All published articles of this journal are available on ScienceDirect.
DMN Operational Synchrony Relates to Self-Consciousness: Evidence from Patients in Vegetative and Minimally Conscious States
Abstract
The default mode network (DMN) has been consistently activated across a wide variety of self-related tasks, leading to a proposal of the DMN’s role in self-related processing. Indeed, there is limited fMRI evidence that the functional connectivity within the DMN may underlie a phenomenon referred to as self-awareness. At the same time, none of the known studies have explicitly investigated neuronal functional interactions among brain areas that comprise the DMN as a function of self-consciousness loss. To fill this gap, EEG operational synchrony analysis [1, 2] was performed in patients with severe brain injuries in vegetative and minimally conscious states to study the strength of DMN operational synchrony as a function of self-consciousness expression. We demonstrated that the strength of DMN EEG operational synchrony was smallest or even absent in patients in vegetative state, intermediate in patients in minimally conscious state and highest in healthy fully self-conscious subjects. At the same time the process of ecoupling of operations performed by neuronal assemblies that comprise the DMN was highest in patients in vegetative state, intermediate in patients in minimally conscious state and minimal in healthy fully self-conscious subjects. The DMN’s frontal EEG operational module had the strongest decrease in operational synchrony strength as a function of selfconsciousness loss, when compared with the DMN’s posterior modules. Based on these results it is suggested that the strength of DMN functional connectivity could mediate the strength of self-consciousness expression. The observed alterations similarly occurred across EEG alpha, beta1 and beta2 frequency oscillations. Presented results suggest that the EEG operational synchrony within DMN may provide an objective and accurate measure for the assessment of signs of self-(un)consciousness in these challenging patient populations. This method therefore, may complement the current diagnostic procedures for patients with severe brain injuries and, hence, the planning of a rational rehabilitation intervention.
INTRODUCTION
The brain ‘default mode network’ (DMN) is commonly defined as a set of cortex areas, encompassing mostly left and right middle frontal gyruses, bilateral frontal medial areas, left and right middle temporal and occipital gyruses, and left and right precuneus, that are more active when the subjects are at rest than when they are engaged in attention-demanding tasks [3-5]. The activity of these areas is usually measured indirectly using metabolically/hemodynamically based methods (PET and fMRI) by evaluating the local metabolic or hemodynamic activity.
The DMN has been consistently activated across a wide variety of self-related tasks [6-11], thus leading to the proposal (even though interpretations vary) of DMN’s role in self-related processing [9, 12] not only during the presentation of self-specific stimuli, e.g., stimulus-induced activity, but also in the resting state itself. For example, Christoff et al. [13], Wicker et al. [14], Gusnard [15], Schilbach et al. [16], and Lou et al. [12] explicitly assume that the default network may be a neural correlate for a “sense of self”. They argue that this network may indeed support the integration of self-referential information while creating an “autobiographical” self [8, 17], remembering one’s past and planning one’s future [18, 19], performing “chronic” self-evaluation [20], or having self-consciousness [15, 16, 21]. Recently direct causal relationship link between DMN and self-related processing has been demonstrated with the transcranial magnetic stimulation (TMS), a non-invasive means of temporarily modulating neural function [12]. In present article, following Northoff et al. [22], we assume that self-referential processing is common to the distinct concepts of self in different domains; meaning that self-referential processing is the core of what constitutes the ‘experiential self’.
It is now widely recognized that in the absence of a full understanding of neural correlates of self-consciousness, it remains difficult to interpret functional imaging data in subjects as proof or disproof of their conscious experience [23]. Recently it has been shown [24] that DMN could be reliably identified by electroencephalogram (EEG) operational connectivity analysis in healthy volunteers. Based on the analysis performed, authors suggested that DMN has a specific functional connotation – it could serve as neurophysiologic basis for self-processing operations, namely first-person perspective taking and an experience of agency [16, 25]. This suggestion is compatible with the fact that both functionally connected DMN and self-awareness are persistently present through wakefulness during rest, cognitive tasks [24] and sleep (during dreaming) in healthy subjects [26, 27].
In spite of the fact that the DMN is rapidly becoming the central theme in contemporary human neuroscience, the number of studies in this area using EEG methodology is still disproportionally small. EEG studies are important since they help to refute claims that DMN observations constitute an epiphenomenon of non-neuronal origin [28]. Moreover these studies hold the potential to advance our understanding of the neural correlates and mechanisms of the DMN, because, in contrast to metabolic/hemodynamic methods, EEG is a direct measure of electric current within masses of neuronal cell assemblies [29, 30]. Recent simultaneous EEG and fMRI studies have been shown that BOLD resting state networks (including DMN) are indeed associated with spontaneous EEG activity [31, 32]. Additionally, only the EEG (and magnetoencephalogram; MEG) provides a satisfactory temporal scale for accessing the dynamic evolution of brain activity associated with cognitive and conscious processes in healthy and diseased states [33-35]. Indeed, by treating the brain as a dynamic system from which we are able to observe certain physiological parameters over time [36] and since it has been suggested that transient synchronization of neuronal systems is essential for brain operation and conscious performance [35-39], a temporal resolution in the order of milliseconds is of special interest [40].
AIM OF THE STUDY AND HYPOTHESIS
In our previous study [24] the relation of EEG operational synchrony within the DMN and expression of self-consciousness was not studied explicitly. To make the suggested statement about putative relation of DMN and self-consciousness stronger, further work was needed to experimentally demonstrate that EEG operational synchrony within DMN structures is weakened or even lost in circumstances when self-awareness expression was also weakened or lost. One approach to do so is to study patients who have disturbances of the self as a result of general anesthesia or brain damage.
Patients with severe brain injuries offer a unique opportunity to study the neural basis of self-(un)consciousness due to the fact that impairment in awareness of self (as well as of environment) is dissociated in such patients from preserved and stable wakefulness1 (see Fig. 1). On the contrary, in patients under general anesthesia it is not possible to disentangle impairment in awareness from impairment in arousal [42]. Awareness is the hallmark of phenomenal consciousness, which refers to a higher level of organization in the brain [26]; it captures all immediate and undeniable (from the first-person perspective) phenomena of subjective experiences (concerning self, hearing, seeing, touching, feeling, embodiment, moving, and thinking) that present to a person right here (subjective space) and right now (subjective present) [43]. It is thought that consciousness is an emergent phenomenon of coherent dynamic binding of operations performed by multiple neuronal assemblies organized within nested hierarchical brain architecture (for a detailed review and discussion see [43]).
