer as fast and as accurate as possible and to avoid fixed search strategies but instead to “trust their gut feeling” to find the T [27].
The learning session took place in a soundproof chamber. Stimuli were presented on an LCD monitor (refresh rate: 60 Hz, resolution: 1920 x 1200 pixels). The experimental code for learning session and scanning session was written in Matlab and presented using Matlab and the Psychtoolbox for Matlab [30]. The viewing distance of 60 cm was kept constant with the help of a chin rest. Each trial started with the presentation of a fixation cross for one second. Right after the fixation cross the display was presented until the participant responded. If the response was wrong, the participant heard a low tone for half a second in which the display maintained visible. Immediately after the correct response or after offset of the feedback tone, a new trial started (Fig. 1a). After each block, participants could take a short break before continuing the experiment. During the break they received feedback regarding their average search time in the previous block. After the experiment, participants completed a questionnaire containing demographic questions as well as general questions about the experiment, e.g. on perceived difficulty and strategy use.
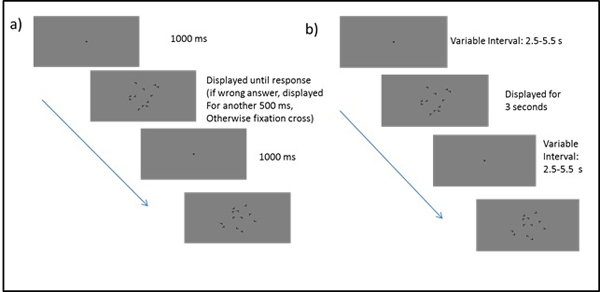
Stimuli and Procedures Scanning Session
All participants performed 2 sessions on 2 separate days. Between the sessions there was at least one day but no more than 7 days. In the 2nd session, the scanning session, stimuli were identical to stimuli in learning session except for size (0.83° by 0.83°).
The scanning session consisted of 16 blocks, which were divided into 4 runs. Each run took 11 minutes and 40 seconds to be completed. The first run of the fMRI session contained the same 16 old displays as in the learning session along with 8 new displays per block whose target positions were the same as in the learning session. Thus, the first run was to establish that the display repetions in the learning session led to a search advantage for repeated displays. From run 2 to 4 of the scanning session, 4 of the 16 old displays were kept unchanged throughout the experiment as a 100% predictable control condition (Context old, Target fixed condition: CoTf). Target positions for these displays were all located in the outer circle. In the remaining 12 displays with repeated distractor configurations, the targets were presented at a novel position in every other block, leading to a target predictability of 50%. When an old display contained a changed target position (Context old, Target changed: CoTc) in the previous block, this display was presented with the learnt target position in the next block (Context old, Target variable, but unchanged: CoTv). The target was in a novel position for 6 old configurations in each block. For an overview of all conditions see Fig. (2). The same task as during learning session was carried out. Participants used a response box consisting of only two keys for answering. Again, participants were asked to answer as fast and as accurate as possible and to avoid fixed search strategies [27].
To enable the use of hyperalignment [31], the first run contained the same display sequence for all participants. These analyses are not reported here. From run 2 on, display presentation sequence and selection of changed displays were individually randomized.
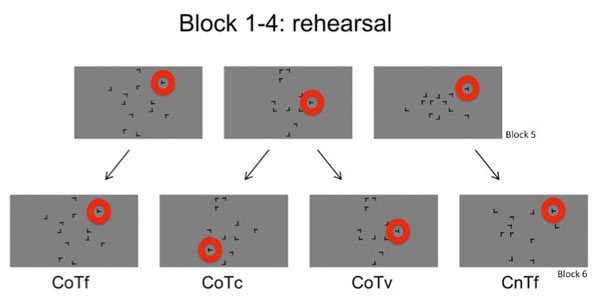
The main experiment was followed by an explicit recognition task. The purpose of this task was to check if the incidental learning task led to implicit or, rather, explicit learning. Specifically, we expected learning to be implicit. As previous findings of intrinsic prediction error signals were obtained in explicit memory tasks (22, 23), an intrinsic prediction error signal in the presence of implicit learning would be a novel finding. The recognition task began with the question “Do you think some of the displays were repeated?” (in German). If participants answered “No” they were informed that some of the displays were repeated. If they answered “Yes”, two other questions were asked to find out from what moment on participants realized that displays were repeated (question 2) and if they tried to remember the old displays (question 3). Following these questions, participants were introduced to the recognition task. This task consisted of presenting the 16 old displays and 8 new displays (randomly generated in each block) once per block for a total of 4 blocks. In these displays, the target was replaced with an additional distractor and participants were asked to indicate via a mouse click which distractor might have been the target. If they were not sure, they were asked to guess. The x and y coordinates of the mouse clicks were recorded.
