All published articles of this journal are available on ScienceDirect.
Functional MRI of Rehabilitation in Chronic Stroke Patients Using Novel MR-Compatible Hand Robots
Abstract
We monitored brain activation after chronic stroke by combining functional magnetic resonance imaging (fMRI) with a novel MR-compatible, hand-induced, robotic device (MR_CHIROD). We evaluated 60 fMRI datasets on a 3 T MR system from five right-handed patients with left-sided stroke ≥6 months prior and mild to moderate hemiparesis. Patients trained the paretic right hand at approximately 75% of maximum strength with an exercise ball for 1 hour/day, 3 days/week for 4 weeks. Multi-level fMRI data were acquired before, during training, upon completion of training, and after a non-training period using parallel imaging employing GeneRalized Autocalibrating Partially Parallel Acquisitions (GRAPPA) while the participant used the MR_CHIROD. Training increased the number of activated sensorimotor cortical voxels, indicating functional cortical plasticity in chronic stroke patients. The effect persisted four weeks after training completion, indicating the potential of rehabilitation in inducing cortical plasticity in chronic stroke patients.
INTRODUCTION
Stroke is one of the main causes of morbidity in modern society. Approximately 400 people per 100,000 over the age of 45 years have a first stroke each year in the United States and Europe. Patients who survive a stroke usually recover at least some of the functionality compromised by the stroke within three months, although only 25% return to the level of daily physical functioning seen in community-matched persons who have not suffered a stroke [1]. Understandably, patients’ level of functioning is positively associated with quality of life [2].
Recovery of function after stroke depends upon many factors, including resolution of edema and survival of the ischemic penumbra [3]. Post-stroke brain plasticity includes change of function in existing synapses, synaptogenesis, cortical reorganization, and probably neurogenesis, and all these changes are stimulated by activity [4]. While a clear causal link between cerebral reorganization and functional recovery has yet to be firmly established, brain imaging studies in chronic stroke patients have shown that plastic changes can occur, including enhanced bilateral activation of the sensorimotor cortex, increased activity in secondary or higher order sensorimotor areas, and recruitment of additional cortical areas during performance of a hand sensorimotor task [5].
Recent findings have revealed evidence of structural plasticity co-localized with areas exhibiting functional plasticity in the human brain after a stroke [6]. Accordingly, recovery of function after stroke is now widely considered to be a consequence of central nervous system (CNS) reorganization. Non-invasive, functional neuroimaging methods, such as functional magnetic resonance imaging (fMRI), positron emission tomography, transcranial magnetic stimulation, magnetoencephalography and electroencephalography, now enable serial studies of training-induced plasticity to be conducted in stroke patients [7]. Training-induced reorganization of the motor system has been consistently reported despite differences in technical and methodological approaches, leading to improvements in function that are commonly seen over weeks, months, and sometimes years after stroke [3, 8].
Meanwhile, recent technological advances have made it possible to use robotic devices to provide safe and intensive rehabilitation to people with mild to severe motor impairments following neurological injury. A robotic device is capable of controlling and quantifying the intensity of practice and objectively measuring changes in movement kinematics and force. In addition to providing new options for treatment, this technology promises to further our understanding of the mechanisms that underlie the recovery of motor function and neural reorganization after stroke. Several studies have demonstrated benefits of robot-assisted therapy for people undergoing neurological recovery [4, 9-18]. These studies revealed that robotic therapy produced significant gains in motor coordination and muscle strength of the exercised shoulder and elbow. Furthermore, these improvements were sustained during a three-year period following discharge from the hospital [9].
Here, we present data in support of online brain fMRI using a novel MR-compatible hand-induced robotic device (MR_CHIROD) to monitor rehabilitation after chronic stroke.