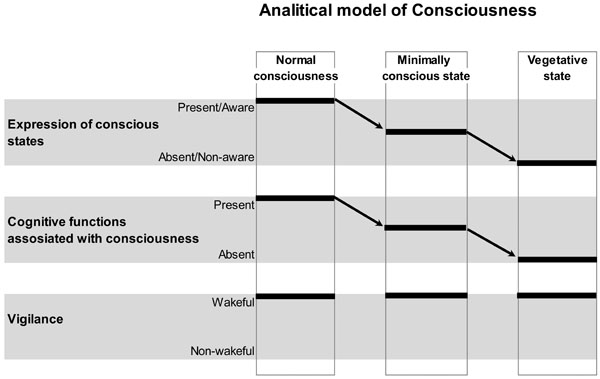
Schematic Illustration of the Analytical Model of Consciousness. On the horizontal plane, conscious states and related with them cognitive functions, as well as vigilance states are plotted. On the vertical plane, normal conscious state, minimally conscious state and unconscious (vegetative) state conditions are presented. Arrows indicate a decrease in the expression of conscious states and related with them cognitive functions from normal, to minimal and to unconscious conditions. Vigilance is supposed to be nearly identical in all three conditions. Therefore, within this analytical model, conscious expression could be reliably dissociated from the vigilance. However, it is clear from the graph that the expression of consciousness and the related with it conscious cognitive functions/operations could not be disentangled. This is a limitation of such a model. Figure is modified from [41].
Therefore the aim of the present study was to investigate the strength of DMN EEG connectivity during resting state in patients who are in vegetative state (VS) and minimally conscious state (MCS) and compare it to DMN resting state EEG connectivity in healthy subjects. Specifically we hypothesized that if DMN is the direct neural correlate of self-awareness as it is currently belived (for the review see [22, 24, 25]), the decrease of EEG DMN connectivity strength should parallel the decrease of expression of self-consciousness in non- or minimally communicative patients with severe brain injuries, as assessed by standardized Level of Cognitive Functioning (LCF) scale [44] and be different between patients with disorders of consciousness and healthy subjects. Furthermore, we expected a particularly strong link between the expression of self-consciousness and connectivity in the frontal pole of DMN, which is reported to play a central role in self-referential processing [11, 45-49], and providing a critical self-related context (experience of agency and point of view) for all human behaviors and activities [24].
To the best of our knowledge the present electrophysiological study is the first EEG DMN connectivity study in patients with severe brain injuries. At the same time, using fMRI analysis it has already been shown that DMN functional connectivity is absent in brain death [50], is extremely low in VS patients [50, 51] and is slightly decreased in MCS patients [50] when compared to healthy subjects.
METHODS
Subjects
The current study was based on the cohort of subjects (patients and healthy volunteers) participating in our previous investigation [41]. There were 21 non- or minimally communicative patients with severe brain injuries suffering from different consciousness disorders, admitted in Neurorehabilitation Unit at the Fondazione Istituto “San Raffaele - G. Giglio” to carry out an intensive neurorehabilitation program.
Upon admission all patients were submitted to a thorough and comprehensive clinical neurological examination. The diagnosis of VS and MCS was made according to currently accepted diagnostic criteria [52-54]. Additionally, the Levels of Cognitive Functioning (LCF) score [44] was assessed on the day of admission and three days later when the EEG was registered. We choose to use the LCF scale instead of the Glasgow Outcome Scale (GOS) [55], the Glasgow Coma Scale [56] or the JFK Coma Recovery Scale [57], because LCF evaluates not only behavioral patterns, but cognitive functions also (which are closer related to consciousness than behavioral patterns), and LCF has been found better related with the presence of EEG abnormalities in patients with disorders of consciousness in previous studies [58, 59]. The LCF scale has different grades ranging from 1 to 8 (1 – patient does not respond to external stimuli and/or command; 8 – patient is self-oriented and responds to the environment, but abstract reasoning abilities are decreased relative to pre-morbid levels).
Based on the strict adherence to the aforesaid diagnostic criteria 14 of the patients (mean age 42.9 ± 20 years) were classified as being in a vegetative state (VS) and the remaining 7 patients (mean age 48.7 ± 19.8 years) were classified as being in a minimally conscious state (MCS). Patients in VS had a LCF score of 1 or 2 while patients in MCS had a LCF score of 3. In order to reduce the variability of clinical evaluation, LCF scores were assigned in all patients only if they were unchanged for the day of admission and the day of EEG registration (three days later); otherwise, patients were excluded from the study. Other exclusion criteria for the patients comprised (a) any acute comorbidity or unstable vital signs; (b) obvious communicating or obstructive hydrocephalus; (c) a history of neurological disease before admission; (d) severe spasticity (causing constant EMG artifacts). Inclusion criteria for the patients included (a) confirmation of diagnosis of VS or MCS according to the clinical definitions [52, 53, 60]; (b) LCF = 1–2 for VS and 3 for MCS patients; (c) no more than 3 months after acute brain event onset; and (d) first-ever acute brain event.
The control group was age matched and consisted of drug-free, healthy volunteers of both sexes (N = 5, mean age 33.2 ± 5.3 years). Before inclusion, the control subjects underwent a medical examination. Control subjects had no significant medical illnesses, were free from psychotropic medication, and none had a history of psychiatric and neurological disorders.
The study was approved by the local institutional Ethics Committee, and complies with Good Medical Practice. Informed and overt consent of subjects’ legal representatives, in line with the Code of Ethics of the World Medical Association (Declaration of Helsinki) and standards established by the Fondazione Istituto “San Raffaele - G. Giglio” Review Board were acquired. The use of the data was authorized by means of written informed consent of the subjects (controls) or caregivers (VS and MCS patients).
EEG Recording
Spontaneous electrical brain activity was recorded with a 21-channel EEG data acquisition system (Neuropack electroencephalograph; Nihon Kohden, Tokyo, Japan). EEG data were collected (cephalic reference – mean of the signals from C3 and C4 electrodes; 0.5–70 Hz bandpass; 200 Hz sampling rate; around 30 min for patients and 5 min for controls) in subjects during a waking resting state (eyes-closed) from 19 electrodes positioned according to the International 10–20 system (i.e. O1, O2, P3, P4, Pz, T5, T6, C3, C4, Cz, T3, T4, F3, F4, Fz, F7, F8, Fp1, Fp2). The impedance of recording electrodes was monitored for each subject and was always below 5 kΩ. To monitor eye movements, an electrooculogram (0.5–70 Hz bandpass) was also collected.