The second session was run within the MRI scanner. Stimuli were presented using a DLP-projector (resolution: 1280 x 1024, refresh rate: 60Hz), and a rear projection screen positioned behind the head in the bore of the magnet that was visible for participants via a mirror mounted on the head coil.
As in the learning session, each block started with a fixation cross (Fig. 1b). The fixation cross was presented for a variable length between 2.5 and 5.5 seconds followed by display presentation for a fixed duration of 3 seconds. If no response was elicited within these 3 seconds, the result was coded as a miss. The total length of each trial thus varied between 5.5 and 8.5 seconds with a mean trial duration of 7 seconds. At the end of each epoch, the average search time for the last epoch was presented on the screen.
At the end of the session, participants were asked to complete the recognition test outside of the scanner. Stimuli were presented on an LCD monitor (resolution: 1280 x 1024, refresh rate: 60Hz). There was a total of 4 blocks of 24 trials. The trial always started with a fixation cross presented for 1 s. Then the display was shown until the participant responded. After the response the display stayed on screen for another 500 ms until the new fixation cross appeared. After completing the recognition test, participants were asked to fill out the same questionnaire as after the learning session.
FMRI Image Acquisition and Processing
FMRI data was acquired using a Siemens MAGNETOM Trio whole body 3T magnetic resonance scanner equipped with an 8-channel head coil. Using a T1-weighted magnetization-prepared rapid acquisition gradient echo sequence (MP-RAGE) structural images were recorded at isotropic voxel size of 1mm (Field of View (FOV) = 256mm, matrix size = 256 x 256, 192 sagittal slices, TR = 2500ms). Functional images of the whole brain were acquired using a T2*-weighted echo planar imaging (EPI) sequence (TE = 30ms, TR = 2000ms, slice thickness = 3mm, slice gap thickness = 0.3 mm, FOV = 192mm, matrix size = 64 x 64, flip angle = 80°) in 4 runs of 350 volumes each. The 34 slices were oriented parallel to the anterior-posterior commissural plane.
MRI data processing was done using FSL 5 [33]. Using the BET procedure in FSL [34], brain extraction of the high-resolution anatomical image was performed. Head motion correction was performed using the MCFLIRT routine [35] in FSL. Spatial smoothing of functional data was achieved by applying a Gaussian kernel with a FWHM of 6mm. A high-pass filter with a cutoff of 80s was applied to the time series. Normalization was accomplished through a 2-step registration procedure. First the functional image was aligned to a high-resolution structural image by a 12-degree-of freedom co-registration, and then this image was standardized to MNI space by a 12-degree-of-freedom co-registration. Contrast maps were standardized using the nonlinear FNIRT routine [36] in FSL.
Data Analysis: Behavior
Analysis of behavioral data was conducted in R [37] using standard packages as well as the additional “ez” package [38]. Prior to analyzing the data, blocks were aggregated to epochs of 4 blocks. Also all trials with reaction times lower than 200ms or higher than 3 standard deviations above the individual mean for each epoch were discarded (learning session: 2.60%, fMRI session: 1.90%). Only correct trials were analyzed (discarded due to error: learning session: 1.75%, fMRI session: 2.02%).
For the recognition task, the Euclidean distance between the previous target location of a display and the mouse click position was calculated. If the click occurred within 10 pixels of the target center, the response was considered correct and the Euclidean distance was set to zero. A paired sample t-test was conducted on the Euclidean distance of old and new displays.
Data Analysis: FMRI
The fMRI data analysis was performed on prewhitened data of run 2 to 4 of the fMRI session. Using the tools of FSL 5 [32] a GLM with 4 regressors of interest (CoTf, CoTv, CoTc, CnTf) was calculated. Regressors were defined via events that lasted from the onset of the display until response, thereby taking into account search time variability in the model and increasing the power [33]. Error trials and head motion parameters were modeled as regressors of no interest. For all regressors the BOLD response was modeled by a canonical double gamma function and its temporal derivative. For higher-level analyses the mixed effects FLAME I routine was used. For thresholding the default settings of FSL Feat were used (cluster forming threshold: z=2.3)
RESULTS
Pretest Session
Behavioral Data
Search Times
A repeated measures ANOVA calculated across epochs 2 to 4 with the factors configuration (old and new) and epoch (2 to 4) showed a significant effect of epoch (F(34,2)=24.46, p<0.05, η2=0.59). The effect of configuration narrowly failed to reach significance (F(17,1)=4.00, p=0.06, η2=0.19). The interaction of both factors was not significant (F(34,2)=0.02, p=0.99).