MATERIALS AND METHODOLOGY
Patients
Patients (≥6 months after stroke) who agreed to be contacted for stroke recovery and rehabilitation studies were recruited through registries of stroke survivors maintained at Massachusetts General Hospital. Briefly, the inclusion criteria were first-ever left-sided ischemic subcortical middle cerebral artery (MCA) stroke confirmed by computed tomography [10] or MRI ≥6 months prior; mild to moderate contralateral hemiparesis affecting the right hand; premorbid right hand dominance; ability to give written consent; and age 40–70 years. Exclusion criteria were defined by the NIH stroke scale questions (NIH_Stroke_Scale_Booklet.pdf, available at www.ninds.nih.gov/doctors/) for decreased level of consciousness (questions 1a, 1b, 1c; each >0), aphasia (question 9; score >2), and neglect (question 11; score >2). No patient had evidence of spasticity or joint stiffness while performing the motor task. Sensory modalities such as proprioception and ability to detect a pinprick and light touch were intact. No patient presented signs or history of somatosensory deficits of the right hand or other neurological or psychiatric disease, deafness and/or blindness, prior cerebrovascular disease, brainstem stroke, or multiple cerebral lesions.
Study Design
All participants served as their own controls, and trained at home using gel hand-exercise balls (www.bpp2.com/ physical_therapy_products/2932.html, cando gel hand exercise balls). Training consisted of squeezing one exercise ball from a set of 6 balls at approximately 75% of maximum strength with the paretic hand for 1 hour/day, 3 days/week during the training period. The appropriate hand exercise ball was selected based on measurement of each patient’s maximum hand-grip strength with a dynamometer. Serial MR neuroimaging exams were performed at baseline (before training), 4 weeks later, (halfway through the training period), another 4 weeks later (at the end of the training period), and again four weeks later after withdrawal from training to assess permanence of the effects. Each patient contributes information at each of 4 time points which makes for a powerful longitudinal random-effects model where the clustered data are modeled by a compound symmetry correlation structure [11]. MRI was performed using the MR neuroimaging protocol detailed below. Brain maps during training were compared to previous brain maps to detect any differences in plasticity. We evaluated 60 fMRI exams from five patients. All studies were approved by the Institutional Review Board at Massachusetts General Hospital and were performed at the Athinoula A. Martinos Center for Biomedical Imaging.
Use of the MR_CHIROD for Online MR Neuroimaging
The design and testing for the first generation MR_CHIROD was published previously [12-14] and the design of the second generation MR_CHIROD was recently published [15]. For this study, two designs were generated for the second-generation prototype, one with rotary brakes and the other with linear brakes. The linear brake version was chosen for fabrication because of its simplicity and lower cost [15]. The assembled MR_CHIROD is shown in [15] and in Fig. (1). The MR_CHIROD consists of three major subsystems: a) an electro-rheological fluid (ERF) resistive element, b) handles (one fixed and one sliding, Fig. 1, top panel), and c) two sensors, one optical encoder to measure patient-induced motion and one force sensor. Each subsystem includes several components of varying complexity. All components were optimally designed with strength and safety in mind for MR-compatibility and for regular and high-stress testing.
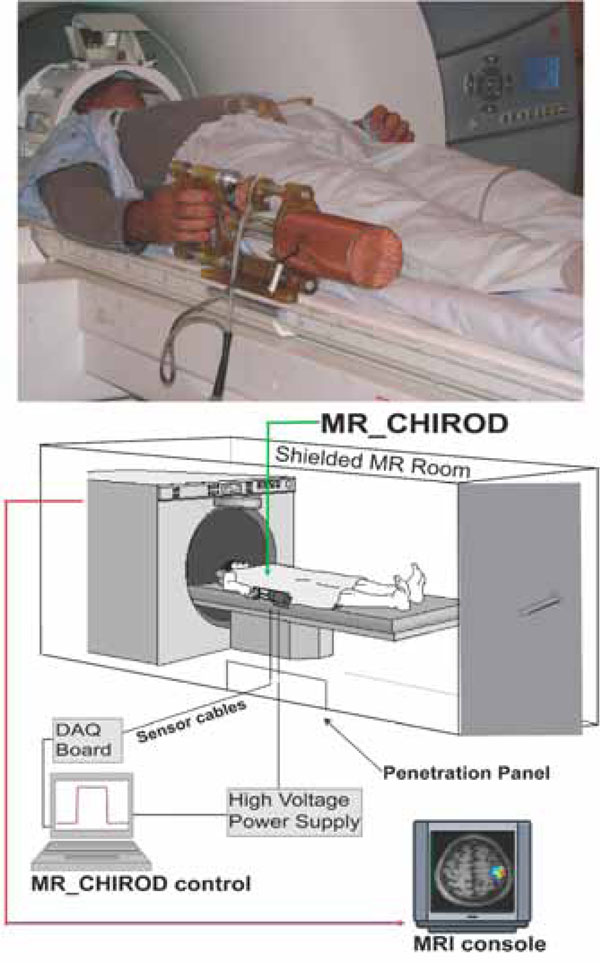