The EEG recordings were performed late morning for all subjects. The control subjects and patients were requested to be relaxed and engaged in no specific mental activity during EEG recording. EEG recordings in patients were started in all cases only if patients spontaneously had their eyes open, the eyelids were then closed by hand. The eyes were closed by hand all the time until the end of EEG registration. At the end of the recordings all patients opened their eyes spontaneously thus suggesting that the level of vigilance (i.e. capability to open eyes) was unchanged compared to the onset of the EEG. In order to keep a constant level of vigilance, an experimenter monitored subject’s and patient’s EEG traces in real time, looking for signs of drowsiness and sleep onset (increase of “tonic” theta rhythms, K complexes and sleep spindles; behavior in control subjects). The presence of an adequate EEG signal was determined by visual inspection of the raw signal on the computer screen. Even though it may be difficult to assess precisely the level of vigilance in patients in VS, preserved sleep patterns may be observed in the majority of patients in VS (for review see [61]).
EEG-Signal Data Preprocessing
The presence of an adequate EEG signal was determined by visually checking each raw signal. Epochs containing artefacts due to eye movements, eyes opening, significant muscle activity, and movements on EEG channels were marked and then automatically rejected from further analysis.
A full EEG stream for each subject free from any artifacts was fragmented into consecutive epochs of 1 min. Therefore the “NORM” group (healthy subjects) has 18 one-min EEGs, “MCS” group (patients in minimally conscious state) has 87 one-min EEGs and “VS” group (patients in vegetative state) has 137 one-min EEGs. Further data processing was performed separately for each 1-minute portion of the signal within each group. Due to the technical requirements of the tools used to process the data, EEGs were re-sampled to 128 Hz. This procedure should not affect the results since 128 Hz sampling rate meets the Nyquist Criterion [62] of a sample rate greater than twice the maximum input frequency for the alpha and beta activity and is sufficient to avoid aliasing and preserve all the information about alpha and beta activity in the input signal. This method was considered sufficient since the sampling rate of the source signals was significantly higher than required.
After re-sampling and prior to further processing procedures, each EEG signal was bandpass-filtered (Butterworth filter of the sixth order) in the alpha (7–13 Hz), beta1 (15–25 Hz) and beta2 (25–30 Hz) frequency bands. Phase shifts were eliminated by forward and backward filtering.
The alpha and beta frequency bands were chosen for several reasons. First, it has been demonstrated that the DMN has significant positive correlation with alpha and beta rhythms [63-65]. Second, in our previous study [41], among five EEG frequency bands (delta, theta, alpha, beta1 and beta2) only alpha, beta1 and beta2 rhythms have shown behavior consistent with the analytical consciousness model proposed in Fingelkurts et al. [66]. According to this model (Fig. 1) the features of EEG which are responsible for the subjective (un)awareness of self and environment should satisfy one of the following rules: (a) NORM ≥ MCS > VS (for awareness) or (b) NORM ≤ MCS < VS (for unawareness). Third, administration of different type of anesthetics (thiopental, propofol, etomidate, sevoflurane, ketamine) demonstrated considerable decrease only in alpha and beta EEG frequencies at the moment of loss of consciousness or shortly after it [67, 68]. The emergence from unconsciousness was characterized by the opposite EEG effect in the same frequencies.
Additionally, for the purposes of the present study it is important that alpha activity (a) is expected to play a leading part in organization of conscious interactions (specifically human – from the first point of view) with the environment [69], (b) is correlated with conscious awareness [70] and (c) is highly correlated with mind wondering and spontaneous self-referential thoughts [71]. Beta activity, on the other hand, plays a crucial role in “internal” attention [72, 73], is related to self sensory-motor processing and is strongly correlated with conscious mentation (as opposed to mental automation) [74-76], and is particularly important for the maintenance of a conscious audio-visual image [77].
A Brief Introduction to Operational Architectonics (OA) Methodology
To address the aims of the present study we need to estimate the strength of EEG functional (operational) connectivity within DMN in MCS and VS patients and then compare it with the NORM group. In the past several years there has been an explosion of interest in the tools that can be used to study brain connectivity (for a review see [78, 79]). Even though these tools borrow complex methodologies and conceptual frameworks from physics, mathematics, and engineering, none of them possess adequate conceptual power to address the subject of consciousness, because they are not motivated by the theories of consciousness2. This is why we applied an Operational Architectonics (OA) methodology [1, 2] to EEG analysis in order to estimate the spatio-temporal patterns of operationally connected cortical areas. Among the different methodological strategies adopted to study and describe consciousness expression and interrelation within the complexity of brain activity, the OA framework has an advantage because of its compromise between mathematical simplicity, neurophysiological accuracy, and cognitive and phenomenal plausibility [82-84]. The OA framework studies a nested hierarchical architecture of brain operations with an explicit role for consciousness (for a detailed analysis see [43]). Accurate analysis of the complex structure and hierarchical architecture of EEG signals reveals the existence of a particular operational space–time which literally resides within the brain’s internal physical space–time and is functionally isomorphic to the phenomenal space–time [43]. As we proposed elsewhere [41], such operational space–time could constitute the neurophysiological basis of mind phenomenal (subjective) architecture (for a similar view see [85]).
The approach to study coordinated activity between different brain areas which is utilized within the OA framework is very different from conventional methods (such as coherence, phase synchrony, and others; see [79] for comparison and analysis of limitations). It fits into a more general class of nonlinear interdependencies between dynamic systems, in which rapid transients in one system can be directly mapped to a second system. The main benefit of this synchrony over others that quantify coherence, correlation, and phase synchronization is conceptual [1, 2]. Contrary to findings of previous EEG studies on functional connectivity, this measure is well suited to extract information about discrete operations of neuronal assemblies from EEG recordings and to estimate the level of inherent synchrony of these operations appearing simultaneously and locally in different cortical areas (for the experimental support see [2]). It can therefore quantify a broader range of coordination processes, especially metastable and non-static nonlinear phenomena [2, 79, 86]. Therefore, the OA framework provides a natural explanation for how, in the words of Baars [87], “a serial, integrated and very limited stream of consciousness emerges from a nervous system that is mostly unconscious, distributed, parallel and of enormous capacity”.