Error Rates
Overall error rates were low (mean: 1.75%, range: 0-6.77%). There was only a significant effect of epoch (F(51,3)=4.53, p<0.05, all other F<1.6, p>0.21).
FMRI Session
Behavioral Data
Search Times
(Fig. 3) shows the mean response times for Epochs 1-4. In block 1 of the scanner session, no significant difference between CoTv (1288.9 ms) and CoTf (1324.3 ms) was observed (t(17)= -1.127, p>0.27.
A repeated measures ANOVA calculated on Epochs (2, 3, 4) and configurations (CoTf, CoTv, and CnTf) yielded a significant main effect of epoch (F(34,2)= 22.51, p<0.05, η2=0.57) and a significant main effect of configuration (F(3,51)=11.60, p<0.05, η2=0.406). The interaction missed significance (F(6,102)= 0.88, p=0.51). Post hoc paired t-Tests corrected with Holm adjustment [39] on Epochs 2-4 for the factor configuration revealed significant differences between the conditions CoTv and CoTc (t(53)=-9.23, p<0.05), CoTv and CnTf (t(53)=-6.45, p<0.05), CoTv and CoTf (t(53)=-3.14, p<0.05) and CoTf and CoTc (t(53)=-2.89, p<0.05).
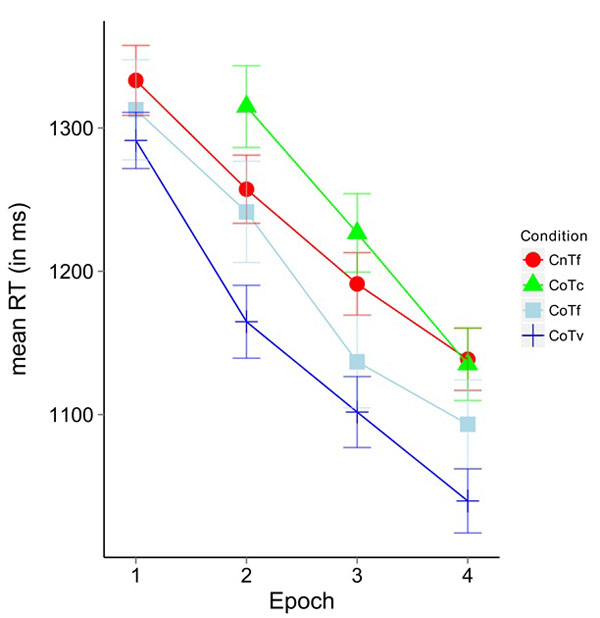
Error Rates
The overall error rate was 4.01% with a range of 0.26%-11.46%. There was a significant effect of epoch (F(3,51)=3.87, p<0.05), all other contrasts were not significant (all F<1.92, all p>0.18).
Recognition Task
We analyzed explicit learning via the Euclidean distance between the actual and indicated target location. A paired t-Test performed on this measure with configuration as independent variable revealed no difference in distance for old and new displays (t(71)=-1.48, p=0.14). Descriptively, the Euclidean distance between predicted and correct target was slightly smaller for old (mean=326.5 pixels) than for new displays (342.5 pixels). However, a paired samples t-test did not reveal a significant difference (t(71)=-1.48, p>0.14). The overall hit rate was low (below 10% exact Value; 10.6% for old displays, 7.1% for new displays).
Imaging Data
Of central interest was the contrast between CoTv and CoTf, because they represented the trials in which the target appeared at the location predicted by the distractor configuration, but with different probability. For a “prediction-error”-like response, we expected higher activation in CoTv, with p=0.5, than in CoTf, with p=1. This was observed in the left hemisphere in the posterior putamen and adjacent claustrum and posterior insula as well as bilaterally in occipital cortex (Fig. 4a, Table 1). No increased activation was observed in the reverse contrast. Fig. (5) shows the activation timecourses for the experimental conditions in the left putamen.