Arrangement of the MR compatible, hand-induced, robotic device (MR_CHIROD) in the MRI suite. A, top: MR_CHIROD used in the scanner; it is attached to the scanner while the subject, lying in the magnet operates it. B, bottom: Schematic of the MR_CHIROD set up in the MRI suite. The resistive element (ERF damper) consists of two electrodes and contains the ERF. The piston (piston shaft drawn) moves through the ERF with a controlled force of contraction provided by the voltage-controlled variable viscosity of the ERF fluid. A Faraday cage encloses the core of the device, allowing a necessary opening for the movable piston shaft. The negative electrode of the damper (connecting to the negative terminal of the power supply) and the Faraday cage are grounded to the penetration panel of the MR room. A low-pass filter is attached to the penetration panel. Sensor readings (force, position) are transmitted through the penetration panel through grounded DSub-9 connectors. The sensor wires are coaxially shielded and all are properly grounded to the penetration panel. The sensor readings are used for real-time, closed loop control of the ERF resistive element. The output from the control-loop regulates the voltage output of the power supply, in turn ensuring control of the ERF resistive element and the generated resistive force.
The MR_CHIROD was designed to provide 200 N of resistive force and to be controlled in real time [15]. It was empirically found that the maximum force of squeezing a dynamometer with fixed handles (Baseline® Hydraulic Hand Dynamometer, 200 lb, Best Priced Products, Inc.) is larger than the maximum force of dynamically squeezing the handles of the MR_CHIROD. Subsequently, maximum force of active squeezing was estimated using the MR_CHIROD itself. Specifically, the MR_CHIROD was set at high values of resistive force and subjects were asked to squeeze. Maximum force was defined as the force at which subjects could barely complete one to three strokes of the MR_CHIROD. Patients’ maximum force of active squeezing was 128 N ± 13 N (N = 5, male). For each patient, percent levels of applied force were calculated using the patient’s own maximum force as a reference (100% of effort).
The MR_CHIROD was configured to securely attach to the scanner table next to the participant, who thus feels no weight (Fig. 1, top panel). During testing, the MR_CHIROD power supply (Trek 609-C, ±4kV/20mA) that is activating the ERF fluid (Fludicon), and the DAQ unit (NI 6062-E) and laptop with control software (NI Labview), were located outside the RF-shielded MRI scanner room (Fig. 1, bottom panel). The cables connecting the power supply and the sensors to MR_CHIROD were of appropriate length and impedance and properly shielded.
MR Neuroimaging Examination Protocol
All studies were performed on a state-of-the-art 3 T MR system for increased signal-to-noise ratio (SNR). We used a systematic approach to optimize our protocol by varying the echo time, the repetition time, the GeneRalized Autocalibrating Partially Parallel Acquisitions (GRAPPA) acceleration factor, the field of view, FOV; and the number of excitations, NEX, to keep the protocol 30–45 min long. We used a 12-channel Siemens Tim coil and collected MR images using the following protocol (in the order indicated below).
Multilevel fMRI
A high-resolution GRAPPA (GeneRalized Autocalibrating Partially Parallel Acquisitions) EPI sequence was used for whole-brain BOLD fMRI at optimal spatial resolution for BOLD detection. Typical parameters: 96 x 96 matrix (2mm x 2mm in plane resolution), 50 slices/3mm slice thickness, GRAPPA factor 3, 85 auto-calibration lines, bandwidth/pixel = 1.3KHz/pixel 60 volumes (180 sec) were used in fMRI processing.
T1-Weighted MR Images
A high-resolution 3-dimensional T1-weighted, MP-RAGE (magnetization-prepared rapid gradient echo) image was acquired for anatomical reference and optimal gray-white matter contrast. (Sagittal orientation, flip angle = 7°, TE = 4.73 ms; TR = 2,530 ms; TI = 1,100 ms; slice thickness = 1.00 mm; matrix = 352 x 352 x 192).