First level of OA – Estimation of the Local Functional Interrelations
OA theory explores the temporal structure of information flow and inter-area interactions within a network of dynamical, transient, and functional neuronal assemblies (which activity is “hidden” in the complex non-stationary structure of EEG signal; see [88]) by examining topographic rapid transition processes (on a millisecond time scale) in local EEGs [1, 2, 89]. An abrupt transition during which the EEG amplitude changes significantly separates each quasi-stationary EEG segment from those preceding and following it, and thus imposes a discontinuity in local cortical dynamics [43]. To uncover these segments of quasi-stationarity from the complex nonstationary structure of local EEG signals, adaptive segmentation procedures should be used [2]. The aim of the segmentation is to divide the EEG signal into naturally existing quasi-stationary segments by estimating the intrinsic points of “gluing” - rapid transitional periods. These instants (the transient phenomena) observed within a short-time window, when EEG amplitude changes significantly, are identified as rapid transition processes (RTP) [2]. RTP is of minor length compared to the quasi-stationary segments, and therefore can be treated as a point or near-point [2, 88]. Thus, the RTPs (or jumps in EEG amplitude) are, in fact, the markers of boundaries between concatenated quasi-stationary segments. RTP is defined as an abrupt change in the analytical amplitude of the signal3 above a particular threshold which is computed based on the developed statistical procedure established experimentally for each local EEG in modeling and empirical studies [2].
According to the OA framework each homogeneous segment in the EEG signal corresponds to a temporary stable microstate – an operation executed by a neuronal assembly [1, 89]. The transition from one segment to another then, reflects the moment of abrupt switching from one neuronal assembly operation to another (see an example in [2]). Even though each cortical area consists of several neuronal assemblies, their dynamics are easily and naturally separated based on a coordinated timing that is enabled by oscillations, which can be measured by EEG frequency bands [29, 30, 91-93]. Experimental studies have shown that different frequencies appear to be related to the timing of different neuronal assemblies, which are associated with different types of sensory and cognitive processes (for a detailed overview, analysis and discussion see [43]).
The general statistical principles of the microstate segmentation have been described extensively elsewhere [1, 2, 88, 89]. Therefore, here we provide only a brief overview of this approach. Adaptive segmentation procedure [2] was used for automatic segmentation of local EEG signals within the multichannel EEG record. This method is based on an automated algorithm that moves a double window screening along each separate EEG signal/channel. The following steps were taken to estimate RTPs: (1) Comparisons were made between ongoing EEG amplitude absolute values averaged in two windows – ‘test’ and ‘level’ (duration of test window << duration of level window), both starting from the first data point. The durations of test and level windows were identical across different subjects and EEG channels for each frequency band (but different between the bands) to guarantee the best conditions for RTP evaluation. (2) If the absolute maximum of the averaged amplitude values in the test window is less or equal to the averaged amplitude values in the level window, then the hypothesis of EEG homogeneity is accepted. (3) If the absolute maximum of the averaged amplitude values in the test window exceeds the averaged amplitude values in the level window, according to the threshold of “false alerts”—first condition (the Student criteria)- its time instant becomes the preliminary estimate of the RTP. (4) A second condition must be fulfilled to eliminate “false alerts” associated with possible anomalous peaks in the amplitude; the five points of the digitized EEG following this preliminary RTP must have a statistically significant difference between averaged amplitude values in the test and level windows (Student’s t-test). (5) If these two criteria are met, the preliminary RTP is considered real. (6) Thereafter, both windows are shifted from this RTP on one time-point, and the procedure is repeated [2].
With this technique, the sequence of RTPs with statistically proven (p < 0.05, Student’s t-test) time coordinates is determined for each EEG channel and for each 1-min epoch individually.
The choice of windows’ duration and of the ‘false alerts’ rule was made during a preliminary modeling studies [2] to guarantee the following two goals: (a) to detect as many of real RTPs as possible and (b) to keep level of ‘contamination’ of the results by ‘false’ RTPs reasonably low.
Second level of OA – Estimation of the Remote Operational (Functional) Connectivity
Temporal synchronization of multiple brain operations executed by different local and transient neuronal assemblies (operational synchrony) gives rise to a new level of brain abstractness – metastable brain states [1, 89]. These metastable brain states or functional Operational Modules (OMs), as we name them, supposed to underlie the realization of complex brain macrooperations: cognitive percepts, phenomenal objects, and reflective thoughts within the operational space-time continuum [43]. The synchronization of operations (i.e. operational synchrony) produced by different neuronal assemblies, which are located in different cortex regions, serve to bind spatially dispersed phenomenal features (bases of sensations) of a multimodal stimulus or objects into integrated and unified patterns of qualities and further into phenomenal objects or complex scenes [43] (see also [85]). Each OM is considered to be a metastable spatial-temporal pattern of brain activity, because the neuronal assemblies which are supposed to constitute it produce different operations/functions and each does its own inherent “job” (thus expressing an autonomous tendency), while at the same time still being temporally entangled with one other (and thus expressing the coordinated tendency) in order to execute a common complex operation or cognitive act of a higher hierarchy [86, 94]. As proposed by Kelso [95], metastability relates exactly to the constant interplay between these autonomous and interdependent tendencies (for further discussion see [39, 96]).
At the EEG level, OM phenomenon is expressed through synchronization of the EEG quasi-stationary segments (indexed by Structural Synchrony, ISS) obtained from different brain locations [1, 2]. The details of this technique are beyond the scope of this article therefore we will only concentrate on some essential aspects. In brief, each RTP in the reference EEG channel (the channel with the minimal number of RTPs from any pair of EEG channels) was surrounded by a short “window” (ms). Any RTP from another (test) EEG channel was considered to coincide if it fell within this window. It can be noted that such coincidence of RTPs is related to a specific type of signal coupling – the structural synchronization of discrete events – which completely ignores the level of signal synchronization in the intervals (segments) between the coinciding RTPs [2]. To arrive at a direct estimate at the 5% level of statistical significance (p < 0.05) of the ISS, computer simulation of RTPs synchronization was undertaken based on random shuffling of time segments marked by RTPs (500 independent trials). As a result of this procedure, the stochastic levels of RTP coupling (ISSstoh), together with the upper and lower thresholds of ISSstoh significance (5%) were calculated.