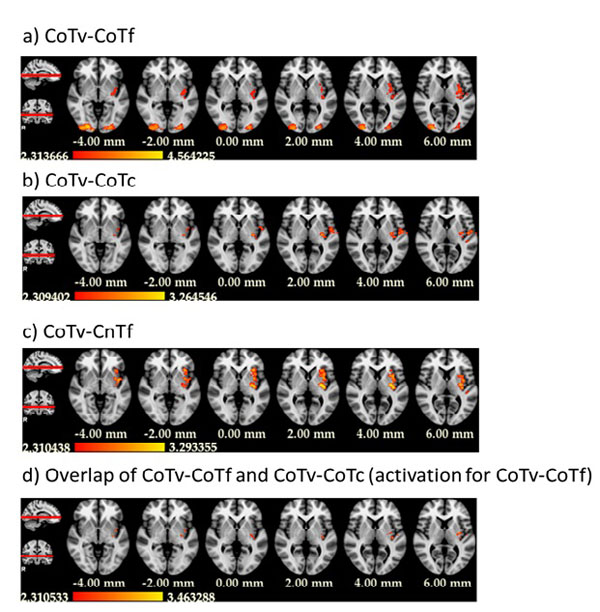
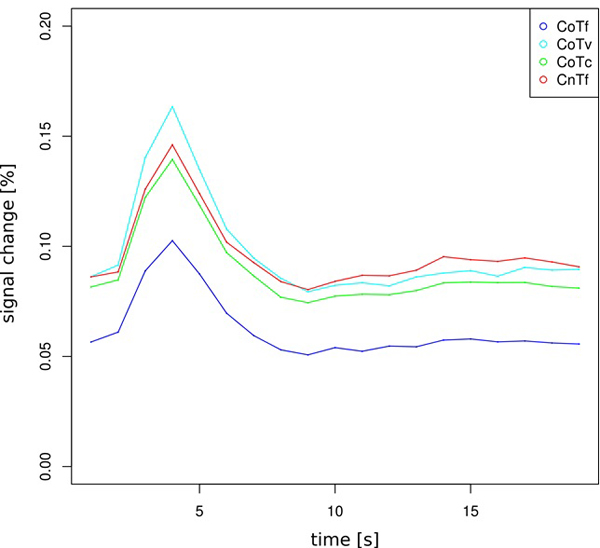
Area | Cluster | Puncorr | Z | x | y | z | BA | Voxels |
---|---|---|---|---|---|---|---|---|
Claustrum | 1 | 2.16 e-4 | 3.52 | -32 | -14 | 14 | 739 | |
Claustrum | 1 | 1.07 e-3 | 3.07 | -32 | -10 | 10 | ||
Claustrum | 1 | 1.87 e-3 | 2.9 | -30 | 8 | 4 | ||
Claustrum | 1 | 5.19 e-4 | 3.28 | -34 | -4 | 6 | ||
Putamen | 1 | 2.05 e-3 | 2.87 | -30 | -14 | -6 | ||
Middle occipital gyrus | 2 | 5.91 e-5 | 3.85 | -24 | -92 | -6 | 18 | 774 |
Middle occipital gyrus | 2 | 2.26 e-3 | 2.84 | -20 | -94 | 10 | 18 | |
Inferior occipital gyrus | 2 | 1.72 e-4 | 3.58 | -30 | -88 | -12 | 18 | |
Middle occipital gyrus | 2 | 8.84 e-5 | 3.75 | -26 | -90 | 0 | 18 | |
Lingual Gyrus | 2 | 6.67 e-5 | 3.82 | -18 | -92 | -8 | 17 | |
Lingual gyrus | 2 | 2.89 e-3 | 2.76 | -14 | -100 | -16 | 17 | |
Lingual gyrus | 3 | 6.73 e-9 | 5.68 | 20 | -88 | -8 | 17 | 1342 |
Middle occipital gyrus | 3 | 1.81 e-5 | 4.13 | 26 | -94 | 8 | 18 | |
Middle Occipital Gyrus | 3 | 9.34 e-6 | 4.28 | 34 | -90 | -8 | 18 | |
Cuneus | 3 | 2.35 e-5 | 4.07 | 14 | -98 | -6 | 17 | |
Middle occipital gyrus | 3 | 6.67 e-5 | 3.82 | 32 | -92 | 0 | 18 |
We also investigated the contrast CoTv - CoTc. Both conditions share the same search displays, i.e. the same predictive contexts, but the target does appear at the predicted location in CoTv, but at unpredicted locations in CoTc. Therefore a positive prediction error should lead to higher activation in CoTv than CoTc. This was observed in the left hemisphere in the posterior putamen, posterior insula and precentral and postcentral gyri (Fig. 4b). No significant activation was observed for the reversed contrast.