Fluid Attenuation Inversion Recovery (FLAIR)
MR Images
A FLAIR image was acquired to provide anatomical localization of hyperintense regions and stroke lesions. Parameters: GRAPPA factor 2, 0.6 mm x 0.8 mm in plane resolution / 288 x 384 matrix, 5 mm slice thickness, TR/TE/TI = 10,000ms / 71ms / 2,500ms.
Motor Hand Paradigm
As the subject squeezed the device, we monitored the changing levels of force and compared precise measures of compression force with features of brain activation. Compression, because of its potential as a marker of motor function in stroke patients and because it can be performed by subjects with motor deficits [16], has been used in several clinical brain-mapping studies [17-20]. Our experimental paradigm consisted of alternating action and rest periods, of 30 s each (Fig. 2). During the action period, the subject compressed the robotic device and released continuously at a rate of 0.5 Hz. The palm of the hand rested on the stationary handle of the device and the four fingers on the moving handle (Fig. 1, top panel). The squeezing motion was performed by flexing the four fingers while the thumb was kept at a natural free-rest position and was neither flexed to form a fist nor used to push against the stationary handle. As was mentioned previously, all subjects selected were right-handed and used only the paretic hand. The fixed set up of the MR_CHIROD on the right side of the scanner table (Fig. 1) made the repetition of the motor task with the left, non-paretic, hand not feasible. The subjects’ squeezing rate was guided by a visual ‘metronome’ cue circle oscillating radially at a frequency of 0.5 Hz, projected on a neutral-background screen. A fixation cross was projected during the rest periods. The stimulus was implemented using the PsychoPhysics Toolbox (www.psychtoolbox.org/). Previous experience has shown that head movement increases at rates above 1.5 Hz, and movement below 1.5 Hz is generally associated with less fatigue, but also with decreased signal. A squeezing rate of 0.5 Hz was a well-justified compromise, especially because the study was performed at 3 T where a higher SNR is anticipated. It was also determined empirically that this rate allows all subjects to complete full squeezing strokes of the device at all force levels.
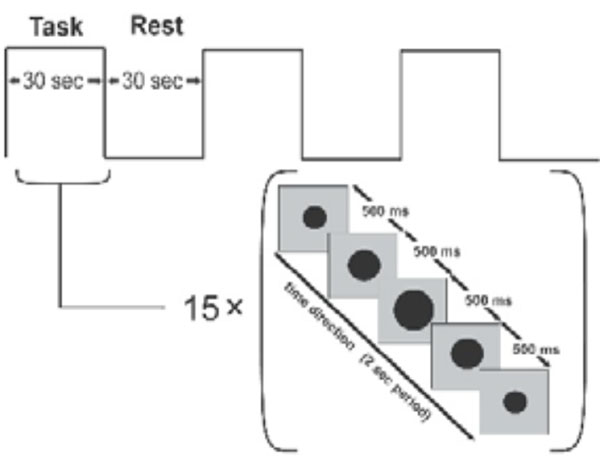
Block design for motor task. The subject squeezes in 30 sec blocks, following a squeezing cue that lasts 2 s and is presented 15 times. The black dot oscillates to cue the rhythm of squeezing. A stationary fixation cross is presented during the idle rest period.
The paradigm lasted 180 s, consisting of 3 action and 3 rest periods. Each volunteer performed the paradigm using 3 different combinations of the resistive force of the robotic device. Specifically, we applied a resistive force equal to 45%, 60%, and 75% of the maximum grip strength and volunteers rested between sessions to minimize fatigue. To ensure that all experimental subjects performed the required motor task, we assigned the larger force of the paradigm to be 75% of an individual subject’s maximum force. According to our experience, since patients were requested to perform using only a portion of their respective maximum force, they were able to perform the task. The percent levels compensate for performance confounds by constraining between-subjects performance to be approximately the same [21]. Training typically took subjects 15 min ± 7 min and was performed before scanning. Patients performed the paradigm at each force level with the paretic hand. We restricted motion artifacts by placing foam rubber pads and straps across the forehead and the arms. Subjects’ arms were kept resting at their sides and extra foam padding was used at the elbow to dampen vibratory couplings between the magnet bore wall and the volunteer’s arm as well as between the volunteer’s arm and his body. The pads also served to minimize elbow flexion and additional reflexive motion, and to minimize translational and rotational head motion (typical translational head motion is well under 1 mm, typically ranging from 0.1 to 0.4 mm for most subjects). Care was taken to restrain inadvertent movement or squeezing of the non-task-performing hand.