The ISS tends towards zero if there is no synchronization between EEG segments derived from different EEG channels and has positive or negative values where such synchronization exists. Positive values (higher than upper stochastic level) indicate ‘active’ coupling of EEG segments (synchronization of EEG segments is observed significantly more often than expected by chance as a result of random shuffling during a computer simulation), whereas negative values (lower than low stochastic level) mark ‘active’ decoupling of segments (synchronization of EEG segments is observed significantly less than expected by chance as a result of random shuffling during a computer simulation). Dynamics of ISS and its positive and negative levels are shown on the Fig. (2). The strength of EEG structural synchrony is proportional to the actual value of ISS: the higher this value, the greater the strength of functional connection.
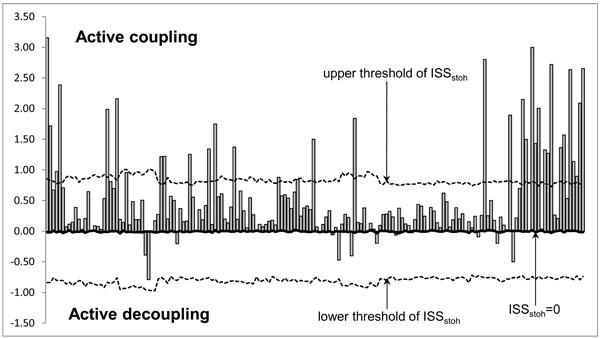
Schematic Illustration of the Index of Structural Synchrony (ISS) and its Stochastic Levels. As an example, the calculations of ISS are shown for a healthy subject in rest condition, eyes closed. The Y-axis displays the ISS values found in the experiment (illustrated as bars). The X-axis displays the 170 possible pair combinations of 20 EEG channels (1 = O1-O2, 2 = O1-P3, 3 = O1-P4, … 168 = Fz-F7, 169 = Fz-F8, 170 = F7-F8).
Using pair-wise analysis, structural synchrony was identified in several (more than two) channels – synchrocomplexes (SC); these are described as operational modules – OM. The criterion for defining an OM is a sequence of the same synchrocomplexes (SC) during each 1-min epoch, whereas a SC is a set of EEG channels in which each channel forms a paired combination with valid values of ISS with all other EEG channels in the same SC; meaning that all pairs of channels in an SC have to have statistically significant ISS linking them together.
As it has been proposed in our previous EEG study [24] a constellation of nine operationally synchronized cortical areas indexed by 3 distinct OMs (frontal OM: F3-Fz-F4; left posterior OM: T5-P3-O1; and right posterior OM: T6-P4-O2) could account, in large part, for the DMN. Therefore the following scalp EEG positions (and correspondent to them cortical areas [97]) were used to estimate the operational synchrony within such OMs in this study: EEG positions F3 and F4 (left and right middle frontal gyruses or Brodmann’s area 8), EEG position Fz (bilateral medial areas or Brodmann’s area 6), EEG positions T5 and T6 (left and right middle temporal gyruses or Brodmann’s area 21), EEG positions P3 and P4 (left and right precuneus or Brodmann’s area 19), and EEG positions O1 and O2 (left and right middle occipital gyruses or Brodmann’s area 18). These anatomical correlations of EEG electrode positions were taken from the reference study of Koessler et al. [97], where matching between EEG electrode positions and anatomical areas of the cortex was clearly established and analyzed using a new EEG-MRI sensor system and an automated projection algorithm (see also [98] for the correlations between EEG activity in a given electrode positions and correspondent to them cortical areas).
Although it is often claimed that volume conduction is the main obstacle in interpreting EEG data in terms of brain connectivity, it has been shown previously experimentally and through modeling studies that the values of the ISS are sensitive to the morpho-functional organization of the cortex rather than to the volume conduction (for relevant details, we refer the reader to [2, 88]; substantial discussion of brain activity sources problem and volume conduction is presented in section 3.2 in [24]).
Statistics
The strength of EEG operational synchrony within the DMN was assessed using an ISS index (see previous subsection). The differences in strength of ISS patterns between different groups (NORM, MCS, and VS) were assessed using Wilcoxon t-test, which is used in the majority of functional connectivity studies (for the overview, see [99]). At first, all strength values of EEG ISS were averaged within each OM for all 1-min EEGs per group (NORM, MCS, or VS). During the final stage an average of ISS strength values for all three OMs was calculated for each group. Since we compared several conditions at a time, a Bonferroni correction was made in order to control for repeated observations of the same measures. P corrected is the value required to keep the number of false positives at p = 5 %. Differences in the demographic data were assessed either by Wilcoxon t-test or by Chi-square test. Differences in the distribution of the percent changes between groups were assessed by Chi-square test.
RESULTS
Demographic Data
There were no significant differences between patients and healthy participants in terms of age (p = 0.28). There were no significant differences between the MCS and VS groups in terms of age (p = 0.41) and time post brain injury (p = 0.46), as well as distribution of TBI and non-TBI etiologies (43% TBI and 57% non-TBI in both groups), left- and right-side lesions (p = 0.62) and medicated vs non-medicated patients (p = 0.82).
EEG Operational Synchrony Strength within the DMN
We observed a significant decrease (pcorrected < 0.05-0.01) in the average strength of EEG operational synchrony (measured by the ISS index) within the DMN in MCS patients and even stronger decrease (pcorrected < 0.01) in VS patients compared to healthy controls. There was also a significant difference between MCS and VS patients (pcorrected < 0.05-0.01). Similar differences were observed in all three (alpha, beta1 and beta 2) frequency bands (Fig. 3). In the beta2 frequency band, while both MCS and VS patients demonstrated a decrease in the average strength of EEG operational synchrony compared to healthy volunteers, only the VS group showed a correspondingly significant decrease (pcorrected < 0.01) (Fig. 3). Additionally in VS patients this value dropped lower than the stochastic level of synchrony, which was estimated to be ±1 (see Fig. 2).
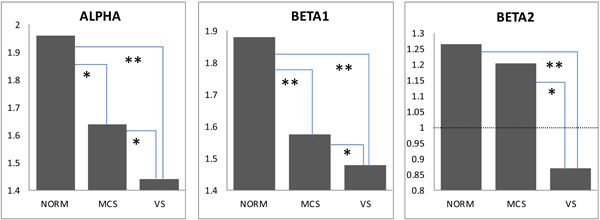
Default Mode Network EEG Operational Connectivity as a Function of self-Consciousness Loss. The strength of EEG operational connectivity decreases from healthy full self-conscious controls (NORM), to minimally conscious (MCS) patients and then to vegetative (VS) patients. Horizontal line on Beta2 graph indicates the upper stochastic level of connectivity. Below this threshold any connectivity is random. * – pcorrected < 0.05, ** – pcorrected < 0.01.