Finally, we contrasted CoTv with CnTf, in which the distractor configurations never repeated and therefore no predictions of the target location could be learnt from the context. This contrast yielded increased activation in the left putamen as well as the left insular cortex and in the left inferior parietal lobule (Fig. 4c). Again, no significant activation was observed for the reversed contrast.
The activations obtained in the contrasts of CoTv versus CoTf, CoTc and CnTf overlapped in the left posterior putamen and the left posterior insula (Fig. 4d).
Since we have observed intrinsic prediction error-like signals in visual category learning in putamen and nucleus accumbens [22], we repeated the ANOVA on a nucleus accumbens mask created using the probabilistic “Harvard-Oxford Subcortical Structures” atlas, thresholded at 50% probability. Even by enhancing power with this ROI-analysis, we observed no significant activation for any of the three contrasts.
DISCUSSION
We investigated the neural response to repeatedly presented search configurations in which the target occurred either at the learnt location or at a new location that was not predicted by the distractor context. Based on previous work [22] we hypothesized that the presence of the target at the location predicted by the search configuration might elicit a prediction-error-like response. This response was expected to occur when an - implicitly - learnt search configuration allowed the - again implicit - “prediction” of the target location with less than 100% certainty and the target appeared at the predicted location. In order to distinguish a prediction error-like response from a response signaling the internally rewarding occurrence of a target at the predicted location, we compared repeated search displays in which the target appeared at the learnt location with 100% or 50% probability. An internal reward signal should occur equally in both cases, whereas a prediction error-like response should be only obtained with 50% probability. When we contrasted target occurrence at a location predicted by 50% versus 100% by the distractor configuration (CoTv - CoTf), we observed an increased response in the left posterior putamen, in line with previous work demonstrating putamen activation to reflect prediction-error like responses in situations in which external reward is missing and subjective confidence replaces external reward as feedback signal [23].
If the putamen activation was elicited by a prediction error-like response, it should mainly be observed when we contrast the search configurations in which the target occurs at the location predicted with 50% probability with the same configurations, but the target occurring at a different location. Again, this response pattern was observed in left putamen, overlapping with the contrast 50% - 100% predictability. Because the identical search configurations were compared in this contrast (CoTv - CoTc), confounding effects elicited by different search configurations can be ruled out.
Finally, a prediction error-like response should be observed when targets occurring at a location predicted with 50% probability were contrasted with search in novel configurations, where no prediction could be developed. This contrast also led to an activation of the left putamen that overlapped with the two previous contrasts.
Taken together, these contrasts show that the posterior putamen was more activated when targets were presented at the predicted location, but only when the prediction was uncertain. This differential pattern rules out that the putamen activation is driven by context learning in general. The pattern is consistent with the response pattern of a positive prediction error. Single cell studies found two typical types of prediction error-like responses. Some neurons displayed increased firing for positive prediction errors and reduced firing for negative prediction errors while others responded with increased firing to both kinds of prediction errors [42; motivational “valence versus salience” coding]. Moreover, dopaminergic neurons may code motivational valence or salience depending on reward certainty [43]. In the present experiment, increased activation for CoTv over CoTc taken alone could thus be interpreted both as increased neuronal firing due to unexpected reward (CoTv increase; positive prediction errors) or decreased firing due to reward omission (CoTc decrease; negative prediction errors). Only the additional observation of increased activation in the CoTv - CoTf contrast suggests that the positive prediction errors interpretation is more likely. CoTf can serve as a baseline, because no prediction errors is expected to occur when the reward is 100% certain. In addition, the stronger activation for CoTv displays in comparison to new (CnTf) displays - while there is no decrease in CoTc activation in comparison to CnTf displays - strengthens the positive prediction error interpretation because there is no learning history and thus no prediction error for CnTf displays. We may not be able to see neuronal activity that codes motivational salience rather than valence, previously observed in left putamen [44], because this may lead to comparable signal increase for Cotv and CoTc and thus cancel each other in the CoTv - CoTc contrast.
A positive prediction error should - at least slightly - increase over the course of the experiment because the accumulated target location probability decreases from the onset of Block 2 of the scanner session to the end of the experiment. This increase was not observed. There was no significant change of the CoTv - CoTc contrast in the putamen. However, over the course of the experiment, there were only 6 instances of changed target position for every display. Thus, the accumulated probability of a target appearing at the learnt location changed between ca. p=.95 for the first possible change in the 5th block of the scanner session (target at expected location in 20 of 21 blocks in the learning and scanner session) to ca. p=.81 for the 6th change (target at learnt location in 26 of 32 blocks). This rather modest change of target location probability plus the relatively low statistical power makes it unlikely to observe a change of the prediction error size in our experiment.