FMRI Data Analysis
A standard processing stream using the Statistical Parametric Mapping (SPM2, www.fil.ion.ucl.ac.uk/spm) was used for single-subject analysis. Images were aligned and normalized to MNI152 space. Additionally, a mask of each patient’s stroke lesion was drawn and incorporated into the normalization step for that patient [22]. Images were then smoothed with a 4x(voxel dimensions) anisotropic Gaussian kernel. The 4x kernel was chosen as an optimal choice between maximizing sensitivity through use of large smoothing kernels [23] and retaining ample spatial specificity of the original functional images. A temporal Gaussian filter (4 sec full-width half-maximum) was applied to account for temporal auto-correlations in the time series. It is important to note that blood oxygen level dependent (BOLD) fMRI dependency on physiological noise decreases with increasing spatial resolution, in that thermal noise accounts for a larger proportion of the total variance [24]. Our protocol used voxels with size of 12 mm3 rather than voxels approximately sized 50 mm3, such as resulting from a typical 3 mm x 3 mm x 5 mm EPI resolution for fMRI. It is also important to note that although temporal SNR (signal to noise ratio) increases with spatial smoothing [25], very broad averaging will “wash out” finer features. Voxel-wise activation threshold was set at P < 0.05, corrected for multiple comparisons.
Further information from the MR_CHIROD was used in the general linear model (GLM) fit to the fMRI timeseries. The MR_CHIROD provides the exact force trace as a function of time with typical sampling resolution ranging from 50 Hz to 100 Hz. The exact onset times and durations of the task blocks were measured from the MR_CHIROD force traces, thus accounting for possible timing errors on the part of the subject and resulting in a better GLM fit.
In this study, bilateral areas of interest were: the sensorimotor cortex (SMC), defined as the combination of the primary motor (M1) and primary sensory (S1) cortices; M1 alone; S1 alone; premotor cortex [26]; supplementary motor area (SMA); and the cerebellum. SMA was defined as the medial wall of the hemisphere from the top of the brain to the depth of the cingulate sulcus with the posterior boundary halfway between the extension of the central and precentral sulci onto the medial surface and the anterior boundary at the vertical line through the anterior commissure [27]. The SMC extended from the precentral to the postcentral gyrus and from the brain vertex to the Sylvian fissure. Areas of interest were selected functionally from the fMRI activation patterns and the location of activation in stroke patients was checked in normalized space using the Wake Forest University (WFU) Pickatlas tool [28, 29] and the Montreal Neurological Institure (MNI) Space Utility (MSU; www.fil.ion.ucl.ac.uk/spm/ext/) in SPM. Interpreting fMRI results based solely on voxel-level significance maps may result in variability [30], which may be an artifact of arbitrary statistical thresholds and thus misleading [31]. Even the 2% most significant voxels have been found to vary considerably across runs, subjects, and analysis techniques [32]. In contrast, reproducibility of fMRI activations at a regional level has been found to be acceptable across sites, subjects, and techniques [33]. In this work, statistically significant voxels (P < 0.05 corrected) were further selected using a percent BOLD signal change with 2.0% set as the threshold [34]. While the numerical value of 2.0% is arbitrary in itself, only cortical motor areas are consistently activated at these values of percent BOLD. The 2.0% threshold was thus used as a filter, helping to select regions of interest without enforcing its boundaries apriori.
Statistical Analysis
Normality of variances in activated voxels was tested using the Shapiro-Wilks test in R (version 2.5.1), (P = 0.94). All comparisons between maximum effort levels during different stages in training were done using independent-samples t-test (two-tailed); a P-value below 0.05 was considered significant.
RESULTS
Stroke patients training at home with exercise gel balls underwent online brain mapping with fMRI using our second generation MR_CHIROD prototype.
Fig. (3) shows the results for a representative patient who underwent online mapping at three different sub-maximal levels of squeezing force (45%, 60% and 75%), halfway through training and at the end of training. The fMRI results suggest that there is an incremental increase in SMC activation with increasing force of squeezing. Completion of training further reinforced the incremental SMC activation at different submaximal levels of squeezing force. Moreover, there was recruitment of SMA activation at higher squeezing forces. The SMA recruitment was more pronounced after training. Similar activation patterns were observed in cerebellar areas (results not shown).