Detailed analysis revealed (pcorrected < 0.000001) that the anterior part of DMN was found to have the strongest decrease (48-66 %) in the EEG operational synchrony compared with the posterior areas (12-32 %) of the DMN (Table 1).
Decrease (in %) in Strength of EEG Operational Synchrony for Posterior and Anterior Parts of DMN
NORM-VS | ||
---|---|---|
Posterior | anterior | |
Alpha | 12 | 63 |
Beta1 | 14 | 48 |
Beta2 | 32 | 66 |
Pcorrected | ![]() |
Negative Values of EEG Operational Synchrony Strength within the DMN
We also have found a significant increase (pcorrected < 0.05-0.000001) in the number of negative EEG connections within the DMN from healthy controls (1.9-6.6 %) to MCS (3.7-8.3 %) and then to VS (8.2-12 %) patients in all three frequency bands (Table 2). Though the difference between NORM and MCS reached statistical values only post-hoc (p < 0.05). The MCS and VS patients differed significantly (pcorrected < 0.05). The largest number of negative EEG connections (6.6-12 %) was characteristic for the beta2 frequency band. As it has been described in the subsection “Second level of OA”, negative values of EEG operational synchrony mark EEG ‘active’ decoupling (see Fig. 2).
The Number (in %) of Negative Values of EEG Operational Synchrony within DMN
NORM | MCS | VS | |
---|---|---|---|
Alpha | 1.9 | 3.7 | 8.5 |
Beta1 | 5 | 5.3 | 8.2 |
Beta2 | 6.6 | 8.3 | 12 |
Pcorrected | ![]() |
DISCUSSION
Demographic Factors
Because there were no significant differences between the MCS and VS groups in terms of age and time post brain injury, distribution of TBI and non-TBI etiologies, left- and right-side lesions, and distribution of medicated vs non-medicated patients, all these factors could not be responsible for the differences in EEG parameters found between MCS and VS groups. The absence of significant difference in age between healthy (NORM) subjects and both (MCS and VS) patient groups could not affect the observed differences between healthy subjects and patients.
EEG DMN Operational Synchrony Strength During Self-Awareness Expression
Our results confirmed both hypotheses (see Introduction section). The first and most straightforwardly predicted finding was that EEG DMN operational synchrony in all three (alpha, beta1 and beta 2) frequency bands was highest in healthy fully self-conscious subjects, lowest or even absent in VS patients, and intermediate in MCS patients: VS < MCS ≤ NORM (Fig. 3). Considering that DMN has been shown to be involved in self-referential processing [12, 14-16, 20-22], these results suggest that the strength of EEG DMN operational synchrony could be a potential indicator of a patient’s expression of self-consciousness. It could differentiate VS patients who lacking self-consciousness from MCS patients who have partially preserved operational connectivity architecture of DMN. We may hypothesize here that such preserved integrity of DMN in MCS patients could in principle be capable of supporting a minimal expression of self.
Fully conscious, healthy controls displayed a particular, relatively high level of operationally integrated DMN EEG architecture (Fig. 3). It has been proposed that the neuronal processes which constitute DMN in healthy subjects are in a delicate metastable balance between local specialized processes and global integration [24]. Excess or lack of either process marks a deviation from this optimal state [36, 96, 100, 101]. Therefore, diminished or absent (like in the beta2 frequency band) operational synchrony4 within the DMN in patients with severe brain injuries suggests a disruption of this network, all the way down to complete absence of any self-awareness, as in the VS patients of this study (Fig. 3). A similar conclusion has been reached by Sarà et al. [104], who used EEG approximate entropy measure and found functional isolation and disconnection within the brain network in VS patients. At the same time such a disabling state could still be responsible for the experiences associated with cognitive operations executed without self- (and any other) consciousness; the so-called returning to a rigid stimulus-response behavior of lower animal species [105]. Considering the current understanding that only a particular dynamic balance of integrated and segregated processes within the brain networks is specific and sufficient to produce self- [24] and full-fledged consciousness [36, 39, 96, 41, 100, 101, 102], we could speculate further that the partially preserved EEG operational connectivity within DMN in VS5 may indicate a minimal level of synchrony that is already insufficient (in contrast to MCS) to support representational content, which has reference to self from the first-person perspective.
This supposition is in line with the results from EEG event-related potential [106-108] and fMRI/PET [109, 110] studies. Based on these research, and considering that experimentally unconscious stimuli could evoke local event-related potentials [111-114] or even unconscious perception of word meanings [115], it has been suggested that simple neuronal responses of VS patients (a) represent at most only isolated cerebral functional modes [116-118] and (b) are autonomic and unconscious [119-121]. This is in line with the OA framework, which claims that simple processing operations could be responsible only for simple functions and/or phenomenal features; and that they are the building blocks of more complex operations responsible for complex/abstract functions and/or phenomenal objects or thoughts [43] (see also [122] for a similar view).
Our second hypothesis was about the strongest decrease of EEG functional connectivity in frontal areas of the DMN in states characterized by a decreased expression of self-consciousness (MCS and VS). This hypothesis was indeed confirmed: the anterior part of DMN was found to have the strongest decrease in the EEG operational synchrony compared with the posterior areas of the DMN (Table 1) in MCS and VS. This second main finding complements previous results, thus showing the chief role of frontal areas in self-referential processing. The recruitment of frontal lobe areas has been described to be associated with (i) top-down attentional control [123], (ii) personal identity and past personal experiences [46], (iii) coordination of basic drives and of other influences on goal-directed behaviors [124], (iv) complete self-consciousness [45] and self-specific stimuli [11] and (v) preferential processing of ‘own-name’ stimuli [49]. Hence, it was suggested that the frontal module of DMN most likely provides a critical self-related context (experience of agency) for all human behaviors and activities [24] (see also [47]). This conclusion is also supported by the fact that the frontal areas are reciprocally connected with nearly all other cortical, subcortical, and brainstem structures [125], and thus, represent some kind of a hub which integrates motivational, emotional, sensory, motor, and mnemonic information [126], serving as the “observing self” to maintain any conscious state [11, 47, 127]. Frontal dysfunction usually leads to an impaired self, decreased will and energy, a tendency to engage in repetitive and stereotypical behavioral patterns, difficulty in response set, poor inhibitory control, abnormalities of affect and emotions, impulsivity, disinhibition and poor motivation [128-132]. At the same time, it has been shown repeatedly that for the full expression of self-consciousness, a parallel existence and interplay of frontal and parietal operationally synchronized spatio-temporal patterns (operational modules) within the same neuronal network are required [11, 127, 133-138].