It should be noted that search for targets occurring at a location predicted with 50% probability by the search configuration led to faster search times than in the other three conditions. Therefore, it can be ruled out that the putamen activation was simply due to unspecific factors tied to higher effort. Could the reverse be true, that the putamen is more strongly activated when fast responses are elicited? Although the putamen is part of the motor loop [1, 2], we have not found previous reports that the putamen might be more active during fast responses. Moreover, we included search time in the analysis to eliminate its influence on the contrasts of interest. In addition, the posterior insula activation was distinct from previously observed activation of the anterior insula due to visual search difficulty or visual working memory demands [45]. Unfortunately, due to coding error, the targets in the CoTf condition were on average located more peripherally than in the other conditions. Therefore, the longer search times for CoTf cannot be meaningfully interpreted. If anything, though, this error led to a more conservative test of the CoTv - CoTf contrast reported above.
The putamen was one of two structures where we observed a prediction error-like response based on internal confidence in a previous visual category learning study [22]. The other structure was the nucleus accumbens, another prominent dopaminergic structure where a prediction error-like response might be predicted in the present study as well. We did not observe such a response in the whole brain analysis. An additional ROI-analysis of the nucleus accumbens also yielded no evidence for a prediction error-like signal in this structure. Thus, there appears to be a difference between the involvement of the nucleus accumbens in our previous study, utilizing an information-integration category learning task, and the present study. The paradigms used in the present and the previous studies differ in many respects. Other than in the category learning task, participants in the current study did not know that they took part in an incidental learning task and the recognition test showed that they could not explicitly discriminate between repeated and novel displays. In information-integration category learning, single objects are presented at fixation, so there is no visuospatial search component. In a recent study investigating distractive effects of previously rewarded items in visual search, no ventral striatal activation was observed [46]. Likewise, in a study of monetary reward modulation of contextual cueing, we found no increase of nucleus accumbens activation for repeated search displays that were associated with high reward [47]. While the involvement of nucleus accumbens - hippocampus circuits in spatial context conditioning has been shown in the rat [48], visual search tasks or other tasks with high visuospatial demands have not typically been used to test nucleus accumbens function (see [49] for a recent review). Thus, it might be useful to study the contribution of the nucleus accumbens to the reward modulation of visuospatial demands in comparison to non-spatial demands in future experiments. Ranganath and Ritchey [50] have argued for a dissociation of an anterior temporal and a posterior medial memory system, the former processing object identity and value, the latter contextual aspects of memory. It may turn out that the nucleus accumbens contributes more to the anterior temporal than the posterior medial memory system.
In addition to the insular/putamen activation, there was a bilateral occipital activation in the contrast CoTv-CoTf that was not observed in the comparison of CoTv with either CoTc or CnTf. This activation cannot be explained by visual search processes per se, because search times were longer in CoTf than CoTv and, additionally, search time was explicitly modeled. We would, albeit carefully, interpret this finding as due to memory encoding processes. CoTf is the only condition in which further encoding of spatial structure is absent or minimal because the occurence of 100% predictable “reward” (target at predicted location) affords no further learning. This is different in both CoTc and CoTv, in which the “reward” probability changes with every trial. Encoding will also occur in CnTf, simply because the displays are novel. Previous research from our lab has shown that occipital cortex can be more activated in repeated displays in contextual cueing, although this was rather retrieval-related activation in this former study [51].
CONCLUSION
We observed increased left posterior putamen activation when visual search targets were presented at the location predicted by the spatial distractor context and when the prediction was uncertain. This pattern fits a positive prediction error signal that is typically observed in reward learning experiments with explicit reward. The current data show that this concept can be transferred to implicitly rewarding situations in visual search, in line with previous evidence for a similar role of the putamen in visual category learning. Our findings complement previous studies that have demonstrated a role of the putamen in prediction errors arising from external feedback [18] as well as from subjective confidence [22].
CONFLICT OF INTREST
The authors confirm that this article content has no conflict of interest.
ACKNOWLEDGEMENTS
We thank Franziska Geringswald and Florian Baumgartner for technical support. This work was funded by Deutsche Forschungsgemeinschaft (Sonderforschungsbereich 779, TP A04).