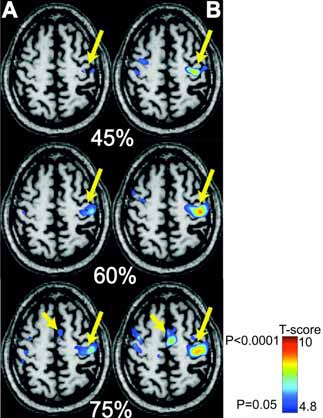
fMRI with MR_CHIROD in a 63-year old chronic stroke patients, four years after his MCA stroke. A, left: Patient performing the motor paradigm halfway through training. B, right: Patient performance after training. The patient squeezed the MR_CHIROD at 45%, 60%, and 75% of maximum grip force. Activation threshold P < 0.05, corrected; activation maps are superimposed on patient T1-weighted anatomical image. SMC activation is the larger arrow; SMA activation is the smaller arrow.
Further analysis was performed in the pattern of SMC activation. Fig. (4) summarizes the results from the five patients at the three different performance levels and at four different time points (baseline, halfway through training, end of training and at follow up 4 weeks after training completion). Completion of training resulted in higher number of activated voxels compared to baseline or halfway through training, at any of the three submaximal performance levels. For example, 60% force of squeezing, at the completion of training resulted in 83.25 ± 5.45% activated voxels, compared with 48.74 ± 2.53% at baseline (P < 0.0001). Follow up 4 weeks after training completion showed 74.94 ± 10.71% SMC activation at 60% force of squeezing, which was significantly higher compared to baseline (P < 0.05). A similar trend was observed in the follow up group at 75% force of squeezing, though the results did not reach statistical significance compared with the baseline group. These results suggest that the increased SMC activation partially persists 4 weeks after training. Finally, Fig. (4) again shows that increased force of squeezing results in progressively greater number of voxel activation both at baseline, halfway through training, at training completion and at follow up.
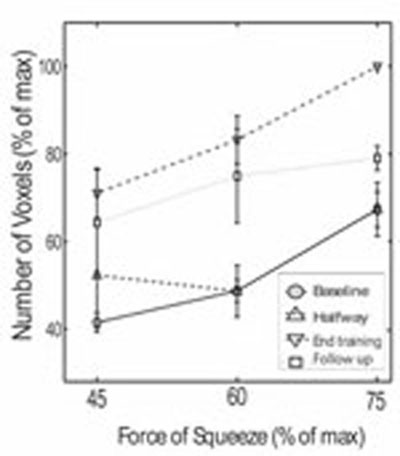
Number of activated voxels in the left (contralateral) SMC as a function of squeezing force in 5 chronic stroke patients (60 fMRI datasets). Online SMC mapping was performed during four different time points: At baseline; halfway through training; at the end of the training and 4 weeks follow-up after training.
DISCUSSION
The present study demonstrates that online fMRI using a novel hand device, MR_CHIROD, can monitor the functional cortical plasticity in chronic stroke patients undergoing late-onset rehabilitation. The study introduces a novel method of monitoring functional cortical plasticity and confirms prior studies suggesting recovery of function after stroke as a consequence of central nervous system reorganization. This method may be used to map functionally relevant adaptive changes in the human brain following CNS damage. It can thus lead to a greater understanding of how these changes are related to the recovery process, facilitate the development of novel therapeutic techniques designed to minimize CNS impairment, and assist in targeting these treatments to individual patients.
Due to our ability to control the resistivity and operability of ERF-controlled devices [12-15], MR_CHIROD may provide more sensitive, specific, and accurate information about the effectiveness of rehabilitation therapy beyond traditional paradigms, although further assessment is needed. The MR-compatibility of the MR_CHIROD allows online brain function monitoring which is an essential aspect of monitoring neurological disabilities associated with stroke recovery as well as recovery after brain tumor surgery, and monitoring of other CNS disorders including neurodegenerative disorders (e.g., Parkinson’s). The MR_CHIROD can also be used for monitoring muscle enhancement and augmentation. The novelty of our device relies on its unconventional type of actuation, via electro rheological fluids (ERF), which change their viscosity in response to varying electric fields. Unlike previously described devices [35, 36], this is the first ERF-based device that has been demonstrated to function in conjunction with fMRI for online brain mapping in chronic stroke patients.