The third finding was about negative values of the index of EEG operational synchrony within the DMN which increased from healthy subjects, to MCS patients and reached its maximum in VS patients: VS > MCS > NORM (Table 2). Within the framework of OA theory, these negative values reflect the process of active decoupling between operations executed by distant neuronal assemblies [2]. Such negative values of EEG operational synchrony were observed in situations when either conscious multimodal perception [139] or cognitive processing related to consciousness [140] failed. On the basis of these data, it is here suggested that the DMN of patients in VS, besides being operationally under-connected, was also characterized by the process of active uncoupling of operations performed by neuronal assemblies, whose synchrony within a unified network has been suggested to be important for maintaining self-awareness [12, 14-16, 20-22]. Therefore, we could speculate that the ability to consciously access and integrate representational content about one’s self could not be achieved in such VS patients. Conversely, the MCS patients had significantly less events of DMN uncoupling, thus leaving a possibility for self-referential processing to be expressed for the time and to the extent which are proportional to the degree of achieved DMN operational synchrony. Here we propose that unstable operational interactions within the DMN may underlie cognitive fluctuations observed in MCS patients. Since this synchrony is decreased in MCS patients compared to healthy fully self-conscious subjects, the expression of self-consciousness is also decreased in such MCS patients as it was assessed by the LCF scale (see Methods section). We further hypothesize that the emergence from MCS may then reflect a further recovery of the sufficient and dynamic integrity within DMN to support normal self-awareness and goal-directed behaviour.
The Functional Role of Alpha and Beta Frequency Bands in a Functionally Connected DMN
Our study has identified that operational integrity of DMN had nearly identical changes in alpha, beta1 and beta2 frequency bands (Fig. 3) as a function of the self-consciousness expression. Based on the previously established facts that among tested five EEG frequency bands (delta, theta, alpha, beta1 and beta 2) (a) only alpha, beta1 and beta2 rhythms have shown changes consistent with the analytical consciousness model [41] and (b) DMN has significant positive correlation only with alpha and beta rhythms [63-65], one needs to question what is the specific functional contribution of these alpha and beta frequency oscillations in DMN’s overall role. This question is specifically important because usually adjacent frequency bands within the same neuronal network are associated with different functional brain states and compete with one other [141, 142].
It has been shown that several rhythms can actually coexist in the same cortical area and/or interact among different cortical areas [143, 144] if their individual functions complement each other in the achieving the overall ‘macro-function’ of the integrated network [1, 2, 43, 89]. As we have observed in the subsection “EEG-Signal Data Preprocessing” of this paper, both alpha and beta frequency oscillations have functions important for the self-referential processing, which has been recently proposed to be the chief functional role of DMN [12, 14-16, 20-22]. According to a meta-analysis of imaging and psychological studies on the self [22] it is concluded that self-referential processing is at the bottom of what constitutes the experiential self. It is thought that such a ‘self’ is created using both ‘concrete physical’ and ‘abstract’ representations [145].
As a general rule, alpha frequency oscillations are responsible for the complex and abstract neural representations due to integration of neuronal effects with long delays and large variability in delays, which lead to the involvement of large brain areas [141]. On the contrary, beta frequency oscillations subserve more precise and spatially limited neural representations due to incorporation of synaptic events from closely located regions with short synaptic delays and limited variability [141]. These neurophysiological peculiarities of alpha and beta frequency oscillations are responsible for their specific functional roles (see “EEG-Signal Data Preprocessing”).
Therefore it could be suggested that dynamic operational synchrony of these three frequency bands (alpha, beta1 and beta2) within the same neuronal network is thus essential for expressing DMN’s putative role – allowing self-referential processing to take place so that reflective awareness become possible. Based on the literature data [69-71, 75, 76] we hypothesize that in such dynamically integrated network, alpha activity would determine a coordinate top-down first-person perspective for cortical traces of discrete semantic representations that are supported by beta oscillatory activity [41]. Further, we propose that such an integrated network (DMN) would persist as long as subjects are engaged in active self-conscious behaviour [24]. Any decrease of such dynamic integrity within the DMN would thus result in a condition, probably present during VS (and, to a least degree, in the MCS), where raw sensory stimuli, that originate from both the outside and within the organism, could not be integrated in the context of a first-person meaningful narrative.
CONCLUSIONS, SIGNIFICANCE AND LIMITATIONS
Concluding Discussion
The notion of self-consciousness is understood in terms of first-person awareness that one is in some mental state [25, 146]. This first-person awareness is widely accepted as an incredibly important part of normal human life [147]. It is assumed that the core of such experiential self is constituted by the self-referential processes [22]. Although there is limited fMRI evidence that the functional connectivity within the DMN may underlie a phenomenon referred to as self-awareness [50, 51, 148-150], none of the known studies have explicitly investigated neurophysiological functional interactions among brain areas that comprise the DMN as a function of self-consciousness expression. In order to address this question EEG operational synchrony analysis [1, 2] was conducted in non- and minimally communicative patients (in VS and MCS) to study the strength of operational synchrony within DMN as a function of self-consciousness expression (indexed by LCF scale).
In the present study we have found converging evidences (at least for the group level) for a breakdown of EEG operational connectivity within the DMN in non- and minimally communicative patients with severe brain injuries in proportion to the degree of expression of clinical self-consciousness measured by the LCF scale. More specifically, we demonstrated that the strength of EEG operational synchrony within DMN was smallest or even absent in patients in VS, intermediate in patients MCS and highest in healthy fully self-conscious subjects: VS < MCS ≤ NORM (Fig. 3). At the same time the process of prohibition of coupling of operations performed by neuronal assemblies that comprise the DMN was highest in VS patients, intermediate in MCS patients and minimal in healthy fully self-conscious subjects: VS > MCS ≥ NORM (Table 2). The strongest decrease in strength of EEG operational synchrony as a function of self-consciousness loss was in the frontal EEG operational module of the DMN, when compared with the DMN’s posterior modules (Table 1).
The observed breakdown in the ability of cortical areas to interact effectively within the DMN in MCS and especially in VS contrasts sharply with persistence of EEG operational synchrony in DMNs of normal subjects [24]. Therefore, we could conclude that it is intact coordinated activity within the DMN that is important for (a) enabling routine self-representational processes to be integrated within a coherent phenomenal self-model [146, 151] which is subjectively experienced as a self [22] and (b) protecting such self-model from disintegration. It could be further proposed that DMN persistent operational integrity may allow discrete moments of “minimal self” to be integrated so that the experience of one’s self-existence in the ‘present’ is continuously related to the ‘past’ and the ‘future’ [24]. When such integrity within DMN drops significantly as in the MCS or even below a critical level as in VS, the fading of self-consciousness until full its absence (VS is a clinical condition of complete unawareness of self) is observed.
The reported alterations within DMN occurred across alpha, beta1 and beta2 frequency oscillations. Considering functional roles of these frequency bands [69-71, 75, 76] and their global (abstract) and specific (motor-sensory) processing complementarity [41], we could conclude that MCS and especially VS, among other discussed aspects, include impairment in the efficient integration of these processes. This conclusion agrees with the view that integration of information processed in the cerebral cortex networks might depend on the interplay between various frequency bands [152-154].
Our findings on the EEG operational synchrony within the DMN in patients with disorders of consciousness extend previous fMRI studies on the DMN functional connectivity in patients with severe brain injuries [50, 51, 148-150] by presenting the results of an estimation of neuronal functional connectivity using the EEG procedure, which is a direct measure of electric current within masses of dendrites of neuronal cell assemblies [155]. Furthermore, EEG operational synchrony measure does not consider functional connectivity with respect to a single region only, as it is done in the seed voxel fMRI approaches, but gives rise to a whole, naturally interconnected network structure which emerges from inherent brain activity. To the best of our knowledge, the present study is the first presentation of DMN EEG functional interactions in non- and minimally communicative patients with severe brain injuries.
At the same time, and in contrast to some researchers, we do not tend to interpret data on the DMN functional connectivity in terms of a full-fledged consciousness6. Consciousness is a very complex phenomenon, which subsumes an enormous amount of dynamic phenomenal features, patterns, and full-fledged objects, thoughts or scenes immediately present to a subject [26]; and the self is only one of such ‘phenomenal objects’ among many other [146]. In this context it is obvious (theoretically and empirically) that very limited number of brain structures that constitute DMN could not support all multivariability and complexity of the global consciousness. In contrast, and as empirical studies suggest, the operationally integrated DMN could be a “neural signature” of self-referential processesonly, with a control function of the overall behavior from a first-person perspective [24]. As it has been shown previously, the concrete conscious cognitive operations (other than self-referential) are supported by specific and transient operational modules, which are responsible for the presentation of specific and transient conscious states (for the overview see [43]). Therefore we could speculate that DMN has an intermediary role between sensory (external) and cognitive (internal) processing operations by integrating them within a unified first-person perspective taking framework.
Clinical Significance
The importance of EEG resting state studies (that do not require active participation from the patient, which is particularly important in non- and minimally communicative patients with severe brain injuries [149]) and advantages of EEG screening in comparison to fMRI or PET studies from a clinical point of view has been discussed by us elsewhere [41]. Here we would like to stress briefly that after further validation of the results on the integrity of DMN we can expect to provide clinicians with an objective instrument for signsof (un)consciousness of self. We hope that such objective measure will help to reduce the currently very high level (37-43% [156-159]) of misdiagnosis of VS patients among patients with severe brain injuries.
Methodological Limitations
One limitation of this study is a relatively small experimental group sample (N = 21; 14 VS patients and 7 MCS patients). As we have discussed elsewhere [41] the main reason for this is the difficulty in finding non- and minimally communicative patients with severe brain injuries that would fulfill all inclusion criteria and have comparable brain lesions. At the same time, we state that to confirm the presented in this paper results, future studies that include a larger group of patients is warranted.
Second limitation concerns the cephalic EEG reference (mean of the signals from C3 and C4 electrodes). Because there is some possibility of distortion of the potentials’ topography with such reference montage [160], future studies need to be organized with the most widely used and reliable EEG reference montage (linked ears) to verify the presented in this paper results.
Considering the comment of Kotchoubey and Lang [80] that differences between chronically immobile, dependent patients with severe brain injuries and the healthy population are so great and diverse that any comparison between such groups would be meaningless, a healthy control group used in the presented study could represent the third potential limitation. However, as we discussed in detail elsewhere [41] there is sufficient evidence indicating that this putative limitation could be irrelevant. At the same time, we stress that to verify/confirm the results presented in this paper, future studies that include a control group of brain-damaged but fully conscious patients is warranted.
CONFLICT OF INTEREST
The authors confirm that this article content has no conflicts of interest.
NOTES
1 About additional advantages and some limitations of such experimental model see Footnote 1 in [41].
2 Indeed, as it has been stressed by Kotchoubey and Lang [80], “diagnostics of consciousness should be based on a foundation other than everyday intuition, and this can only be a sound theory of neural correlates of consciousness (NCC), or, lacking such a theory, at least a plausible theoretical approach”. For a similar view see Tononi [81] who stated that “it is important to complement clinical and experimental investigations with a theoretical approach aiming at understanding consciousness — what it is and how it can be generated — at a fundamental level (…)”.
3 In mathematical statistics this phenomenon is known as the change-point problem [90].
4 It has been suggested that functional disconnection of some elements from the system, while the elements by themselves could still be activated, would have virtually the same effect as the destruction or permanent inactivation of these elements [102]. This prediction was confirmed in computer simulations [103].
5 At the same time, as we have discussed in [24], this fact may point to a more fundamental or intrinsic property of minimal integrity of DMN. It can be responsible for the need of the organism (even being unconscious) to integrate the internal representations and/or operations within so-called “central-model” to make them available for further selective resource allocation and processing. In this sense, such basic central-model may be unconscious.
6 Data on the EEG operational architectonics that characterizes the full-fledged consciousness in the same cohort of patients are reported somewhere [41].
ACKNOWLEDGEMENTS
The authors thank Caterina Prestandrea (neurophysiology technician), who made all the EEG recordings and Carlos Neves (Computer Science specialist) for programming, technical, and IT support. Special thanks for English editing to Dmitry Skarin. This work was supported by BM-Science Centre, Finland. Authors declare no conflict of interests.