Our parallel MRI (pMRI) approach to neuroimaging using GRAPPA [37-39] is novel as well. This method is not hindered by artifacts and is advantageous at high magnetic fields [40-43]. We did not observe a decreased BOLD signal as reported by Lütcke et al. [44] and we believe that our use of phased array coils increased spatial and/or temporal resolution further [45-47].
The first principal finding of this study is that motor training in chronic stroke patients results in increased SMC activation. Moreover, there is at least partial persistence of the increase SMC activation four weeks after the completion of the training. The second principal finding of our study is that even in chronic stroke patients, increased squeezing force is associated with increased contralateral SMC and SMA activation. A relationship between force of squeezing and motor cortex activation has been demonstrated previously in healthy volunteers [34, 48]. Observation of a similar activation pattern in stroke patients as evidenced by recruitment of the SMA suggests that cortical plasticity occurs even in areas affected by stroke, such as the SMC. Our observations may be explained by modulation of the relationship between brain activity and peak grip force by a preserved corticospinal system following a subcortical stroke [49].
Our data are consistent with the fundamental function of M1 in controlling voluntary movement based on a distributed network rather than discrete representations as originally proposed by Penfield & Rasmussen [50]. Furthermore, evidence of preserved capacity for plasticity in patients with chronic stroke indicates that these networks are capable of modification. Indeed, data in humans and corroborative studies in animals based on neuronal recordings and non-invasive neuroimaging methods have shown considerable plasticity of M1 representations and cell properties following pathological or traumatic changes in relation to everyday experiences, including motor skill learning and cognitive motor performance [51].
The persistence of the training-induced functional cortical plasticity is remarkable given that training is generally effective only when post-stroke rehabilitation is begun expeditiously with good motivation, sufficient intensity and frequency, and is maintained over a long period of time [4]. Here, we have shown that even in chronic stroke cases, functional cortical plasticity is possible. This finding supports previous reports [52, 53]. In particular, the latter study subjected chronic stroke patients to goal-directed robotic therapy and assessed them with traditional motor evaluation tests [53]. The study showed sustained improvement in motor abilities four months after discharge. This functional cortical plasticity is probably due to plastic functional reorganization of M1 in adult mammals, which apparently results from broad connections and the capacity for activity-driven changes in synaptic strength [51].
From a neuroscientific perspective, the study of cortical plasticity or recovery associated with training faces some fundamental problems because changes in plasticity naturally occur as the patient recovers from stroke. This makes it difficult to tease apart cortical activity changes that are due to spontaneous or training-induced plasticity. It is generally believed that the process of spontaneous recovery following stroke is due, in part, to changes in cortical plasticity, although the naturally occurring processes of motor recovery and exercise-induced changes in brain plasticity may overlap. From the perspective of this study, however, the issue of spontaneous recovery is largely removed as a concern because of our focus on subjects who are at least six months post-stroke and whose residual motor deficits are stable. In these subjects, therefore, any improvement in motor performance is more likely attributable to exercise-induced changes in cortical plasticity rather than to spontaneous recovery.
In the future, we plan to include a longitudinal study of a control group (chronic stroke patients without rehabilitation). However, this is not without its own pitfalls since the control group will have in principle different baseline and longitudinal trajectories. Instead, comparing the patients to their own baseline is a powerful longitudinal random-effects model since each patient contributes information at each of 4 time points and the clustered data is modeled by a compound symmetry correlation structure [11].
CONCLUSION
Stroke patient rehabilitation can be induced by motor training, resulting in functional cortical plasticity. Online brain fMRI using novel hand devices provides accurate monitoring and can be used in rehabilitation. This is clinically relevant since it will allow caregivers to select the most appropriate rehabilitation approach and to fine-tune it based on a brain maps obtained before and after a short trial of therapy.
ACKNOWLEDGEMENTS
This work was supported in part by a grant from the National Institute of Biomedical Imaging and Bioengineering of the National Institutes of Health (Grant number R21 EB004665-01A2) to A. Aria Tzika. We thank Dr. Mavroidis, the principal investigator of the subcontract to Northeastern University to build the MR_CHIROD. Finally we thank Zenab Amin Ph.D. and Ann Power Smith Ph.D. of Write Science Right for editorial assistance.